Post Syndicated from Celso Martinho original https://blog.cloudflare.com/ai-side-channel-attack-mitigated
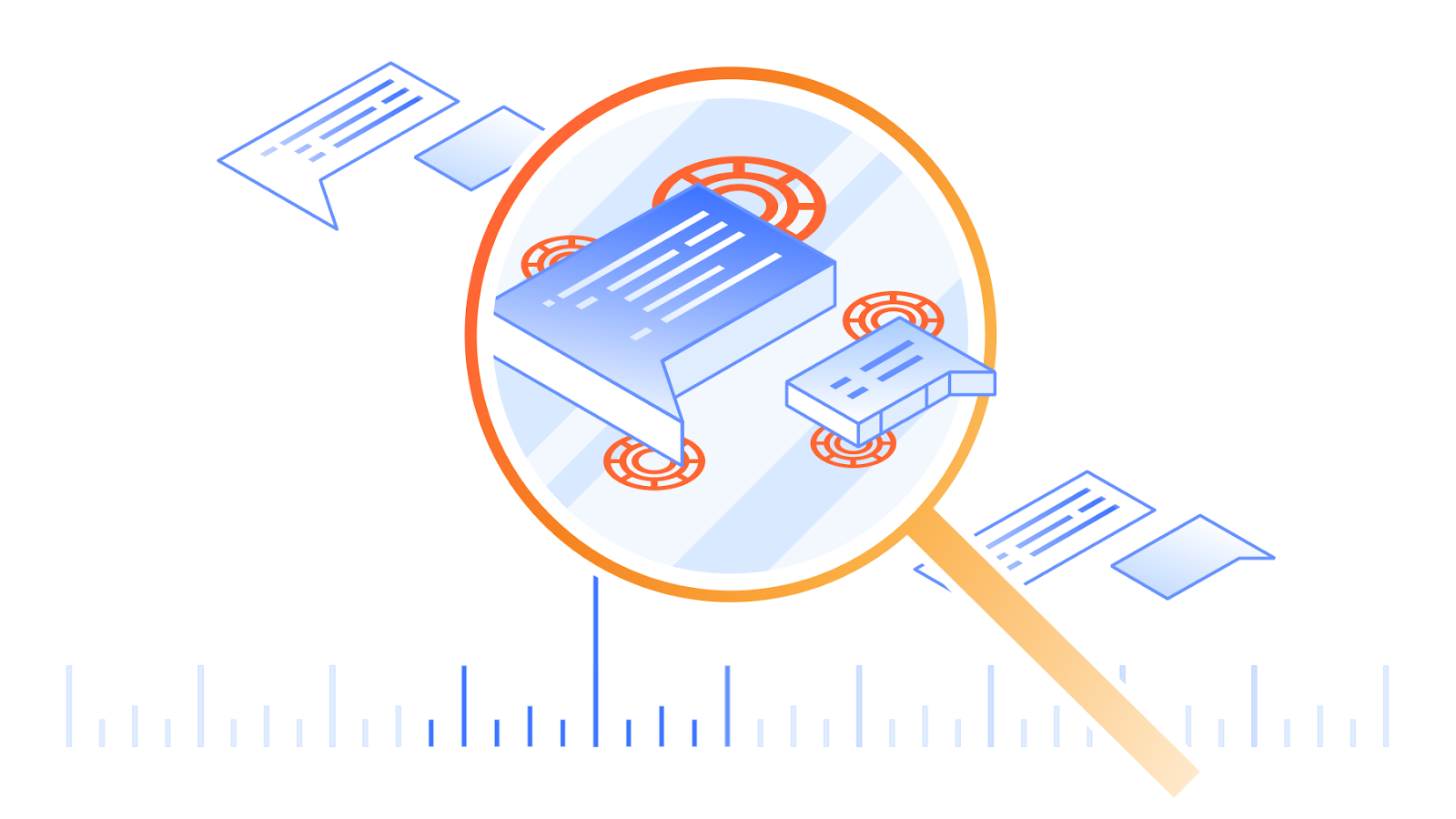
Since the discovery of CRIME, BREACH, TIME, LUCKY-13 etc., length-based side-channel attacks have been considered practical. Even though packets were encrypted, attackers were able to infer information about the underlying plaintext by analyzing metadata like the packet length or timing information.
Cloudflare was recently contacted by a group of researchers at Ben Gurion University who wrote a paper titled “What Was Your Prompt? A Remote Keylogging Attack on AI Assistants” that describes “a novel side-channel that can be used to read encrypted responses from AI Assistants over the web”.
The Workers AI and AI Gateway team collaborated closely with these security researchers through our Public Bug Bounty program, discovering and fully patching a vulnerability that affects LLM providers. You can read the detailed research paper here.
Since being notified about this vulnerability, we’ve implemented a mitigation to help secure all Workers AI and AI Gateway customers. As far as we could assess, there was no outstanding risk to Workers AI and AI Gateway customers.
How does the side-channel attack work?
In the paper, the authors describe a method in which they intercept the stream of a chat session with an LLM provider, use the network packet headers to infer the length of each token, extract and segment their sequence, and then use their own dedicated LLMs to infer the response.
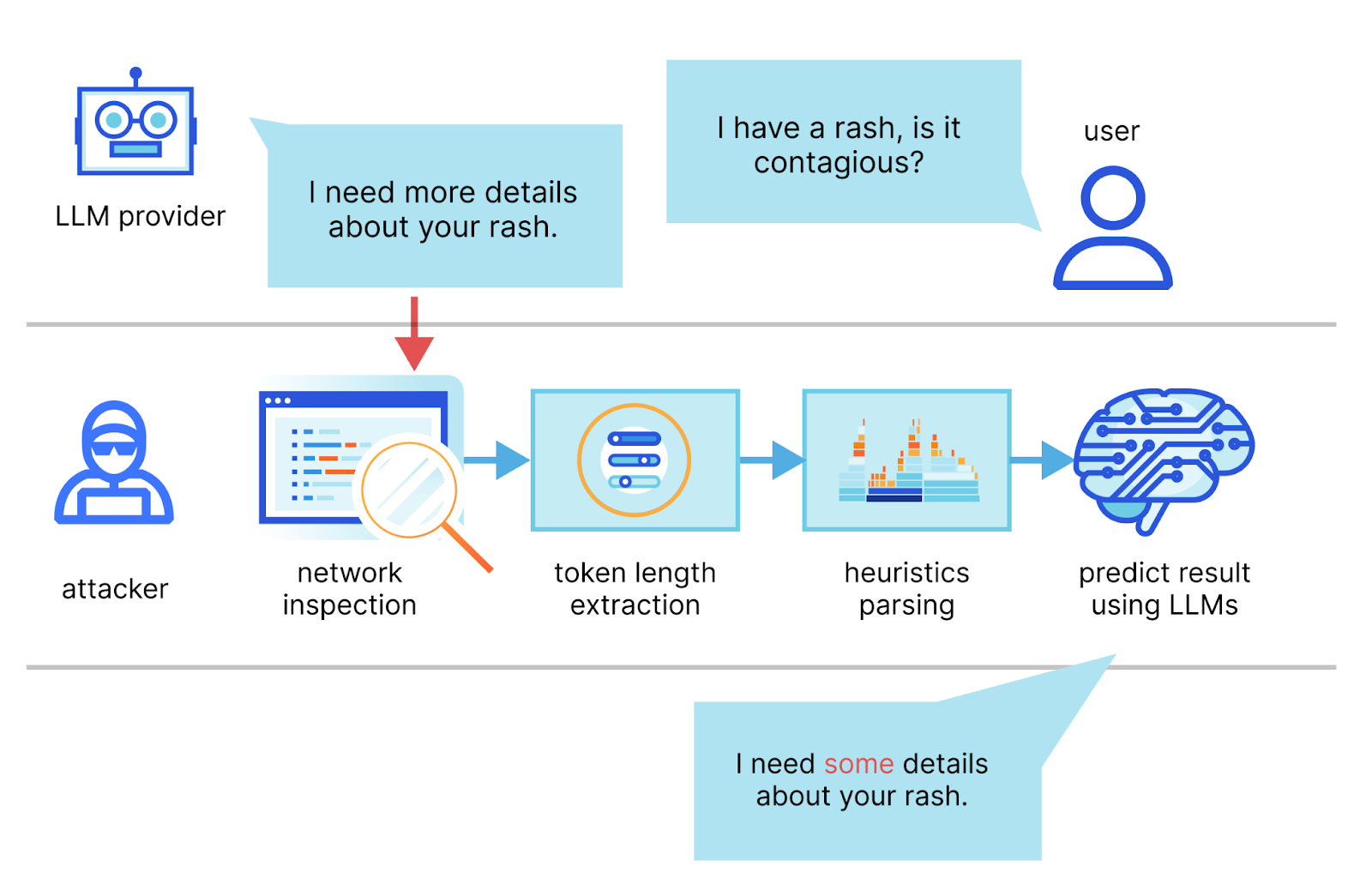
The two main requirements for a successful attack are an AI chat client running in streaming mode and a malicious actor capable of capturing network traffic between the client and the AI chat service. In streaming mode, the LLM tokens are emitted sequentially, introducing a token-length side-channel. Malicious actors could eavesdrop on packets via public networks or within an ISP.
An example request vulnerable to the side-channel attack looks like this:
curl -X POST \
https://api.cloudflare.com/client/v4/accounts/<account-id>/ai/run/@cf/meta/llama-2-7b-chat-int8 \
-H "Authorization: Bearer <Token>" \
-d '{"stream":true,"prompt":"tell me something about portugal"}'
Let’s use Wireshark to inspect the network packets on the LLM chat session while streaming:
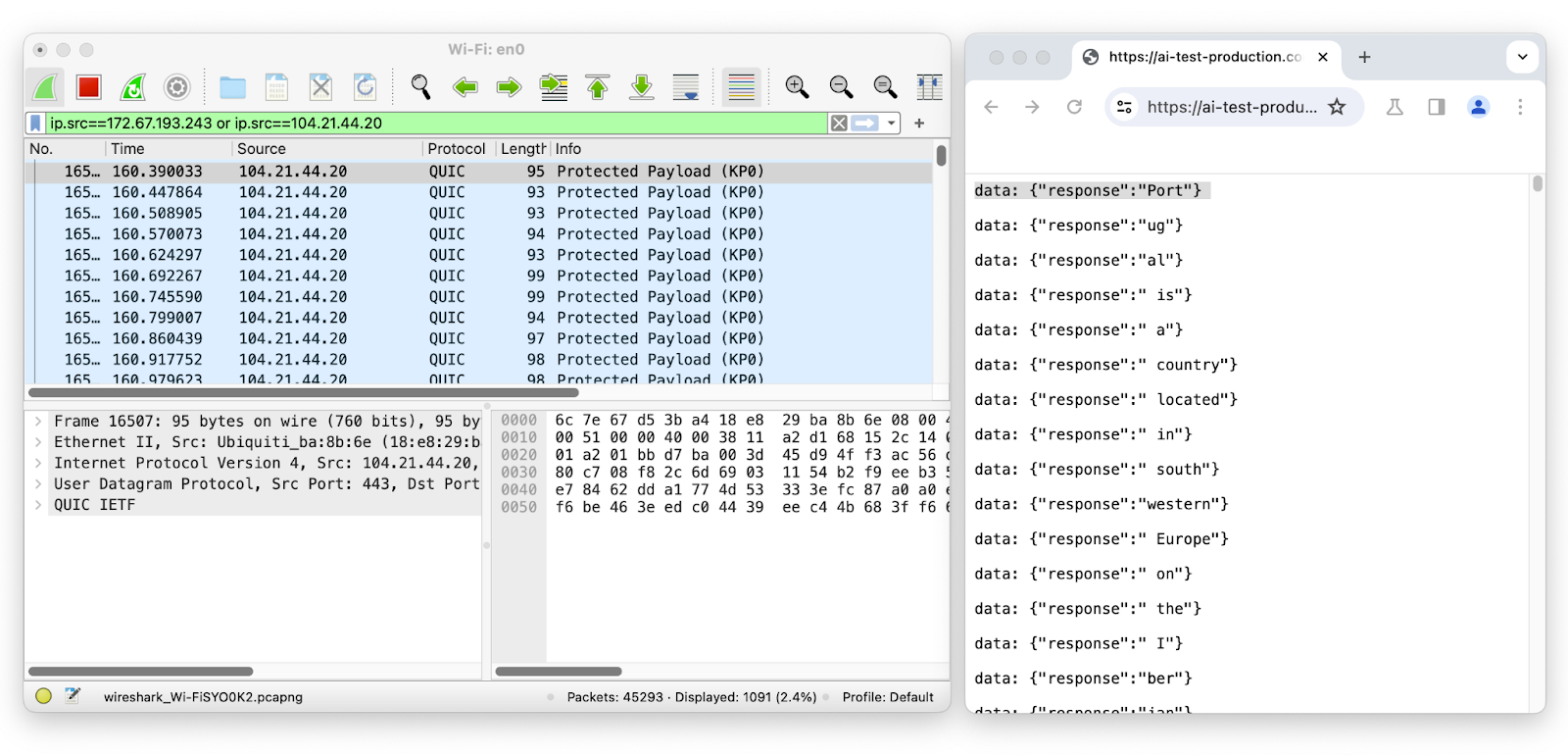
The first packet has a length of 95 and corresponds to the token “Port” which has a length of four. The second packet has a length of 93 and corresponds to the token “ug” which has a length of two, and so on. By removing the likely token envelope from the network packet length, it is easy to infer how many tokens were transmitted and their sequence and individual length just by sniffing encrypted network data.
Since the attacker needs the sequence of individual token length, this vulnerability only affects text generation models using streaming. This means that AI inference providers that use streaming — the most common way of interacting with LLMs — like Workers AI, are potentially vulnerable.
This method requires that the attacker is on the same network or in a position to observe the communication traffic and its accuracy depends on knowing the target LLM’s writing style. In ideal conditions, the researchers claim that their system “can reconstruct 29% of an AI assistant’s responses and successfully infer the topic from 55% of them”. It’s also important to note that unlike other side-channel attacks, in this case the attacker has no way of evaluating its prediction against the ground truth. That means that we are as likely to get a sentence with near perfect accuracy as we are to get one where only things that match are conjunctions.
Mitigating LLM side-channel attacks
Since this type of attack relies on the length of tokens being inferred from the packet, it can be just as easily mitigated by obscuring token size. The researchers suggested a few strategies to mitigate these side-channel attacks, one of which is the simplest: padding the token responses with random length noise to obscure the length of the token so that responses can not be inferred from the packets. While we immediately added the mitigation to our own inference product — Workers AI, we wanted to help customers secure their LLMs regardless of where they are running them by adding it to our AI Gateway.
As of today, all users of Workers AI and AI Gateway are now automatically protected from this side-channel attack.
What we did
Once we got word of this research work and how exploiting the technique could potentially impact our AI products, we did what we always do in situations like this: we assembled a team of systems engineers, security engineers, and product managers and started discussing risk mitigation strategies and next steps. We also had a call with the researchers, who kindly attended, presented their conclusions, and answered questions from our teams.
Unfortunately, at this point, this research does not include actual code that we can use to reproduce the claims or the effectiveness and accuracy of the described side-channel attack. However, we think that the paper has theoretical merit, that it provides enough detail and explanations, and that the risks are not negligible.
We decided to incorporate the first mitigation suggestion in the paper: including random padding to each message to hide the actual length of tokens in the stream, thereby complicating attempts to infer information based solely on network packet size.
Workers AI, our inference product, is now protected
With our inference-as-a-service product, anyone can use the Workers AI platform and make API calls to our supported AI models. This means that we oversee the inference requests being made to and from the models. As such, we have a responsibility to ensure that the service is secure and protected from potential vulnerabilities. We immediately rolled out a fix once we were notified of the research, and all Workers AI customers are now automatically protected from this side-channel attack. We have not seen any malicious attacks exploiting this vulnerability, other than the ethical testing from the researchers.
Our solution for Workers AI is a variation of the mitigation strategy suggested in the research document. Since we stream JSON objects rather than the raw tokens, instead of padding the tokens with whitespace characters, we added a new property, “p” (for padding) that has a string value of variable random length.
Example streaming response using the SSE syntax:
data: {"response":"portugal","p":"abcdefghijklmnopqrstuvwxyz0123456789a"}
data: {"response":" is","p":"abcdefghij"}
data: {"response":" a","p":"abcdefghijklmnopqrstuvwxyz012"}
data: {"response":" southern","p":"ab"}
data: {"response":" European","p":"abcdefgh"}
data: {"response":" country","p":"abcdefghijklmno"}
data: {"response":" located","p":"abcdefghijklmnopqrstuvwxyz012345678"}
This has the advantage that no modifications are required in the SDK or the client code, the changes are invisible to the end-users, and no action is required from our customers. By adding random variable length to the JSON objects, we introduce the same network-level variability, and the attacker essentially loses the required input signal. Customers can continue using Workers AI as usual while benefiting from this protection.
One step further: AI Gateway protects users of any inference provider
We added protection to our AI inference product, but we also have a product that proxies requests to any provider — AI Gateway. AI Gateway acts as a proxy between a user and supported inference providers, helping developers gain control, performance, and observability over their AI applications. In line with our mission to help build a better Internet, we wanted to quickly roll out a fix that can help all our customers using text generation AIs, regardless of which provider they use or if they have mitigations to prevent this attack. To do this, we implemented a similar solution that pads all streaming responses proxied through AI Gateway with random noise of variable length.
Our AI Gateway customers are now automatically protected against this side-channel attack, even if the upstream inference providers have not yet mitigated the vulnerability. If you are unsure if your inference provider has patched this vulnerability yet, use AI Gateway to proxy your requests and ensure that you are protected.
Conclusion
At Cloudflare, our mission is to help build a better Internet – that means that we care about all citizens of the Internet, regardless of what their tech stack looks like. We are proud to be able to improve the security of our AI products in a way that is transparent and requires no action from our customers.
We are grateful to the researchers who discovered this vulnerability and have been very collaborative in helping us understand the problem space. If you are a security researcher who is interested in helping us make our products more secure, check out our Bug Bounty program at hackerone.com/cloudflare.