Post Syndicated from Matthew Green original https://blog.rapid7.com/2024/02/29/how-to-hunt-for-uefi-malware-using-velociraptor/
UEFI threats have historically been limited in number and mostly implemented by nation state actors as stealthy persistence. However, the recent proliferation of Black Lotus on the dark web, Trickbot enumeration module (late 2022), and Glupteba (November 2023) indicates that this historical trend may be changing.
With this context, it is becoming important for security practitioners to understand visibility and collection capabilities for UEFI threats. This post covers some of these areas and presents several recent Velociraptor artifacts that can be used in the field. Rapid7 has also released a white paper providing detailed information about how UEFI malware works and some of the most common types.
Background
Unified Extensible Firmware Interface, or UEFI, is the interface between a system’s hardware and its operating system (OS). The technology can be viewed as an updated BIOS capability to improve and add security to the boot process.
The two main types of UEFI persistence are:
- Serial Peripheral Interface (SPI) based
- Firmware payload implant that is resilient to even a hard disk format.
- Difficult to implement — there are risks associated with implementing and potentially bricking a machine if there are mistakes with the firmware.
- Difficult to detect at scale — defenders need to extract firmware which typically requires a signed driver, then running tools for analysis.
- Typically an analyst would dump firmware, then extract variables and other interesting files like PEs for deep dive analysis.
2. EFI System Partition (ESP) based
- A special FAT partition that stores bootloaders and sits late in the EFI boot process.
- Much easier to implement, only requiring root privileges and to bypass Secure Boot.
- Does not survive a machine format.
EFI Secure Variables API visibility
EFI Secure Variables (or otherwise known as NVRAM) is how the system distributes components from the firmware during boot. From an analysis point of view, whilst dumping the firmware is difficult needing manual workflow, all operating systems provide some visibility from user space. This blog will discuss the Windows API; however, for reference Linux and macOS provides similar data.
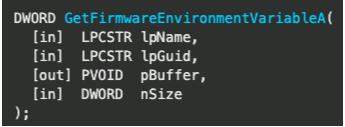
GetFirmwareEnvironmentVariable (Windows) can collect the name, namespace guid and value of EFI secure variables. This collection can be used to check current state including key/signature database and revocation.
Some of the data points it enables extracting are:
- Platform Key (PK) — top level key.
- Key Exchange Key (KEK) — used to sign Signatures Database and Forbidden Signatures Database updates.
- Signature database (db) — contains keys and/or hashes of allowed EFI binaries.
- Forbidden signatures database (dbx) — contains keys and/or hashes of denylisted EFI binaries.
- Other boot configuration settings.
It’s worth noting that this technique is relying on the Windows API and could be subverted with capable malware, but the visibility can provide leads for an analyst around boot configuration or signatures. There are also “boot only” NVRAM variables that can not be accessed outside boot, so a manual chip dump would need to be collected.
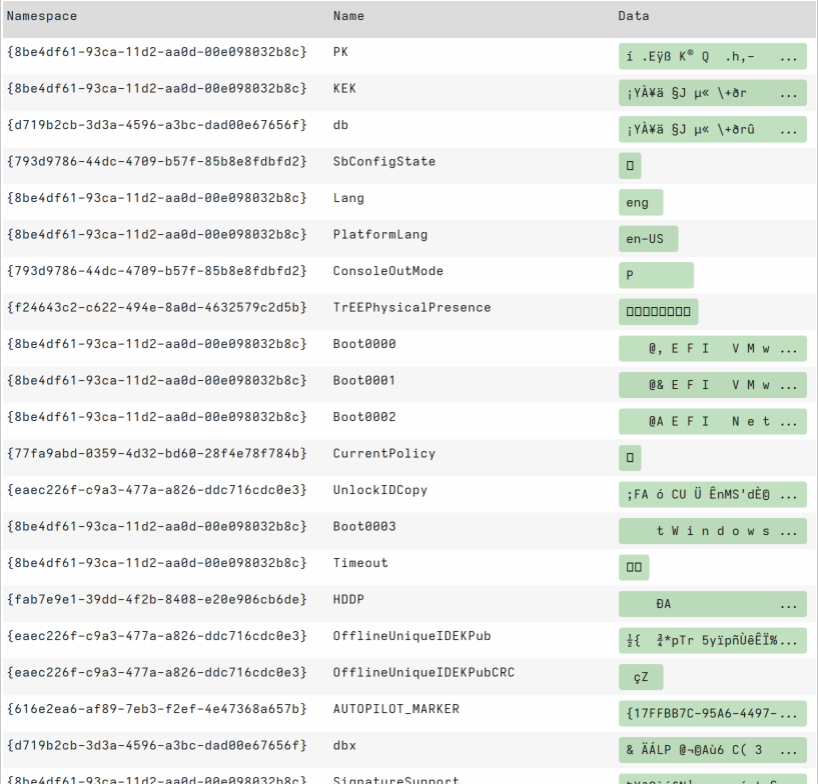
Velociraptor has a community contributed capability: Generic.System.EfiSignatures. This artifact collects EFI Signature information from the client to check for unknown certificates and revoked hashes. This is a great artifact for data stacking across machines and is built by parsing data values from the efivariables() plugin.
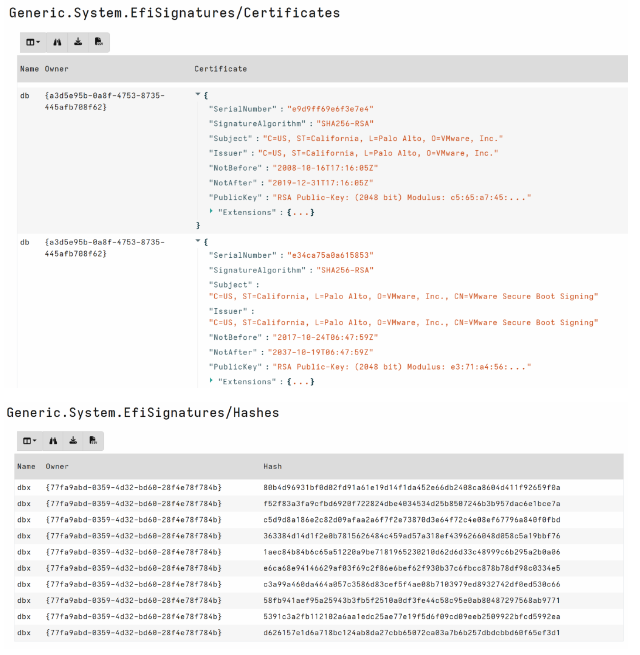
EFI System Partition (ESP) visibility
The ESP is a FAT partitioned file system that contains boot loaders and other critical files used during the boot process which do not change regularly. As such, it can be a relatively simple task to find abnormalities using forensics.
For example, parsing the File Allocation Table we can review metadata around path, timestamps, and deleted status that may provide leads for analysis.
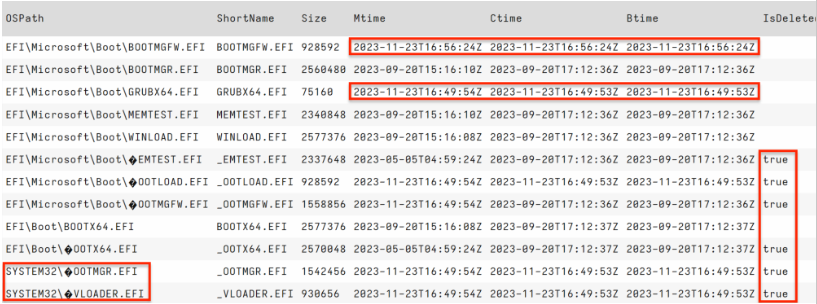
In the screenshot above we observe several EFI bootloader files with timestamps out of alignment. We would typically expect these files to have the same timestamps around operating system install. We can also observe deleted files and the existence of a System32 folder in the temporal range of these entries.
The EFI/ folder should be the only folder in the ESP root so querying for any paths that do not begin with EFI/ is a great hunt that detects our lead above. You can see in my screenshot below, the BlackLotus staging being bubbled to the top adding filtering for this use case.
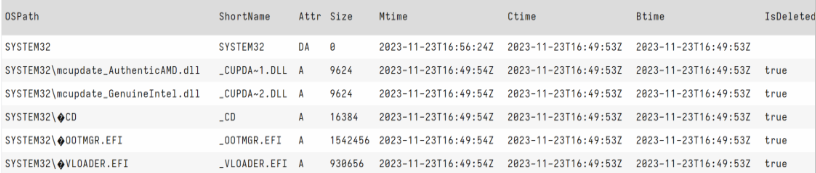
Interestingly, BlackLotus was known to use the Baton Drop exploit so we can compare to the publicly available Baton Drop and observe similarities to deleted files on the ESP.
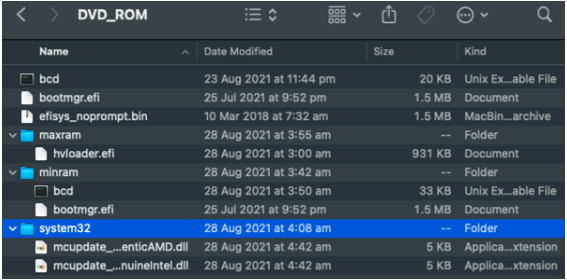
The final component of ESP-based visibility is checking the bytes of file contents. We can run YARA to look for known malware traits, or obtain additional file type metadata that can provide leads for analysis. The screenshot below highlights the well known Black Lotus certificate information and PE header timestamp.
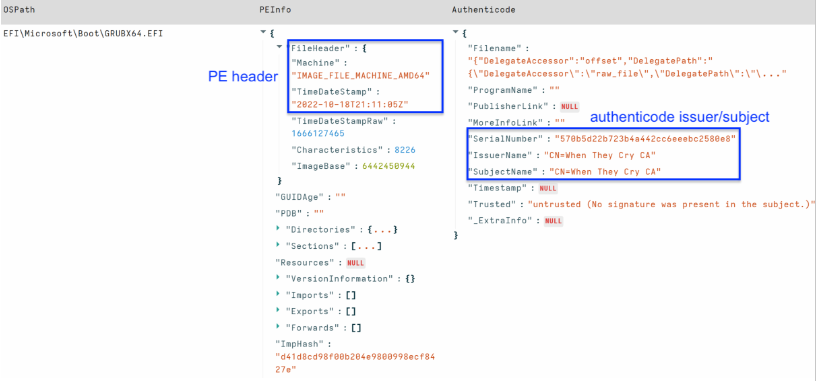

Available Velociraptor artifacts for this visibility of the ESP are:
- Windows.Forensics.UEFI — This artifact enables disk analysis over an EFI System Partition (ESP). The artifact queries the specified physical disk, parses the partition table to target the ESP File Allocation Table (FAT). The artifact returns file information, and PE enrichment as typical EFI files are in the PE format.
- Windows.Detection.Yara.UEFI — This artifact expands on basic enumeration of the ESP and enables running yara over the EFI system partition.
Measured Boot log visibility
Bootkit security has always been a “race to the bottom.” If the malware could load prior to security tools, a defender would need to assume they may be defeated. Since Windows 8, Measured Boot is a feature implemented to help protect machines from early boot malware. Measured Boot checks each startup component — from firmware to boot drivers — and stores this information in the Trusted Platform Module (TPM). A binary log is then made available to verify the boot state of the machine. The default Measured Boot log location is C:\Windows\Logs\MeasuredBoot\*.log and a new file is recorded for each boot.
Windows.Forensics.UEFI.BootApplication parses Windows MeasuredBoot TCGLogs to extract PathName of events, which can assist detection of potential ESP based persistence (EV_EFI_Boot_Services_Application). The artifact leverages Velociraptor tools to deploy and execute Matt Graeber’s excellent powershell module TCGLogTools to parse TCGLogs on disk and memory.
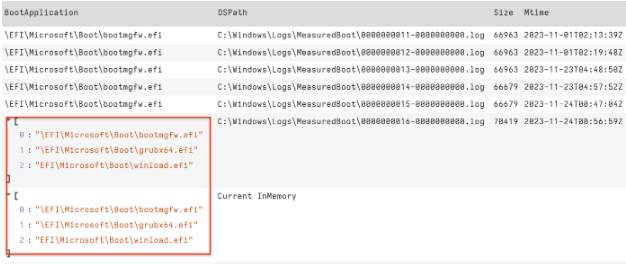
We can see when running on an infected machine that the BOOT application path has clearly changed from the default: \EFI\Microsoft\Boot\bootmgfw.efi. Therefore, Boot Application is a field that is stackable across the network.
We can also output extended values, including digest hashes for verification.
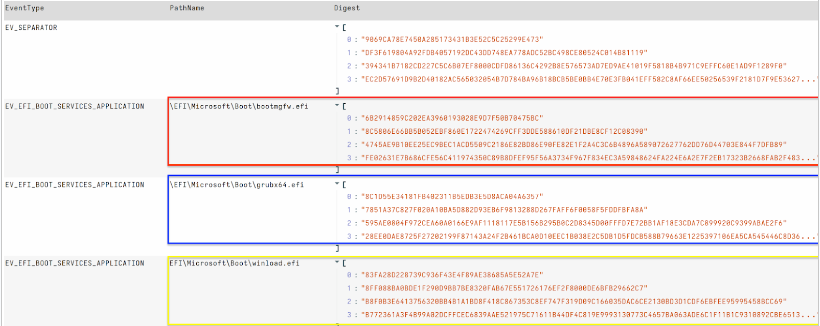
Other forensic artifacts
There are many other generic forensic artifacts analysts could focus on for assisting detection of a UEFI threat. From malware network activity to unexpected errors in the event log associated with Antivirus/Security tools on the machine.
For example: BlackLotus made an effort to evade detection by changing Windows Defender access tokens to SE_PRIVILEGE_REMOVED. This technique keeps the Defender service running but effectively disables it. While Velociraptor may not have protected process privileges to check tokens directly, we can check for other indicators such as errors associated with use.
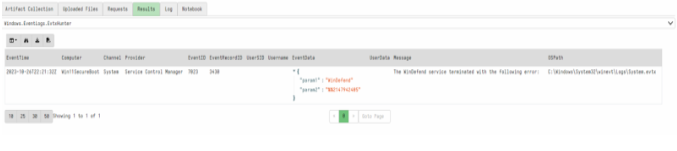
Similarly, Memory integrity (HVCI) is a feature of virtualization-based security (VBS) in Windows. It provides a stronger virtualization environment via isolation and kernel memory allocations.The feature is related to Secure Boot and can be disabled for malware that needs a lower integrity environment to run. It requires setting the configuration registry key value to 0.
HKLM\SYSTEM\CurrentControlSet\Control\DeviceGuard\Scenarios\HypervisorEnforcedCodeIntegrity\Value
0 – disabled
1 – enabled
Windows.Registry.HVCI available on the artifact exchange can be used to query for this key value.

Conclusion
Despite UEFI threats possessing intimidating capabilities, security practitioners can deploy some visibility with current tools for remote investigation. Forensically parsing disk and not relying on the Windows API, or reviewing other systemic indicators that may signal compromise, is a practical way to detect components of these threats. Knowing collection capabilities, the gaps, and how to mitigate these is just as important as knowing the threat.
In this post we have covered some of Velociraptor’s visibility for UEFI threats and we have only scratched the surface for those who know their environment and can query it effectively. Rapid7 supports Velociraptor open source, providing the community with Velociraptor and open source features unavailable even in some paid tools.
References:
- ESET, Martin Smolar – BlackLotus UEFI bootkit: Myth confirmed
- Microsoft Incident Response – Guidance for investigating attacks using CVE-2022-21894: The BlackLotus campaign
- Trellix Insights: TrickBot offers new TrickBoot
- Palo Alto Unit 42: Diving Into Glupteba’s UEFI Bootkit
- Sentinel1: Moving from common sense knowledge about uefi to actually dumping uefi firmware