Post Syndicated from Brendan Jenkins original https://aws.amazon.com/blogs/security/accelerate-security-automation-using-amazon-codewhisperer/
In an ever-changing security landscape, teams must be able to quickly remediate security risks. Many organizations look for ways to automate the remediation of security findings that are currently handled manually. Amazon CodeWhisperer is an artificial intelligence (AI) coding companion that generates real-time, single-line or full-function code suggestions in your integrated development environment (IDE) to help you quickly build software. By using CodeWhisperer, security teams can expedite the process of writing security automation scripts for various types of findings that are aggregated in AWS Security Hub, a cloud security posture management (CSPM) service.
In this post, we present some of the current challenges with security automation and walk you through how to use CodeWhisperer, together with Amazon EventBridge and AWS Lambda, to automate the remediation of Security Hub findings. Before reading further, please read the AWS Responsible AI Policy.
Current challenges with security automation
Many approaches to security automation, including Lambda and AWS Systems Manager Automation, require software development skills. Furthermore, the process of manually writing code for remediation can be a time-consuming process for security professionals. To help overcome these challenges, CodeWhisperer serves as a force multiplier for qualified security professionals with development experience to quickly and effectively generate code to help remediate security findings.
Security professionals should still cultivate software development skills to implement robust solutions. Engineers should thoroughly review and validate any generated code, as manual oversight remains critical for security.
Solution overview
Figure 1 shows how the findings that Security Hub produces are ingested by EventBridge, which then invokes Lambda functions for processing. The Lambda code is generated with the help of CodeWhisperer.
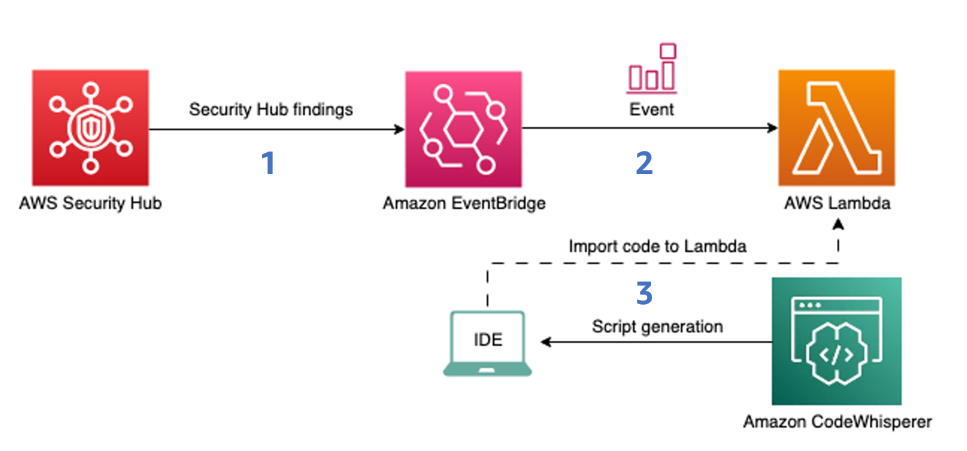
Figure 1: Diagram of the solution
Security Hub integrates with EventBridge so you can automatically process findings with other services such as Lambda. To begin remediating the findings automatically, you can configure rules to determine where to send findings. This solution will do the following:
- Ingest an Amazon Security Hub finding into EventBridge.
- Use an EventBridge rule to invoke a Lambda function for processing.
- Use CodeWhisperer to generate the Lambda function code.
It is important to note that there are two types of automation for Security Hub finding remediation:
- Partial automation, which is initiated when a human worker selects the Security Hub findings manually and applies the automated remediation workflow to the selected findings.
- End-to-end automation, which means that when a finding is generated within Security Hub, this initiates an automated workflow to immediately remediate without human intervention.
Important: When you use end-to-end automation, we highly recommend that you thoroughly test the efficiency and impact of the workflow in a non-production environment first before moving forward with implementation in a production environment.
Prerequisites
To follow along with this walkthrough, make sure that you have the following prerequisites in place:
- An AWS account
- Visual Studio Code (VS Code) or supported JetBrains IDEs
- Python
- CodeWhisperer enabled locally in your IDE
- AWS Config enabled
- Security Hub enabled, with the NIST Special Publication 800-53 Revision 5 standard selected
Implement security automation
In this scenario, you have been tasked with making sure that versioning is enabled across all Amazon Simple Storage Service (Amazon S3) buckets in your AWS account. Additionally, you want to do this in a way that is programmatic and automated so that it can be reused in different AWS accounts in the future.
To do this, you will perform the following steps:
- Generate the remediation script with CodeWhisperer
- Create the Lambda function
- Integrate the Lambda function with Security Hub by using EventBridge
- Create a custom action in Security Hub
- Create an EventBridge rule to target the Lambda function
- Run the remediation
Generate a remediation script with CodeWhisperer
The first step is to use VS Code to create a script so that CodeWhisperer generates the code for your Lambda function in Python. You will use this Lambda function to remediate the Security Hub findings generated by the [S3.14] S3 buckets should use versioning control.
Note: The underlying model of CodeWhisperer is powered by generative AI, and the output of CodeWhisperer is nondeterministic. As such, the code recommended by the service can vary by user. By modifying the initial code comment to prompt CodeWhisperer for a response, customers can change the corresponding output to help meet their needs. Customers should subject all code generated by CodeWhisperer to typical testing and review protocols to verify that it is free of errors and is in line with applicable organizational security policies. To learn about best practices on prompt engineering with CodeWhisperer, see this AWS blog post.
To generate the remediation script
- Open a new VS Code window, and then open or create a new folder for your file to reside in.
- Create a Python file called cw-blog-remediation.py as shown in Figure 2.
Figure 2: New VS Code file created called cw-blog-remediation.py
- Add the following imports to the Python file.
- Because you have the context added to your file, you can now prompt CodeWhisperer by using a natural language comment. In your file, below the import statements, enter the following comment and then press Enter.
- Accept the first recommendation that CodeWhisperer provides by pressing Tab to use the Lambda function handler, as shown in Figure 3.
&ngsp;Figure 3: Generation of Lambda handler
- To get the recommendation for the function from CodeWhisperer, press Enter. Make sure that the recommendation you receive looks similar to the following. CodeWhisperer is nondeterministic, so its recommendations can vary.
- Take a moment to review the user actions and keyboard shortcut keys. Press Tab to accept the recommendation.
- You can change the function body to fit your use case. To get the Amazon Resource Name (ARN) of the S3 bucket from the EventBridge event, replace the bucket variable with the following line:
- To prompt CodeWhisperer to extract the bucket name from the bucket ARN, use the following comment:
Your function code should look similar to the following:
- Create a .zip file for cw-blog-remediation.py. Find the file in your local file manager, right-click the file, and select compress/zip. You will use this .zip file in the next section of the post.
Create the Lambda function
The next step is to use the automation script that you generated to create the Lambda function that will enable versioning on applicable S3 buckets.
To create the Lambda function
- Open the AWS Lambda console.
- In the left navigation pane, choose Functions, and then choose Create function.
- Select Author from Scratch and provide the following configurations for the function:
- For Function name, select sec_remediation_function.
- For Runtime, select Python 3.12.
- For Architecture, select x86_64.
- For Permissions, select Create a new role with basic Lambda permissions.
- Choose Create function.
- To upload your local code to Lambda, select Upload from and then .zip file, and then upload the file that you zipped.
- Verify that you created the Lambda function successfully. In the Code source section of Lambda, you should see the code from the automation script displayed in a new tab, as shown in Figure 4.
Figure 4: Source code that was successfully uploaded
- Choose the Code tab.
- Scroll down to the Runtime settings pane and choose Edit.
- For Handler, enter cw-blog-remediation.lambda_handler for your function handler, and then choose Save, as shown in Figure 5.
Figure 5: Updated Lambda handler
- For security purposes, and to follow the principle of least privilege, you should also add an inline policy to the Lambda function’s role to perform the tasks necessary to enable versioning on S3 buckets.
- In the Lambda console, navigate to the Configuration tab and then, in the left navigation pane, choose Permissions. Choose the Role name, as shown in Figure 6.
Figure 6: Lambda role in the AWS console
- In the Add permissions dropdown, select Create inline policy.
Figure 7: Create inline policy
- Choose JSON, add the following policy to the policy editor, and then choose Next.
- Name the policy PutBucketVersioning and choose Create policy.
- In the Lambda console, navigate to the Configuration tab and then, in the left navigation pane, choose Permissions. Choose the Role name, as shown in Figure 6.
Create a custom action in Security Hub
In this step, you will create a custom action in Security Hub.
To create the custom action
- Open the Security Hub console.
- In the left navigation pane, choose Settings, and then choose Custom actions.
- Choose Create custom action.
- Provide the following information, as shown in Figure 8:
- For Name, enter TurnOnS3Versioning.
- For Description, enter Action that will turn on versioning for a specific S3 bucket.
- For Custom action ID, enter TurnOnS3Versioning.
Figure 8: Create a custom action in Security Hub
- Choose Create custom action.
- Make a note of the Custom action ARN. You will need this ARN when you create a rule to associate with the custom action in EventBridge.
Create an EventBridge rule to target the Lambda function
The next step is to create an EventBridge rule to capture the custom action. You will define an EventBridge rule that matches events (in this case, findings) from Security Hub that were forwarded by the custom action that you defined previously.
To create the EventBridge rule
- Navigate to the EventBridge console.
- On the right side, choose Create rule.
- On the Define rule detail page, give your rule a name and description that represents the rule’s purpose—for example, you could use the same name and description that you used for the custom action. Then choose Next.
- Scroll down to Event pattern, and then do the following:
- For Event source, make sure that AWS services is selected.
- For AWS service, select Security Hub.
- For Event type, select Security Hub Findings – Custom Action.
- Select Specific custom action ARN(s) and enter the ARN for the custom action that you created earlier.
Figure 9: Specify the EventBridge event pattern for the Security Hub custom action workflow
As you provide this information, the Event pattern updates.
- Choose Next.
- On the Select target(s) step, in the Select a target dropdown, select Lambda function. Then from the Function dropdown, select sec_remediation_function.
- Choose Next.
- On the Configure tags step, choose Next.
- On the Review and create step, choose Create rule.
Run the automation
Your automation is set up and you can now test the automation. This test covers a partial automation workflow, since you will manually select the finding and apply the remediation workflow to one or more selected findings.
Important: As we mentioned earlier, if you decide to make the automation end-to-end, you should assess the impact of the workflow in a non-production environment. Additionally, you may want to consider creating preventative controls if you want to minimize the risk of event occurrence across an entire environment.
To run the automation
- In the Security Hub console, on the Findings tab, add a filter by entering Title in the search box and selecting that filter. Select IS and enter S3 general purpose buckets should have versioning enabled (case sensitive). Choose Apply.
- In the filtered list, choose the Title of an active finding.
- Before you start the automation, check the current configuration of the S3 bucket to confirm that your automation works. Expand the Resources section of the finding.
- Under Resource ID, choose the link for the S3 bucket. This opens a new tab on the S3 console that shows only this S3 bucket.
- In your browser, go back to the Security Hub tab (don’t close the S3 tab—you will need to return to it), and on the left side, select this same finding, as shown in Figure 10.
Figure 10: Filter out Security Hub findings to list only S3 bucket-related findings
- In the Actions dropdown list, choose the name of your custom action.
Figure 11: Choose the custom action that you created to start the remediation workflow
- When you see a banner that displays Successfully started action…, go back to the S3 browser tab and refresh it. Verify that the S3 versioning configuration on the bucket has been enabled as shown in figure 12.
Figure 12: Versioning successfully enabled
Conclusion
In this post, you learned how to use CodeWhisperer to produce AI-generated code for custom remediations for a security use case. We encourage you to experiment with CodeWhisperer to create Lambda functions that remediate other Security Hub findings that might exist in your account, such as the enforcement of lifecycle policies on S3 buckets with versioning enabled, or using automation to remove multiple unused Amazon EC2 elastic IP addresses. The ability to automatically set public S3 buckets to private is just one of many use cases where CodeWhisperer can generate code to help you remediate Security Hub findings.
To sum up, CodeWhisperer acts as a tool that can help boost the productivity of security experts who have coding abilities, assisting them to swiftly write code to address security issues. However, security specialists should continue building their software development capabilities to implement robust solutions. Engineers should carefully review and test any generated code, since human oversight is still vital for security.
If you have feedback about this post, submit comments in the Comments section below. If you have questions about this post, contact AWS Support.