Post Syndicated from Steve Welham original https://blog.cloudflare.com/introducing-magic-cloud-networking
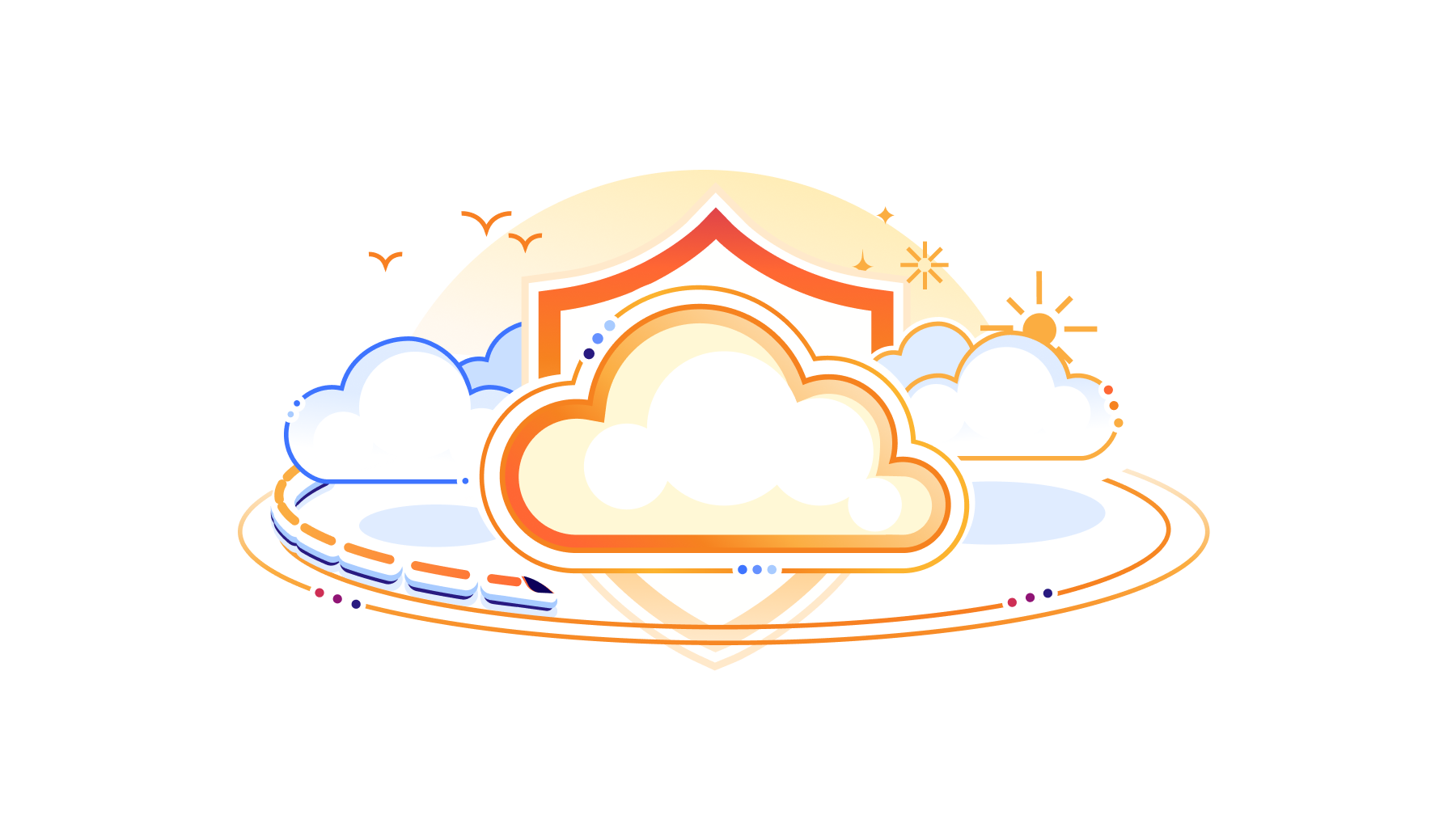
Today we are excited to announce Magic Cloud Networking, supercharged by Cloudflare’s recent acquisition of Nefeli Networks’ innovative technology. These new capabilities to visualize and automate cloud networks will give our customers secure, easy, and seamless connection to public cloud environments.
Public clouds offer organizations a scalable and on-demand IT infrastructure without the overhead and expense of running their own datacenter. Cloud networking is foundational to applications that have been migrated to the cloud, but is difficult to manage without automation software, especially when operating at scale across multiple cloud accounts. Magic Cloud Networking uses familiar concepts to provide a single interface that controls and unifies multiple cloud providers’ native network capabilities to create reliable, cost-effective, and secure cloud networks.
Nefeli’s approach to multi-cloud networking solves the problem of building and operating end-to-end networks within and across public clouds, allowing organizations to securely leverage applications spanning any combination of internal and external resources. Adding Nefeli’s technology will make it easier than ever for our customers to connect and protect their users, private networks and applications.
Why is cloud networking difficult?
Compared with a traditional on-premises data center network, cloud networking promises simplicity:
- Much of the complexity of physical networking is abstracted away from users because the physical and ethernet layers are not part of the network service exposed by the cloud provider.
- There are fewer control plane protocols; instead, the cloud providers deliver a simplified software-defined network (SDN) that is fully programmable via API.
- There is capacity — from zero up to very large — available instantly and on-demand, only charging for what you use.
However, that promise has not yet been fully realized. Our customers have described several reasons cloud networking is difficult:
- Poor end-to-end visibility: Cloud network visibility tools are difficult to use and silos exist even within single cloud providers that impede end-to-end monitoring and troubleshooting.
- Faster pace: Traditional IT management approaches clash with the promise of the cloud: instant deployment available on-demand. Familiar ClickOps and CLI-driven procedures must be replaced by automation to meet the needs of the business.
- Different technology: Established network architectures in on-premises environments do not seamlessly transition to a public cloud. The missing ethernet layer and advanced control plane protocols were critical in many network designs.
- New cost models: The dynamic pay-as-you-go usage-based cost models of the public clouds are not compatible with established approaches built around fixed cost circuits and 5-year depreciation. Network solutions are often architected with financial constraints, and accordingly, different architectural approaches are sensible in the cloud.
- New security risks: Securing public clouds with true zero trust and least-privilege demands mature operating processes and automation, and familiarity with cloud-specific policies and IAM controls.
- Multi-vendor: Oftentimes enterprise networks have used single-vendor sourcing to facilitate interoperability, operational efficiency, and targeted hiring and training. Operating a network that extends beyond a single cloud, into other clouds or on-premises environments, is a multi-vendor scenario.
Nefeli considered all these problems and the tensions between different customer perspectives to identify where the problem should be solved.
Trains, planes, and automation
Consider a train system. To operate effectively it has three key layers:
- tracks and trains
- electronic signals
- a company to manage the system and sell tickets.
A train system with good tracks, trains, and signals could still be operating below its full potential because its agents are unable to keep up with passenger demand. The result is that passengers cannot plan itineraries or purchase tickets.
The train company eliminates bottlenecks in process flow by simplifying the schedules, simplifying the pricing, providing agents with better booking systems, and installing automated ticket machines. Now the same fast and reliable infrastructure of tracks, trains, and signals can be used to its full potential.
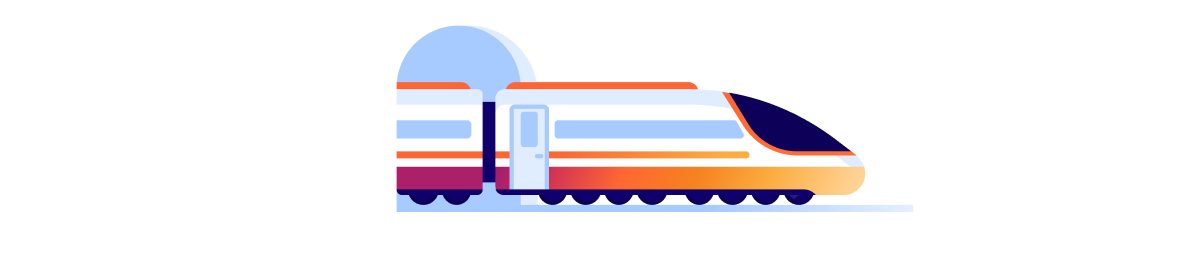
Solve the right problem
In networking, there are an analogous set of three layers, called the networking planes:
- Data Plane: the network paths that transport data (in the form of packets) from source to destination.
- Control Plane: protocols and logic that change how packets are steered across the data plane.
- Management Plane: the configuration and monitoring interfaces for the data plane and control plane.
In public cloud networks, these layers map to:
- Cloud Data Plane: The underlying cables and devices are exposed to users as the Virtual Private Cloud (VPC) or Virtual Network (VNet) service that includes subnets, routing tables, security groups/ACLs and additional services such as load-balancers and VPN gateways.
- Cloud Control Plane: In place of distributed protocols, the cloud control plane is a software defined network (SDN) that, for example, programs static route tables. (There is limited use of traditional control plane protocols, such as BGP to interface with external networks and ARP to interface with VMs.)
- Cloud Management Plane: An administrative interface with a UI and API which allows the admin to fully configure the data and control planes. It also provides a variety of monitoring and logging capabilities that can be enabled and integrated with 3rd party systems.
Like our train example, most of the problems that our customers experience with cloud networking are in the third layer: the management plane.
Nefeli simplifies, unifies, and automates cloud network management and operations.
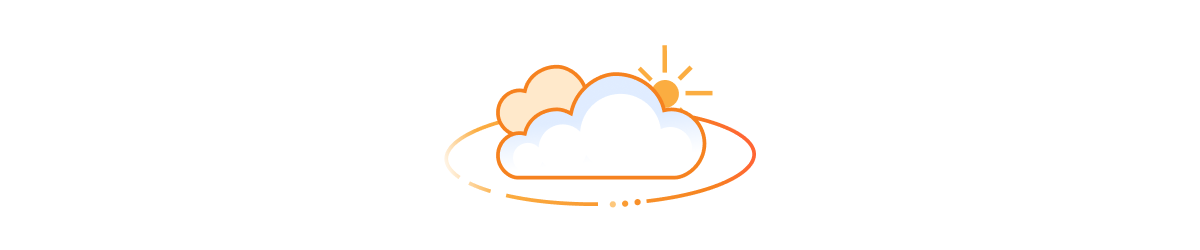
Avoid cost and complexity
One common approach to tackle management problems in cloud networks is introducing Virtual Network Functions (VNFs), which are virtual machines (VMs) that do packet forwarding, in place of native cloud data plane constructs. Some VNFs are routers, firewalls, or load-balancers ported from a traditional network vendor’s hardware appliances, while others are software-based proxies often built on open-source projects like NGINX or Envoy. Because VNFs mimic their physical counterparts, IT teams could continue using familiar management tooling, but VNFs have downsides:
- VMs do not have custom network silicon and so instead rely on raw compute power. The VM is sized for the peak anticipated load and then typically runs 24x7x365. This drives a high cost of compute regardless of the actual utilization.
- High-availability (HA) relies on fragile, costly, and complex network configuration.
- Service insertion — the configuration to put a VNF into the packet flow — often forces packet paths that incur additional bandwidth charges.
- VNFs are typically licensed similarly to their on-premises counterparts and are expensive.
- VNFs lock in the enterprise and potentially exclude them benefitting from improvements in the cloud’s native data plane offerings.
For these reasons, enterprises are turning away from VNF-based solutions and increasingly looking to rely on the native network capabilities of their cloud service providers. The built-in public cloud networking is elastic, performant, robust, and priced on usage, with high-availability options integrated and backed by the cloud provider’s service level agreement.
In our train example, the tracks and trains are good. Likewise, the cloud network data plane is highly capable. Changing the data plane to solve management plane problems is the wrong approach. To make this work at scale, organizations need a solution that works together with the native network capabilities of cloud service providers.
Nefeli leverages native cloud data plane constructs rather than third party VNFs.
Introducing Magic Cloud Networking
The Nefeli team has joined Cloudflare to integrate cloud network management functionality with Cloudflare One. This capability is called Magic Cloud Networking and with it, enterprises can use the Cloudflare dashboard and API to manage their public cloud networks and connect with Cloudflare One.
End-to-end
Just as train providers are focused only on completing train journeys in their own network, cloud service providers deliver network connectivity and tools within a single cloud account. Many large enterprises have hundreds of cloud accounts across multiple cloud providers. In an end-to-end network this creates disconnected networking silos which introduce operational inefficiencies and risk.
Imagine you are trying to organize a train journey across Europe, and no single train company serves both your origin and destination. You know they all offer the same basic service: a seat on a train. However, your trip is difficult to arrange because it involves multiple trains operated by different companies with their own schedules and ticketing rates, all in different languages!
Magic Cloud Networking is like an online travel agent that aggregates multiple transportation options, books multiple tickets, facilitates changes after booking, and then delivers travel status updates.
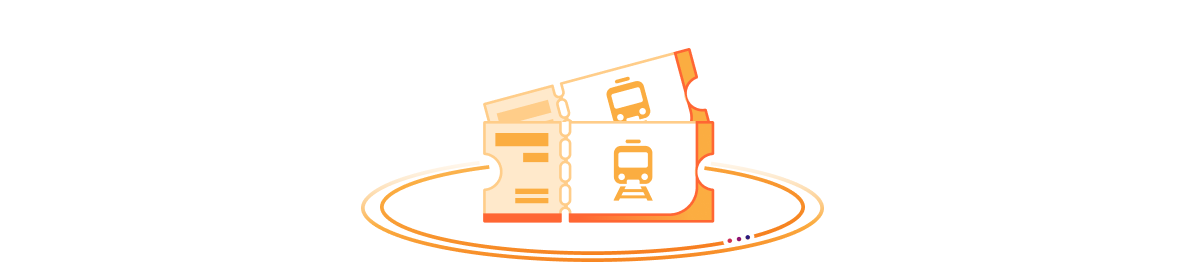
Through the Cloudflare dashboard, you can discover all of your network resources across accounts and cloud providers and visualize your end-to-end network in a single interface. Once Magic Cloud Networking discovers your networks, you can build a scalable network through a fully automated and simple workflow.
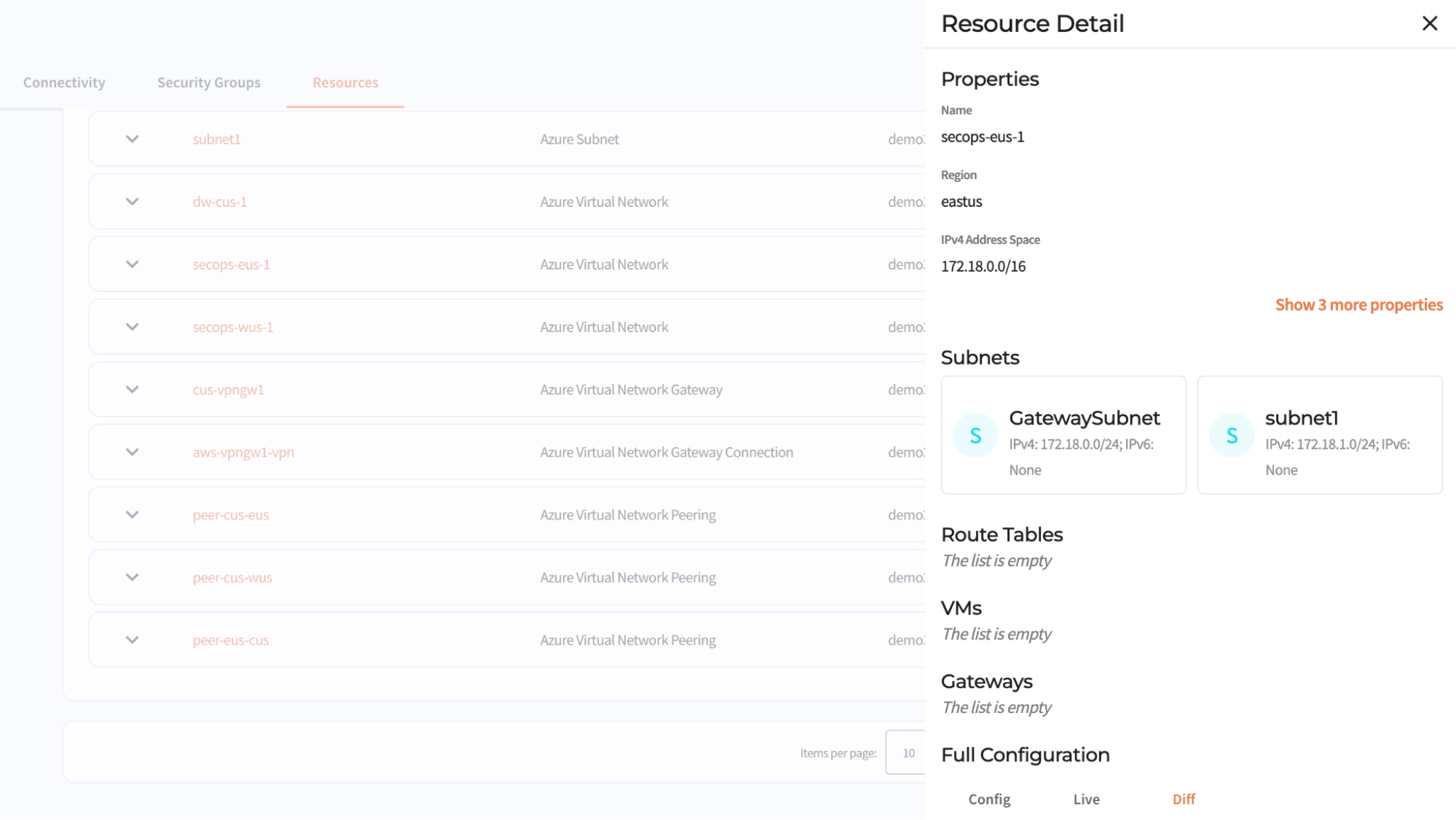
Taming per-cloud complexity
Public clouds are used to deliver applications and services. Each cloud provider offers a composable stack of modular building blocks (resources) that start with the foundation of a billing account and then add on security controls. The next foundational layer, for server-based applications, is VPC networking. Additional resources are built on the VPC network foundation until you have compute, storage, and network infrastructure to host the enterprise application and data. Even relatively simple architectures can be composed of hundreds of resources.
The trouble is, these resources expose abstractions that are different from the building blocks you would use to build a service on prem, the abstractions differ between cloud providers, and they form a web of dependencies with complex rules about how configuration changes are made (rules which differ between resource types and cloud providers). For example, say I create 100 VMs, and connect them to an IP network. Can I make changes to the IP network while the VMs are using the network? The answer: it depends.
Magic Cloud Networking handles these differences and complexities for you. It configures native cloud constructs such as VPN gateways, routes, and security groups to securely connect your cloud VPC network to Cloudflare One without having to learn each cloud’s incantations for creating VPN connections and hubs.
Continuous, coordinated automation
Returning to our train system example, what if the railway maintenance staff find a dangerous fault on the railroad track? They manually set the signal to a stop light to prevent any oncoming trains using the faulty section of track. Then, what if, by unfortunate coincidence, the scheduling office is changing the signal schedule, and they set the signals remotely which clears the safety measure made by the maintenance crew? Now there is a problem that no one knows about and the root cause is that multiple authorities can change the signals via different interfaces without coordination.
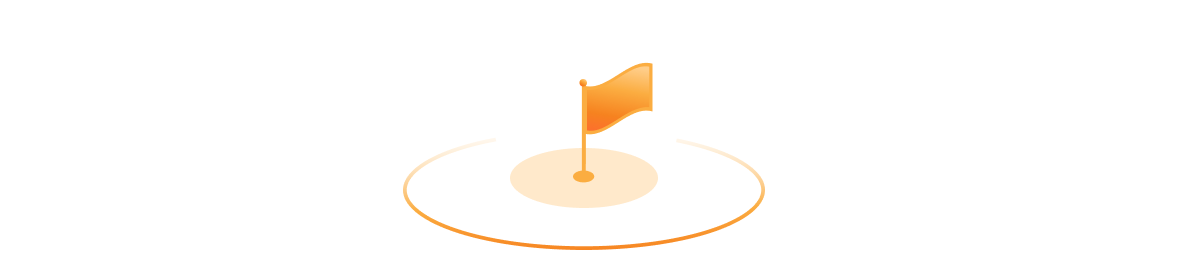
The same problem exists in cloud networks: configuration changes are made by different teams using different automation and configuration interfaces across a spectrum of roles such as billing, support, security, networking, firewalls, database, and application development.
Once your network is deployed, Magic Cloud Networking monitors its configuration and health, enabling you to be confident that the security and connectivity you put in place yesterday is still in place today. It tracks the cloud resources it is responsible for, automatically reverting drift if they are changed out-of-band, while allowing you to manage other resources, like storage buckets and application servers, with other automation tools. And, as you change your network, Cloudflare takes care of route management, injecting and withdrawing routes globally across Cloudflare and all connected cloud provider networks.
Magic Cloud Networking is fully programmable via API, and can be integrated into existing automation toolchains.
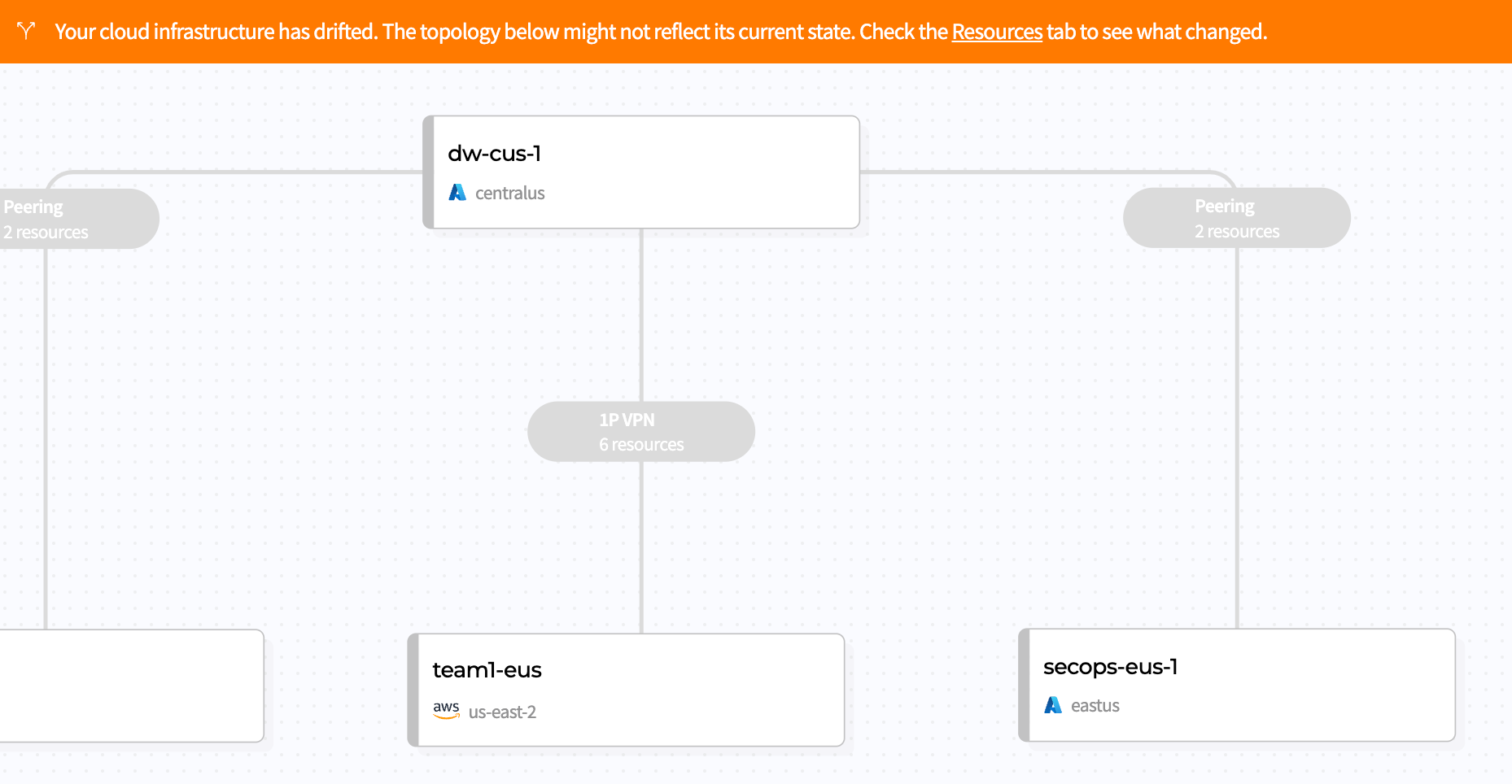
Ready to start conquering cloud networking?
We are thrilled to introduce Magic Cloud Networking as another pivotal step to fulfilling the promise of the Connectivity Cloud. This marks our initial stride in empowering customers to seamlessly integrate Cloudflare with their public clouds to get securely connected, stay securely connected, and gain flexibility and cost savings as they go.
Join us on this journey for early access: learn more and sign up here.
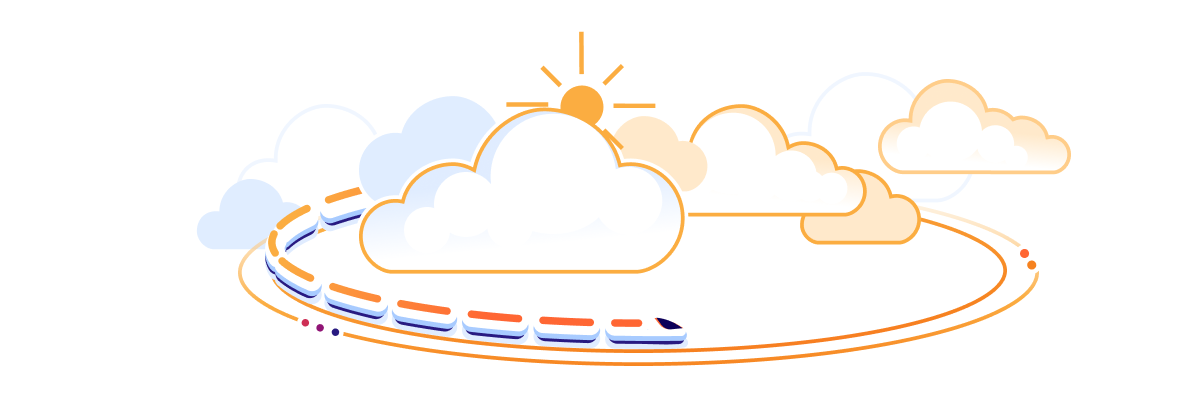