Post Syndicated from Grab Tech original https://engineering.grab.com/attribution-platform
The Grab superapp offers a comprehensive array of services from ride-hailing and food delivery to financial services. This creates multifaceted user journeys, traversing homepages, product pages, checkouts, and interactions with diverse content, including advertisements and promo codes.
Background: Why ads and attribution matter in our superapp
Ads are crucial for Grab in driving user engagement and supporting our ecosystem by seamlessly connecting users with our services. In the ever-evolving world of advertising, the ability to gauge the impact of marketing investments takes on pivotal significance. Advertisers dedicate substantial resources to promote their businesses, necessitating a clear understanding of the return on AdSpend (ROAS) for each campaign. In this context, attribution plays a central role, serving as the guiding compass for advertisers and marketers, elucidating the effectiveness of touchpoints within campaigns.
For instance, a merchant-partner seeks to enhance its reach by advertising on the Grab food delivery homepage. With the assistance of our attribution system, the merchant-partner can now precisely gauge the impact of their homepage ads on Grab. This involves tracking user engagement and monitoring the resulting orders that stem from these interactions. This level of granularity not only highlights the value of attribution but also demonstrates its capability in providing detailed insights into the effectiveness of advertising campaigns and enabling merchant-partners to optimise their campaigns with more precision.
In this blog, we delve into the technical intricacies, software architecture, challenges, and solutions involved in crafting a state-of-the-art engineering solution for the attribution platform.
Genesis: Pre-project landscape
When our journey began in 2020, Grab’s marketing efforts had limited attribution capabilities and data analytics was predominantly reliant on ad hoc queries conducted by business and data analysts. Before the introduction of a standardised approach, we had to manage discrepant results and a time-consuming manual process of data preparation, cleansing, and storage across teams. When issues arose in the analytical pipeline, resolution efforts took relatively longer and were reoccurring. We needed a comprehensive engineering solution that would address the identified gaps, and significantly enhance metrics related to ROI, attribution accuracy, and data-handling efficiency.
Inception: The pure ads attribution engine (Kappa architecture)
We chose Kappa architecture due to its imperative role in achieving near real-time attribution, especially in support of our new pricing model, cost per order (CPO). With this solution, we aimed to drastically reduce data latency from 2-3 days to just a few minutes. Traditional ETL (Extract, Transform, and Load) based batch processing methods were evaluated but quickly found to be inadequate for our purposes, mainly due to their speed.
In the advertising industry, rapid decision-making is critical. Traditional batch processing solutions would introduce significant latency, hampering our ability to make real-time, data-driven decisions. With its architecture’s inherent capability for real-time stream processing, Kappa emerged as the logical choice. Additionally, Kappa offers the agility required to empower our ad-serving team for real-time decision support, and better ad ranking and selection, enabling dynamic and effective targeting decisions without delay.
The first step on this journey was to create a pure and near real-time stream processing Ads Attribution Engine. This engine was based on the Kappa architecture to provide advertisers with quick insights into their ROAS offering real-time attribution, enabling advertisers to optimise their campaigns efficiently.
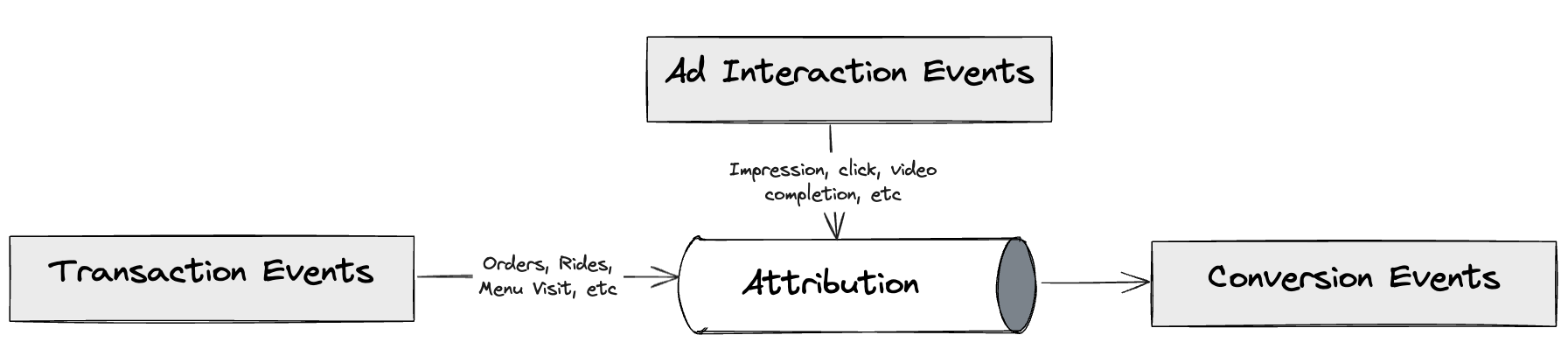
In this solution, we used the following tools in our tech stack:
- Kafka for event streams
- DDB for events storage
- Amazon S3 as the data lake
- An in-house stream processing framework similar to Keystone
- Redis for caching events
- ScyllaDB for storing ad metadata
- Amazon relational database service (RDS) for analytics
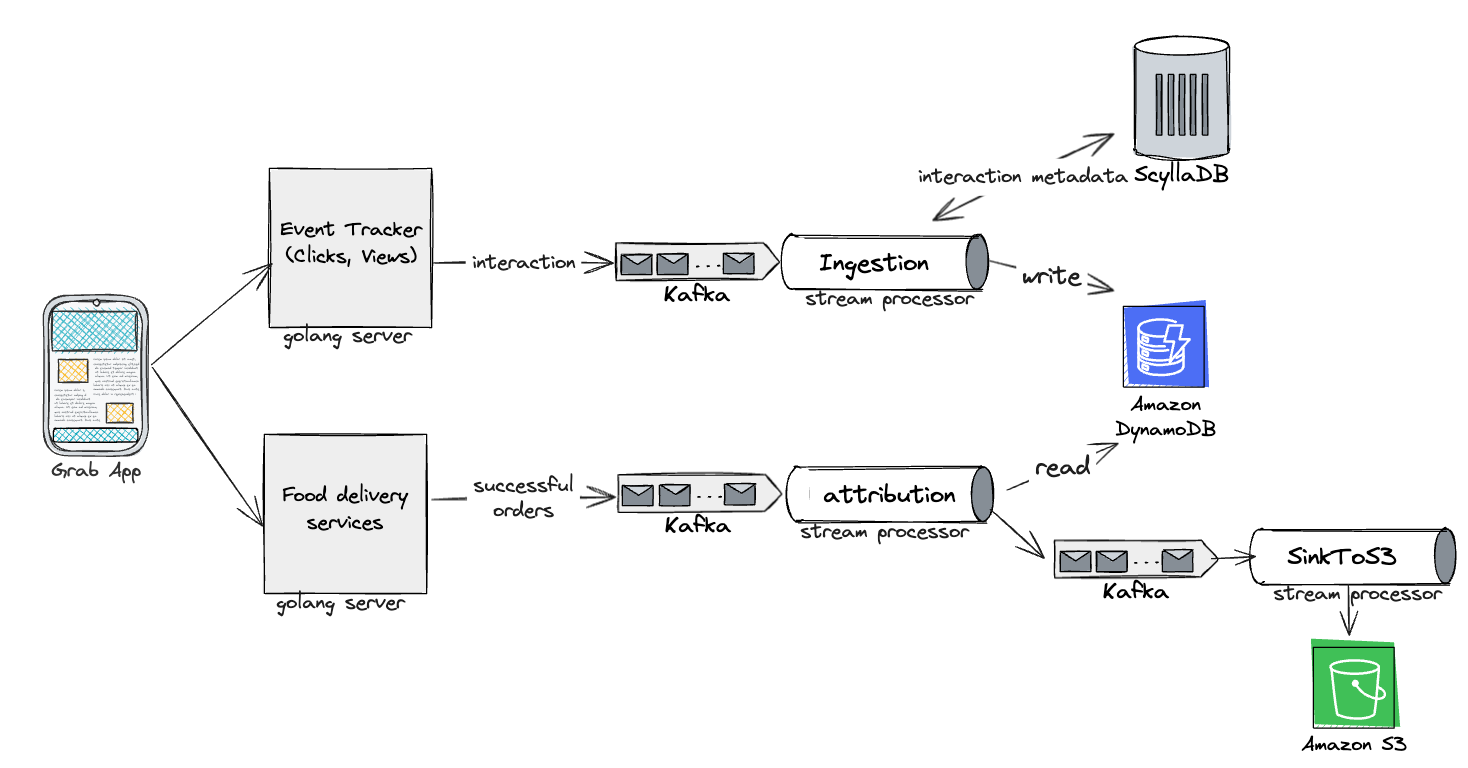
Evolution: Merging marketing levers – Ads and promos
We began to envision a world where we could merge various marketing levers into a unified Attribution Engine, starting with ads and promos. This evolved vision also aimed to prevent order double counting (when a user interacts with both ads and promos in the same checkout), which would provide a more holistic attribution solution.
With the unified Attribution Engine, we would also enable more sophisticated personalisation through machine learning models and drive higher conversions.
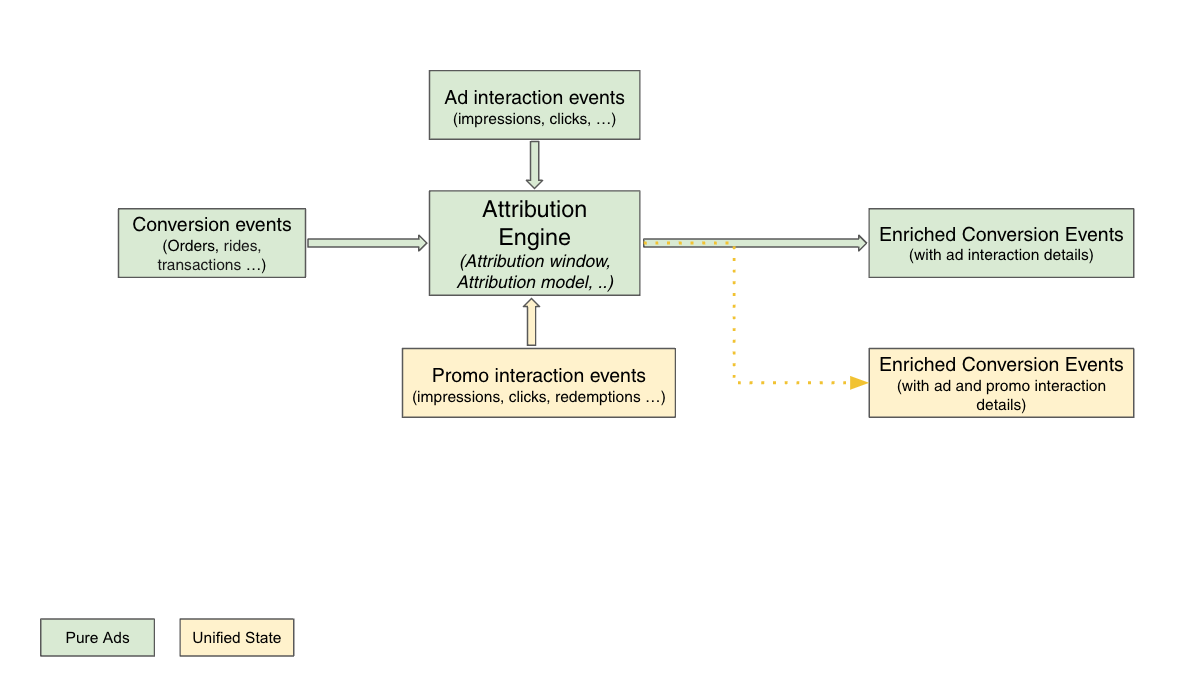
The unified attribution engine used mostly the same tech stack, except for analytics where Druid was used instead of RDS.
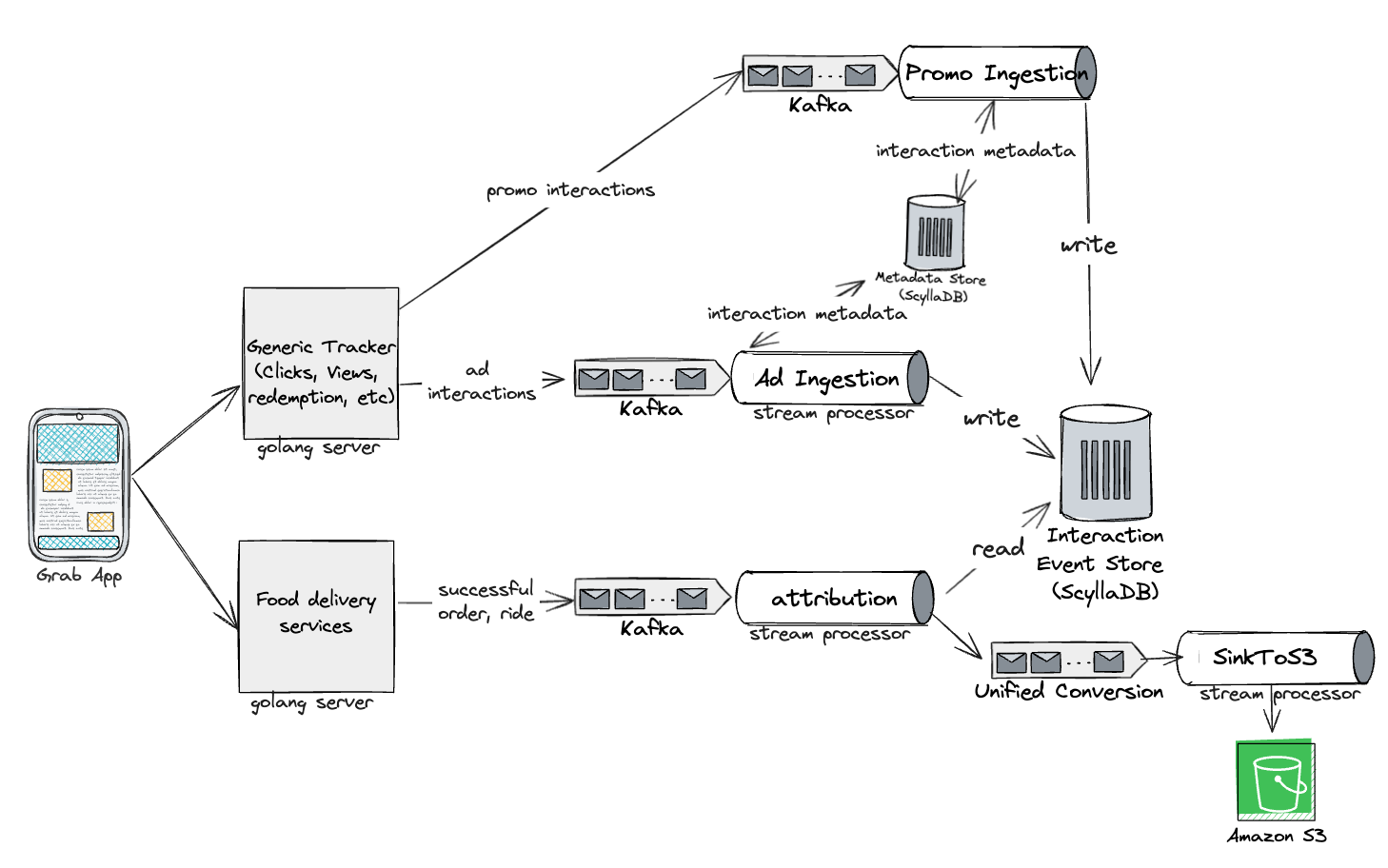
Introspection: Identifying shortcomings and the path to improvement
While the unified attribution engine was a step in the right direction, it wasn’t without its challenges. There were challenges related to real-time data processing costs, scalability for longer attribution windows, latency and lag issues, out-of-order events leading to misattribution, and the complexity of implementing multi-touch attribution models. To truly empower advertisers and enhance the attribution process, we knew we needed to evolve further.
Rebirth: The birth of a full-fledged attribution platform (Lambda architecture)
This journey eventually led us to build a full-fledged attribution platform using Lambda architecture, which blended both batch and real-time stream processing methods. With this change, our platform could rapidly and accurately process data and attribute the impact of ads and promos on user behaviour.
Why Lambda architecture?
This choice was a strategic one – real-time processing is vital for tracking events as they occur, but it offers only a current snapshot of user behaviour. This means we would not be able to analyse historical data, which is a crucial aspect of accurate attribution and exploring multiple attribution models. Historical data allows us to identify trends, patterns, and correlations not evident in real-time data alone.
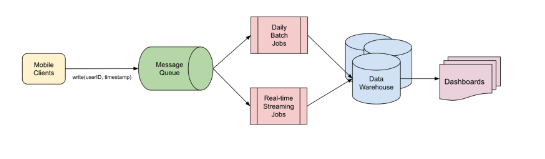
In this system’s tech stack, the key components are:
- Coban, an in-house stream processing framework used for real-time data processing
- Spark-based ETL jobs for batch processing
- Amazon S3 as the data warehouse
- An offline layer that is capable of providing historical context, handling large data volumes, performing complex analytics, and so on.
Key benefits of the offline layer
- Provides historical context: The offline layer enriches the attribution process by providing a historical perspective on user interactions, essential for precise attribution analysis spanning extended time periods.
- Handles enormous data volumes: This layer efficiently manages and processes extensive data generated by advertising campaigns, ensuring that attribution seamlessly accommodates large-scale data sets.
- Performs complex analytics: Enables more intricate computations and data analysis than real-time processing alone, the offline layer is instrumental in fine-tuning attribution models and enhancing their accuracy.
- Ensures reliability in the face of challenges: By providing fault tolerance and resilience against system failures, the offline layer ensures the continuous and dependable operation of the attribution system, even during unexpected events.
- Optimises data storage and serving: Relying on Amazon S3, the storage layer for raw data optimises storage by building interactive reporting APIs.
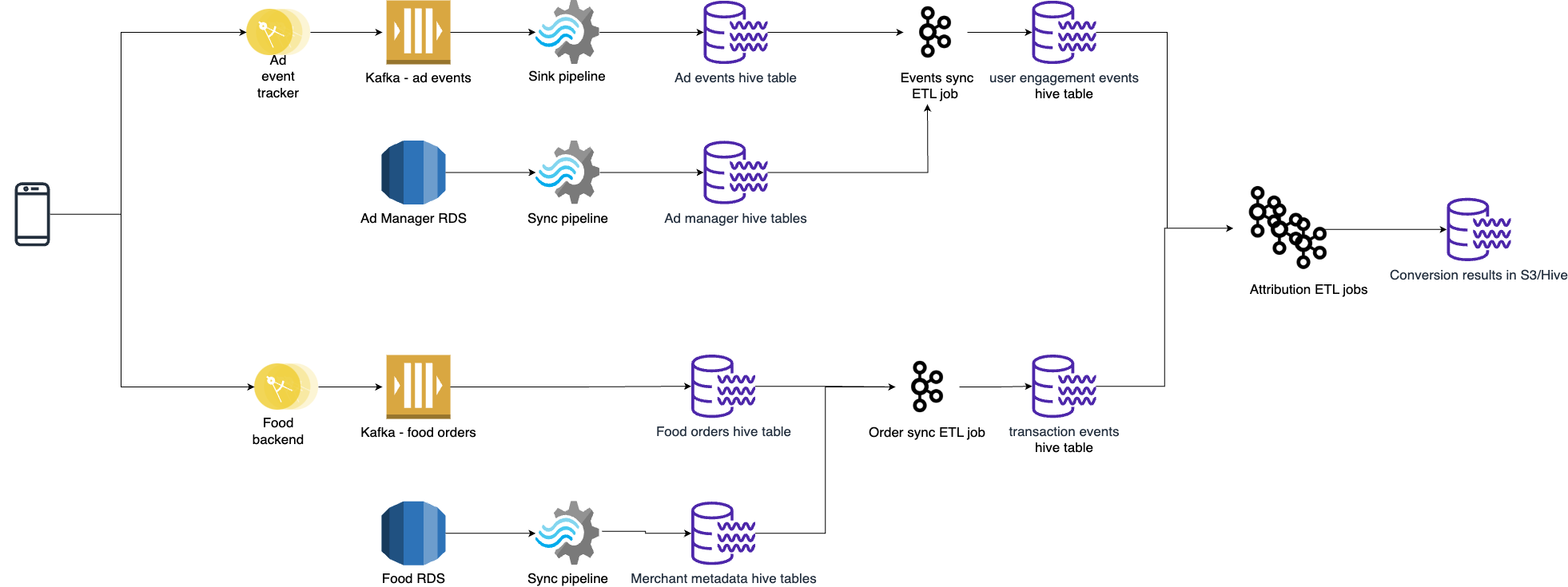
Challenges with Lambda and mitigation
Lambda architecture allows us to have the accuracy and robustness of batch processing along with real-time stream processing. However, we noticed some drawbacks that may lead to complexity due to maintaining both batch and stream processing:
- Operating two parallel systems for batch and stream processing can lead to increased complexity in production environments.
- Lambda architecture requires two sets of business logic – one for the batch layer and another for the stream layer.
- Synchronisation across both layers can make system alterations more challenging.
- This dual implementation could also allude to inconsistencies and introduce potential bugs into the system.
To mitigate these complications, we’re establishing an optimisation strategy for our current system. By distinctly separating the responsibilities of our real-time pipelines from those of our offline jobs, we intend to harness the full potential of each approach, while simultaneously curbing the added complexity.
Hence, redefining the way we utilise Lambda architecture, striking an efficient balance between real-time responsiveness and sturdy accuracy with the below proposal.
Vanguard: Enhancements in the future
In the coming months, we will be implementing the optimisation strategy and improving our attribution platform solution. This strategy can be broken down into the following sections.
Real-time pipeline handling time-sensitive data: Real-time pipelines can process and deliver time-sensitive metrics like CPO-related data in near real-time, allowing for budget capping and immediate adjustments to marketing spend. This can provide us with actionable insights that can help with areas like real-time bidding, real-time marketing, or dynamic pricing. By limiting the volume of data through the real-time path, we can ensure it’s more manageable and focused on immediate actionable data.
Batch jobs handling all other reporting data: Batch processing is best suited for computations that are not time-bound and where completeness is more important. By dedicating more time to the processing phase, batch processing can handle larger volumes and more complex computations, providing more comprehensive and accurate reporting.
This approach will simplify our Lambda architecture, as the batch and real-time pipelines will have clear separation of duties. It may also reduce the chance of discrepancies between the real-time and batch-processing datasets and lower the operational load of our real-time system.
Conclusion: A holistic attribution picture
Through our journey of building a comprehensive attribution platform, we can now deliver a holistic and dependable view of user behaviour and empower merchant-partners to use insights from advertisements and promotions. This journey has been a long one, but we were able to improve our attribution solution in several ways:
- Attribution latency: Successfully reduced attribution latency from 2-3 days to just a few minutes, ensuring that advertisers can access real-time insights and feedback.
- Data accuracy: Through improved data collection and processing, we achieved data discrepancies of less than 1%, enhancing the accuracy and reliability of attribution data.
- Conversion rate: Advertisers witnessed a significant increase in conversion rates, a direct result of our real-time attribution capabilities.
- Cost efficiency: Embracing the Lambda architecture led to a ~25% reduction in real-time data processing costs, allowing for more efficient campaign optimisations.
- Operational resilience: Building an offline layer provided fault tolerance and resilience against system failures, ensuring that our attribution system continued to operate seamlessly, even during unexpected events.
Join us
Grab is the leading superapp platform in Southeast Asia, providing everyday services that matter to consumers. More than just a ride-hailing and food delivery app, Grab offers a wide range of on-demand services in the region, including mobility, food, package and grocery delivery services, mobile payments, and financial services across 428 cities in eight countries.
Powered by technology and driven by heart, our mission is to drive Southeast Asia forward by creating economic empowerment for everyone. If this mission speaks to you, join our team today!