Post Syndicated from Chris Munns original https://aws.amazon.com/blogs/compute/applying-spot-to-spot-consolidation-best-practices-with-karpenter/
This post is written by Robert Northard – AWS Container Specialist Solutions Architect, and Carlos Manzanedo Rueda – AWS WW SA Leader for Efficient Compute
Karpenter is an open source node lifecycle management project built for Kubernetes. In this post, you will learn how to use the new Spot-to-Spot consolidation functionality released in Karpenter v0.34.0, which helps further optimize your cluster. Amazon Elastic Compute Cloud (Amazon EC2) Spot Instances are spare Amazon EC2 capacity available for up to 90% off compared to On-Demand prices. One difference between On-Demand and Spot is that Spot Instances can be interrupted by Amazon EC2 when the capacity is needed back. Karpenter’s built-in support for Spot Instances allows users to seamlessly implement Spot best practices and helps users optimize the cost of stateless, fault tolerant workloads. For example, when Karpenter observes a Spot interruption, it automatically starts a new node in response.
Karpenter provisions nodes in response to unschedulable pods based on aggregated CPU, memory, volume requests, and other scheduling constraints. Over time, Karpenter has added functionality to simplify instance lifecycle configuration, providing a termination controller, instance expiration, and drift detection. Karpenter also helps optimize Kubernetes clusters by selecting the optimal instances while still respecting Kubernetes pod-to-node placement nuances, such as nodeSelector, affinity and anti-affinity, taints and tolerations, and topology spread constraints.
The Kubernetes scheduler assigns pods to nodes based on their scheduling constraints. Over time, as workloads are scaled out and scaled in or as new instances join and leave, the cluster placement and instance load might end up not being optimal. In many cases, it results in unnecessary extra costs. Karpenter has a consolidation feature that improves cluster placement by identifying and taking action in situations such as:
- when a node is empty
- when a node can be removed as the pods that are running on it can be rescheduled into other existing nodes
- when the number of pods in a node has gone down and the node can now be replaced with a lower-priced and rightsized variant (which is shown in the following figure)
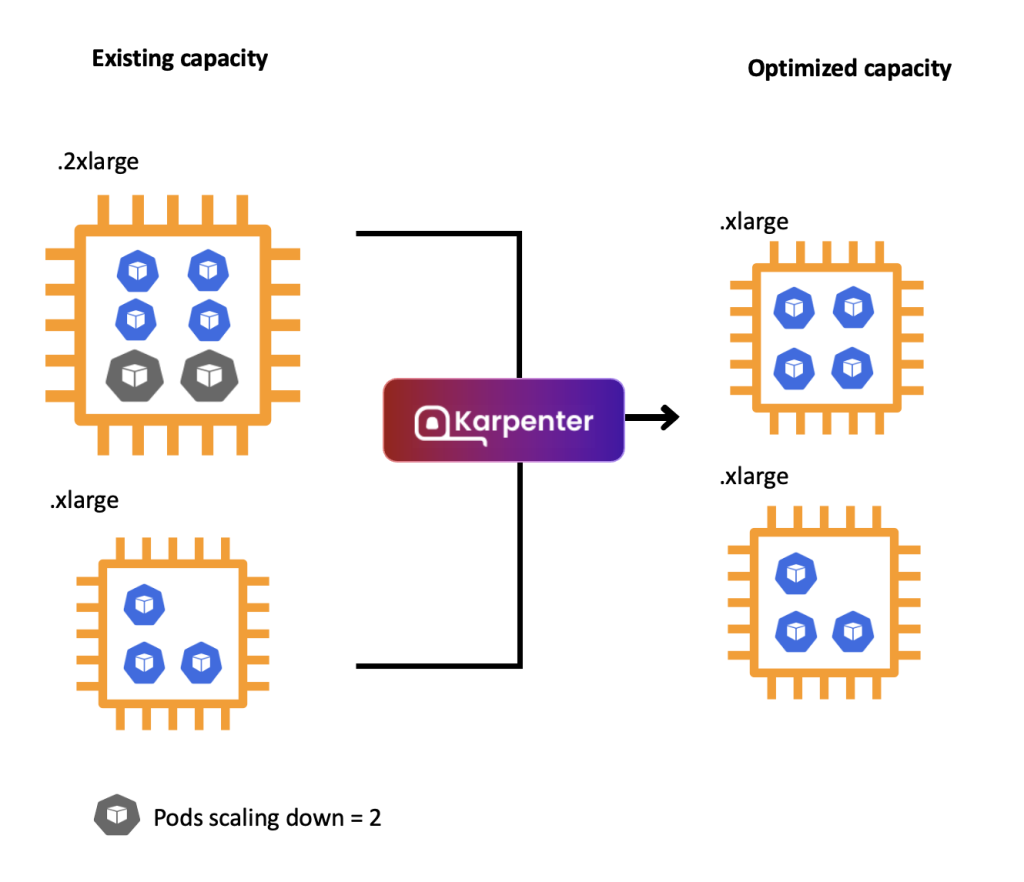
Karpenter consolidation, replacing one 2xlarge Amazon EC2 Instance with an xlarge Amazon EC2 Instance.
Karpenter versions prior to v0.34.0 only supported consolidation for Amazon EC2 On-Demand Instances. On-Demand consolidation allowed consolidating from On-Demand into Spot Instances and to lower-priced On-Demand Instances. However, once a pod was placed on a Spot Instance, Spot nodes were only removed when the nodes were empty. In v0.34.0, you can enable the feature gate to use Spot-to-Spot consolidation.
Solution overview
When launching Spot Instances, Karpenter uses the price-capacity-optimized allocation strategy when calling the Amazon EC2 instant Fleet API (shown in the following figure) and passes in a selection of compute instance types based on the Karpenter NodePool configuration. The Amazon EC2 Fleet API in instant mode is a synchronous API call that immediately returns a list of instances that launched and any instance that could not be launched. For any instances that could not be launched, Karpenter might request alternative capacity or remove any soft Kubernetes scheduling constraints for the workload.
Spot-to-Spot consolidation needed an approach that was different from On-Demand consolidation. For On-Demand consolidation, rightsizing and lowest price are the main metrics used. For Spot-to-Spot consolidation to take place, Karpenter requires a diversified instance configuration (see the example NodePool defined in the walkthrough) with at least 15 instances types. Without this constraint, there would be a risk of Karpenter selecting an instance that has lower availability and, therefore, higher frequency of interruption.
Prerequisites
The following prerequisites are required to complete the walkthrough:
- Install an Amazon Elastic Kubernetes Service (Amazon EKS) cluster (version 1.29 or higher) with Karpenter (v0.34.0 or higher). The Karpenter Getting Started Guide provides steps for setting up an Amazon EKS cluster and adding Karpenter.
- Enable replacement with Spot consolidation through the SpotToSpotConsolidation feature gate. This can be enabled during a helm install of the Karpenter chart by adding –-set settings.featureGates.spotToSpotConsolidation=true argument.
- Install kubectl, the Kubernetes command line tool for communicating with the Kubernetes control plane API, and kubectl context configured with Cluster Operator and Cluster Developer permissions.
Walkthrough
The following walkthrough guides you through the steps for simulating Spot-to-Spot consolidation.
1. Create a Karpenter NodePool and EC2NodeClass
Create a Karpenter NodePool and EC2NodeClass. Replace the following with your own values. If you used the Karpenter Getting Started Guide to create your installation, then the value would be your cluster name.
- Replace <karpenter-discovery-tag-value> with your subnet tag for Karpenter subnet and security group auto-discovery.
- Replace <role-name> with the name of the AWS Identity and Access Management (IAM) role for node identity.
cat <<EOF > nodepool.yaml
apiVersion: karpenter.sh/v1beta1
kind: NodePool
metadata:
name: default
spec:
template:
metadata:
labels:
intent: apps
spec:
nodeClassRef:
name: default
requirements:
- key: karpenter.sh/capacity-type
operator: In
values: ["spot"]
- key: karpenter.k8s.aws/instance-category
operator: In
values: ["c","m","r"]
- key: karpenter.k8s.aws/instance-size
operator: NotIn
values: ["nano","micro","small","medium"]
- key: karpenter.k8s.aws/instance-hypervisor
operator: In
values: ["nitro"]
limits:
cpu: 100
memory: 100Gi
disruption:
consolidationPolicy: WhenUnderutilized
---
apiVersion: karpenter.k8s.aws/v1beta1
kind: EC2NodeClass
metadata:
name: default
spec:
amiFamily: Bottlerocket
subnetSelectorTerms:
- tags:
karpenter.sh/discovery: "<karpenter-discovery-tag-value>"
securityGroupSelectorTerms:
- tags:
karpenter.sh/discovery: "<karpenter-discovery-tag-value>"
role: "<role-name>"
tags:
Name: karpenter.sh/nodepool/default
IntentLabel: "apps"
EOF
kubectl apply -f nodepool.yaml
The NodePool definition demonstrates a flexible configuration with instances from the C, M, or R EC2 instance families. The configuration is restricted to use smaller instance sizes but is still diversified as much as possible. For example, this might be needed in scenarios where you deploy observability DaemonSets. If your workload has specific requirements, then see the supported well-known labels in the Karpenter documentation.
2. Deploy a sample workload
Deploy a sample workload by running the following command. This command creates a Deployment with five pod replicas using the pause container image:
cat <<EOF > inflate.yaml
apiVersion: apps/v1
kind: Deployment
metadata:
name: inflate
spec:
replicas: 5
selector:
matchLabels:
app: inflate
template:
metadata:
labels:
app: inflate
spec:
nodeSelector:
intent: apps
containers:
- name: inflate
image: public.ecr.aws/eks-distro/kubernetes/pause:3.2
resources:
requests:
cpu: 1
memory: 1.5Gi
EOF
kubectl apply -f inflate.yaml
Next, check the Kubernetes nodes by running a kubectl get nodes CLI command. The capacity pool (instance type and Availability Zone) selected depends on any Kubernetes scheduling constraints and spare capacity size. Therefore, it might differ from this example in the walkthrough. You can see Karpenter launched a new node of instance type c6g.2xlarge, an AWS Graviton2-based instance, in the eu-west-1c Region:
$ kubectl get nodes -L karpenter.sh/nodepool -L node.kubernetes.io/instance-type -L topology.kubernetes.io/zone -L karpenter.sh/capacity-type
NAME STATUS ROLES AGE VERSION NODEPOOL INSTANCE-TYPE ZONE CAPACITY-TYPE
ip-10-0-12-17.eu-west-1.compute.internal Ready <none> 80s v1.29.0-eks-a5ec690 default c6g.2xlarge eu-west-1c spot
3. Scale in a sample workload to observe consolidation
To invoke a Karpenter consolidation event scale, inflate the deployment to 1. Run the following command:
kubectl scale --replicas=1 deployment/inflate
Tail the Karpenter logs by running the following command. If you installed Karpenter in a different Kubernetes namespace, then replace the name for the -n argument in the command:
kubectl -n karpenter logs -l app.kubernetes.io/name=karpenter --all-containers=true -f --tail=20
After a few seconds, you should see the following disruption via consolidation message in the Karpenter logs. The message indicates the c6g.2xlarge Spot node has been targeted for replacement and Karpenter has passed the following 15 instance types—m6gd.xlarge, m5dn.large, c7a.xlarge, r6g.large, r6a.xlarge and 10 other(s)—to the Amazon EC2 Fleet API:
{"level":"INFO","time":"2024-02-19T12:09:50.299Z","logger":"controller.disruption","message":"disrupting via consolidation replace, terminating 1 candidates ip-10-0-12-181.eu-west-1.compute.internal/c6g.2xlarge/spot and replacing with spot node from types m6gd.xlarge, m5dn.large, c7a.xlarge, r6g.large, r6a.xlarge and 10 other(s)","commit":"17d6c05","command-id":"60f27cb5-98fa-40fb-8231-05b31fd41892"}
Check the Kubernetes nodes by running the following kubectl get nodes CLI command. You can see that Karpenter launched a new node of instance type c6g.large:
$ kubectl get nodes -L karpenter.sh/nodepool -L node.kubernetes.io/instance-type -L topology.kubernetes.io/zone -L karpenter.sh/capacity-type
NAME STATUS ROLES AGE VERSION NODEPOOL INSTANCE-TYPE ZONE CAPACITY-TYPE
ip-10-0-12-156.eu-west-1.compute.internal Ready <none> 2m1s v1.29.0-eks-a5ec690 default c6g.large eu-west-1c spot
Use kubectl get nodeclaims to list all objects of type NodeClaim and then describe the NodeClaim Kubernetes resource using kubectl get nodeclaim/<claim-name> -o yaml. In the NodeClaim .spec.requirements, you can also see the 15 instance types passed to the Amazon EC2 Fleet API:
apiVersion: karpenter.sh/v1beta1
kind: NodeClaim
...
spec:
nodeClassRef:
name: default
requirements:
...
- key: node.kubernetes.io/instance-type
operator: In
values:
- c5.large
- c5ad.large
- c6g.large
- c6gn.large
- c6i.large
- c6id.large
- c7a.large
- c7g.large
- c7gd.large
- m6a.large
- m6g.large
- m6gd.large
- m7g.large
- m7i-flex.large
- r6g.large
...
What would happen if a Spot node could not be consolidated?
If a Spot node cannot be consolidated because there are not 15 instance types in the compute selection, then the following message will appear in the events for the NodeClaim object. You might get this event if you overly constrained your instance type selection:
Normal Unconsolidatable 31s karpenter SpotToSpotConsolidation requires 15 cheaper instance type options than the current candidate to consolidate, got 1
Spot best practices with Karpenter
The following are some best practices to consider when using Spot Instances with Karpenter.
- Avoid overly constraining instance type selection: Karpenter selects Spot Instances using the price-capacity-optimized allocation strategy, which balances the price and availability of AWS spare capacity. Although a minimum of 15 instances are needed, you should avoid constraining instance types as much as possible. By not constraining instance types, there is a higher chance of acquiring Spot capacity at large scales with a lower frequency of Spot Instance interruptions at a lower cost.
- Gracefully handle Spot interruptions and consolidation actions: Karpenter natively handles Spot interruption notifications by consuming events from an Amazon Simple Queue Service (Amazon SQS) queue, which is populated with Spot interruption notifications through Amazon EventBridge. As soon as Karpenter receives a Spot interruption notification, it gracefully drains the interrupted node of any running pods while also provisioning a new node for which those pods can schedule. With Spot Instances, this process needs to complete within 2 minutes. For a pod with a termination period longer than 2 minutes, the old node will be interrupted prior to those pods being rescheduled. To test a replacement node, AWS Fault Injection Service (FIS) can be used to simulate Spot interruptions.
- Carefully configure resource requests and limits for workloads: Rightsizing and optimizing your cluster is a shared responsibility. Karpenter effectively optimizes and scales infrastructure, but the end result depends on how well you have rightsized your pod requests and any other Kubernetes scheduling constraints. Karpenter does not consider limits or resource utilization. For most workloads with non-compressible resources, such as memory, it is generally recommended to set requests==limits because if a workload tries to burst beyond the available memory of the host, an out-of-memory (OOM) error occurs. Karpenter consolidation can increase the probability of this as it proactively tries to reduce total allocatable resources for a Kubernetes cluster. For help with rightsizing your Kubernetes pods, consider exploring Kubecost, Vertical Pod Autoscaler configured in recommendation mode, or an open source tool such as Goldilocks.
- Configure metrics for Karpenter: Karpenter emits metrics in the Prometheus format, so consider using Amazon Managed Service for Prometheus to track interruptions caused by Karpenter Drift, consolidation, Spot interruptions, or other Amazon EC2 maintenance events. These metrics can be used to confirm that interruptions are not having a significant impact on your service’s availability and monitor NodePool usage and pod lifecycles. The Karpenter Getting Started Guide contains an example Grafana dashboard configuration.
You can learn more about other application best practices in the Reliability section of the Amazon EKS Best Practices Guide.
Cleanup
To avoid incurring future charges, delete any resources you created as part of this walkthrough. If you followed the Karpenter Getting Started Guide to set up a cluster and add Karpenter, follow the clean-up instructions in the Karpenter documentation to delete the cluster. Alternatively, if you already had a cluster with Karpenter, delete the resources created as part of this walkthrough:
kubectl delete -f inflate.yaml
kubectl delete -f nodepool.yaml
Conclusion
In this post, you learned how Karpenter can actively replace a Spot node with another more cost-efficient Spot node. Karpenter can consolidate Spot nodes that have the right balance between lower price and low-frequency interruptions when there are at least 15 selectable instances to balance price and availability.
To get started, check out the Karpenter documentation as well as Karpenter Blueprints, which is a repository including common workload scenarios following the best practices.
You can share your feedback on this feature by a raising a GitHub Issue.