Post Syndicated from Claire Johnson original https://www.raspberrypi.org/blog/new-resource-to-help-teachers-make-computing-culturally-relevant/
Here at the Raspberry Pi Foundation, we believe that it’s important that our academic research has a practical application. An important area of research we are engaged in is broadening participation in computing education by investigating how the subject can be made more culturally relevant — we have published several studies in this area.
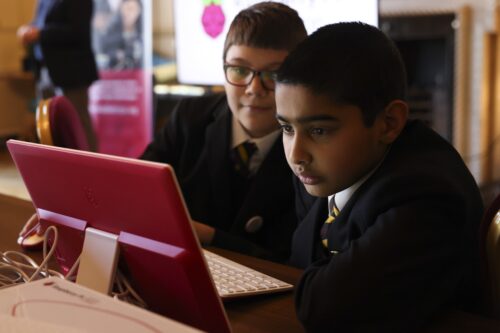
However, we know that busy teachers do not have time to keep abreast of all the latest research. This is where our Pedagogy Quick Reads come in. They show teachers how an area of current research either has been or could be applied in practice.
Our new Pedagogy Quick Reads summarises the central tenets of culturally relevant pedagogy (the theory) and then lays out 10 areas of opportunity as concrete ways for you to put the theory into practice.
Why is culturally relevant pedagogy necessary?
Computing remains an area where many groups of people are underrepresented, including those marginalised because of their gender, ethnicity, socio-economic background, additional educational needs, or age. For example, recent stats in the BCS’ Annual Diversity Report 2023 record that in the UK, the proportion of women working in tech was 20% in 2021, and Black women made up only 0.7% of tech specialists. Beyond gender and ethnicity, pupils who have fewer social and economic opportunities ‘don’t see Computing as a subject for somebody like them’, a recent report from Teach First found.
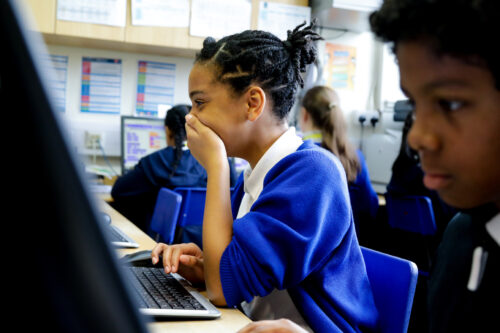
The fact that in the UK, 94% of girls and 79% of boys drop Computing at age 14 should be of particular concern for Computing educators. This last statistic makes it painfully clear that there is much work to be done to broaden the appeal of Computing in schools. One approach to make the subject more inclusive and attractive to young people is to make it more culturally relevant.
As part of our research to help teachers effectively adapt their curriculum materials to make them culturally relevant and engaging for their learners, we’ve identified 10 areas of opportunity — areas where teachers can choose to take actions to bring the latest research on culturally relevant pedagogy into their classrooms, right here, right now.
Applying the areas of opportunity in your classroom
The Pedagogy Quick Read gives teachers ideas for how they can use the areas of opportunity (AOs) to begin to review their own curriculum, teaching materials, and practices. We recommend picking one area initially, and focusing on that perhaps for a term. This helps you avoid being overwhelmed, and is particularly useful if you are trying to reach a particular group, for example, Year 9 girls, or low-attaining boys, or learners who lack confidence or motivation.
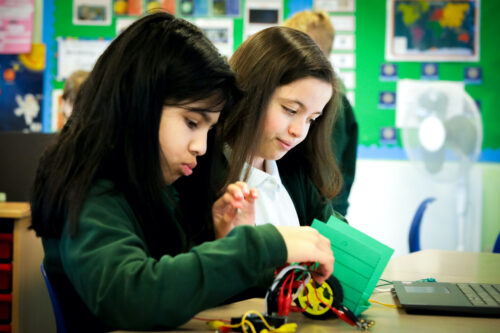
For example, one simple intervention is AO1 ‘Finding out more about our learners’. It’s all too easy for teachers to assume that they know what their students’ interests are. And getting to know your students can be especially tricky at secondary level, when teachers might only see a class once a fortnight or in a carousel.
However, finding out about your learners can be easily achieved in an online survey homework task, set at the beginning of a new academic year or term or unit of work. Using their interests, along with considerations of their backgrounds, families, and identities as inputs in curriculum planning can have tangible benefits: students may begin to feel an increased sense of belonging when they see their interests or identities reflected in the material later used.
How we’re using the AOs
The Quick Read presents two practical case studies of how we’ve used the 10 AO to adapt and assess different lesson materials to increase their relevance for learners.
Case study 1: Teachers in UK primary school adapt resources
As we’ve shared before, we implemented culturally relevant pedagogy as part of UK primary school teachers’ professional development in a recent research project. The Quick Read provides details of how we supported teachers to use the AOs to adapt teaching material to make it more culturally relevant to learners in their own contexts. Links to the resources used to review 2 units of work, lesson by lesson, to adapt tasks, learning material, and outcomes are included in the Quick Read.
Case study 2: Reflecting on the adaption of resources for a vocational course for young adults in a Kenyan refugee camp
In a different project, we used the AOs to reflect on our adaptation of classroom materials from The Computing Curriculum, which we had designed for schools in England originally. Partnering with Amala Education, we adapted Computing Curriculum materials to create a 100-hour course for young adults at Kakuma refugee camp in Kenya who wanted to develop vocational digital literacy skills.
The diagram below shows our ratings of the importance of applying each AO while adapting materials for this particular context. In this case, the most important areas for making adaptations were to make the context more culturally relevant, and to improve the materials’ accessibility in terms of readability and output formats (text, animation, video, etc.).
You can use this method of reflection as a way to evaluate your progress in addressing different AOs in a unit of work, across the materials for a whole year group, or even for your school’s whole approach. This may be useful for highlighting those areas which have, perhaps, been overlooked.
Applying research to practice with the AOs
The ‘Areas of opportunity’ Pedagogy Quick Read aims to help teachers apply research to their practice by summarising current research and giving practical examples of evidence-based teaching interventions and resources they can use.
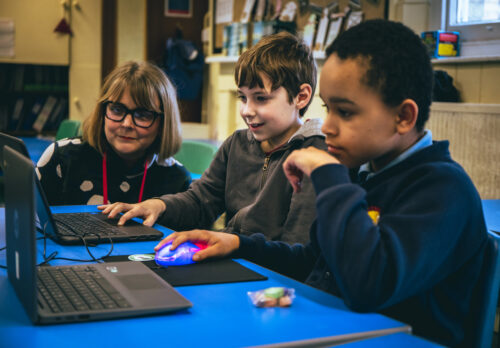
The set of AOs was developed as part of a wider research project, and each one is itself research-informed. The Quick Read includes references to that research for everyone who wants to know more about culturally relevant pedagogy. This supporting evidence will be useful to teachers who want to address the topic of culturally relevant pedagogy with senior or subject leaders in their school, who often need to know that new initiatives are evidence-based.
Our goal for the Quick Read is to raise awareness of tried and tested pedagogies that increase accessibility and broaden the appeal of Computing education, so that all of our students can develop a sense of belonging and enjoyment of Computing.
Let us know if you have a story to tell about how you have applied one of the areas of opportunity in your classroom.
To date, our research in the field of culturally relevant pedagogy has been generously supported by funders including Cognizant and Google. We are very grateful to our partners for enabling us to learn more about how to make computing education inclusive for all.
The post New resource to help teachers make Computing culturally relevant appeared first on Raspberry Pi Foundation.