Post Syndicated from Ben Garside original https://www.raspberrypi.org/blog/localising-ai-education-adapting-experience-ai-resources/
It’s been almost a year since we launched our first set of Experience AI resources in the UK, and we’re now working with partner organisations to bring AI literacy to teachers and students all over the world.
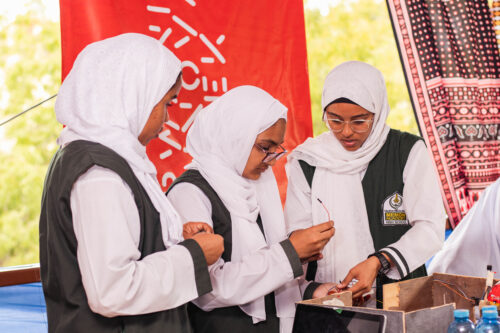
Developed by the Raspberry Pi Foundation and Google DeepMind, Experience AI provides everything that teachers need to confidently deliver engaging lessons that will inspire and educate young people about AI and the role that it could play in their lives.
Over the past six months we have been working with partners in Canada, Kenya, Malaysia, and Romania to create bespoke localised versions of the Experience AI resources. Here is what we’ve learned in the process.
Creating culturally relevant resources
The Experience AI Lessons address a variety of real-world contexts to support the concepts being taught. Including real-world contexts in teaching is a pedagogical strategy we at the Raspberry Pi Foundation call “making concrete”. This strategy significantly enhances the learning experience for learners because it bridges the gap between theoretical knowledge and practical application.
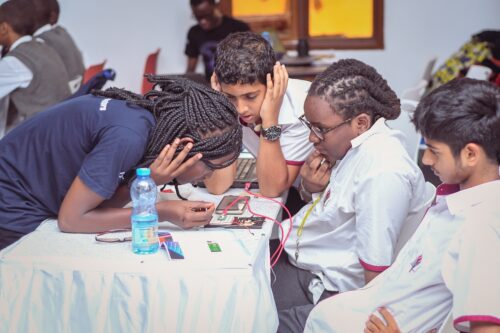
The initial aim of Experience AI was for the resources to be used in UK schools. While we put particular emphasis on using culturally relevant pedagogy to make the resources relatable to learners from backgrounds that are underrepresented in the tech industry, the contexts we included in them were for UK learners. As many of the resource writers and contributors were also based in the UK, we also unavoidably brought our own lived experiences and unintentional biases to our design thinking.
Therefore, when we began thinking about how to adapt the resources for schools in other countries, we knew we needed to make sure that we didn’t just convert what we had created into different languages. Instead we focused on localisation.
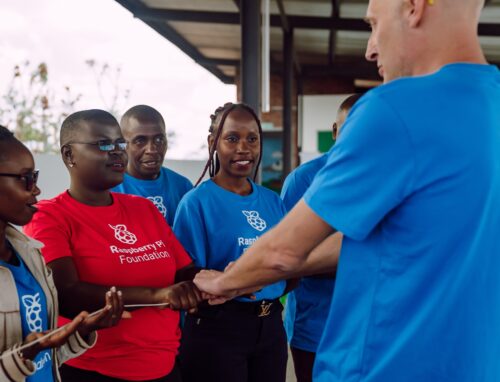
Localisation goes beyond translating resources into a different language. For example in educational resources, the real-world contexts used to make concrete the concepts being taught need to be culturally relevant, accessible, and engaging for students in a specific place. In properly localised resources, these contexts have been adapted to provide educators with a more relatable and effective learning experience that resonates with the students’ everyday lives and cultural background.
Working with partners on localisation
Recognising our UK-focused design process, we made sure that we made no assumptions during localisation. We worked with partner organisations in the four countries — Digital Moment, Tech Kidz Africa, Penang Science Cluster, and Asociația Techsoup — drawing on their expertise regarding their educational context and the real-world examples that would resonate with young people in their countries.
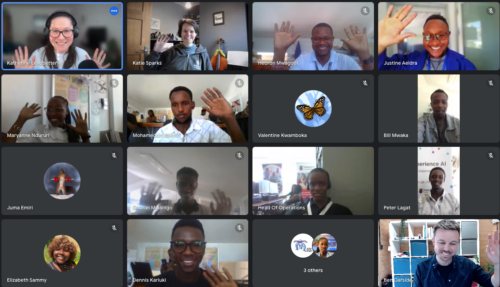
We asked our partners to look through each of the Experience AI resources and point out the things that they thought needed to change. We then worked with them to find alternative contexts that would resonate with their students, whilst ensuring the resources’ intended learning objectives would still be met.
Spotlight on localisation for Kenya
Tech Kidz Africa, our partner in Kenya, challenged some of the assumptions we had made when writing the original resources.
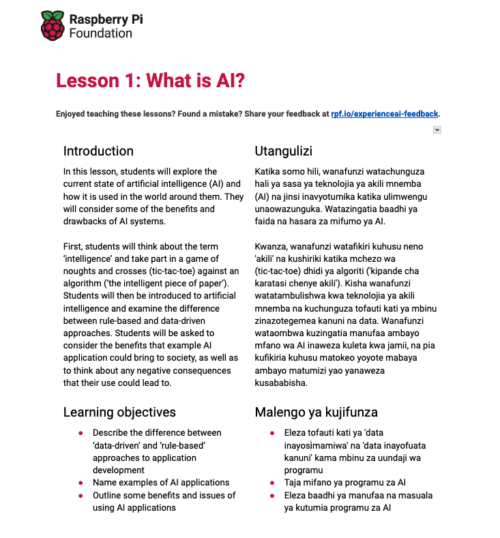
Relevant applications of AI technology
Tech Kidz Africa wanted the contexts in the lessons to not just be relatable to their students, but also to demonstrate real-world uses of AI applications that could make a difference in learners’ communities. They highlighted that as agriculture is the largest contributor to the Kenyan economy, there was an opportunity to use this as a key theme for making the Experience AI lessons more culturally relevant.
This conversation with Tech Kidz Africa led us to identify a real-world use case where farmers in Kenya were using an AI application that identifies disease in crops and provides advice on which pesticides to use. This helped the farmers to increase their crop yields.
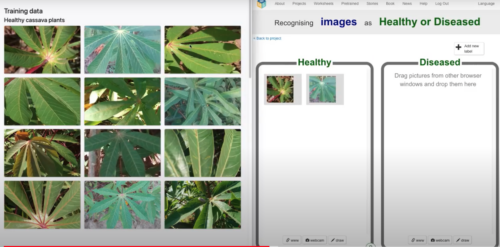
We included this example when we adapted an activity where students explore the use of AI for “computer vision”. A Google DeepMind research engineer, who is one of the General Chairs of the Deep Learning Indaba, recommended a data set of images of healthy and diseased cassava crops (1). We were therefore able to include an activity where students build their own machine learning models to solve this real-world problem for themselves.
Access to technology
While designing the original set of Experience AI resources, we made the assumption that the vast majority of students in UK classrooms have access to computers connected to the internet. This is not the case in Kenya; neither is it the case in many other countries across the world. Therefore, while we localised the Experience AI resources with our Kenyan partner, we made sure that the resources allow students to achieve the same learning outcomes whether or not they have access to internet-connected computers.
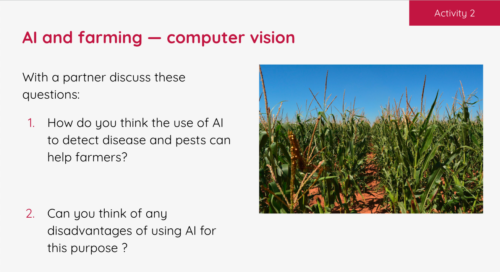
Assuming teachers in Kenya are able to download files in advance of lessons, we added “unplugged” options to activities where needed, as well as videos that can be played offline instead of being streamed on an internet-connected device.
What we’ve learned
The work with our first four Experience AI partners has given us with lots of localisation learnings, which we will use as we continue to expand the programme with more partners across the globe:
- Cultural specificity: We gained insight into which contexts are not appropriate for non-UK schools, and which contexts all our partners found relevant.
- Importance of local experts: We know we need to make sure we involve not just people who live in a country, but people who have a wealth of experience of working with learners and understand what is relevant to them.
- Adaptation vs standardisation: We have learned about the balance between adapting resources and maintaining the same progression of learning across the Experience AI resources.
Throughout this process we have also reflected on the design principles for our resources and the choices we can make while we create more Experience AI materials in order to make them more amenable to localisation.
Join us as an Experience AI partner
We are very grateful to our partners for collaborating with us to localise the Experience AI resources. Thank you to Digital Moment, Tech Kidz Africa, Penang Science Cluster, and Asociația Techsoup.
We now have the tools to create resources that support a truly global community to access Experience AI in a way that resonates with them. If you’re interested in joining us as a partner, you can register your interest here.
(1) The cassava data set was published open source by Ernest Mwebaze, Timnit Gebru, Andrea Frome, Solomon Nsumba, and Jeremy Tusubira. Read their research paper about it here.
The post Localising AI education: Adapting Experience AI for global impact appeared first on Raspberry Pi Foundation.