Post Syndicated from Vy Ton original https://blog.cloudflare.com/building-d1-a-global-database
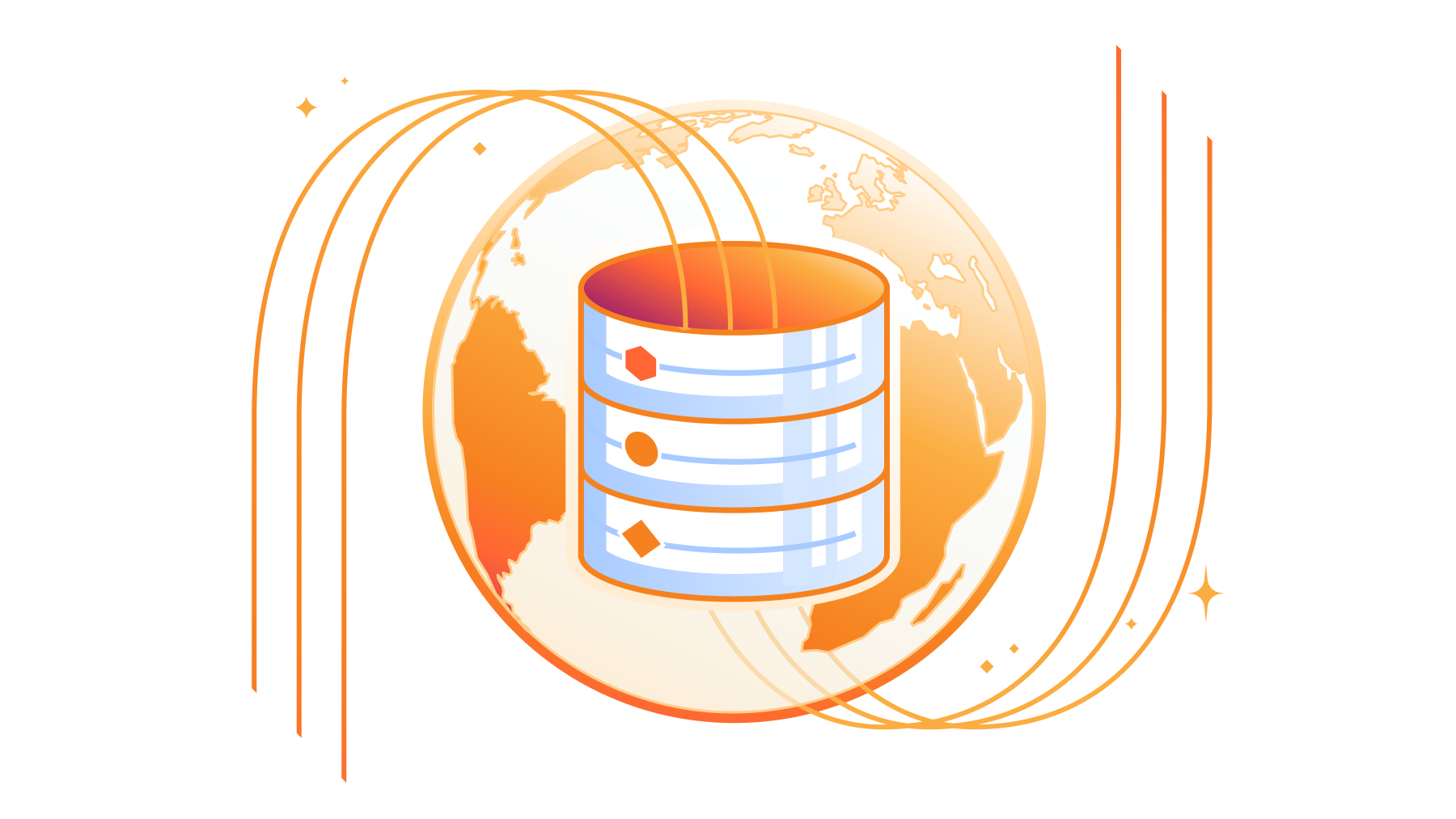
Developers who build Worker applications focus on what they’re creating, not the infrastructure required, and benefit from the global reach of Cloudflare’s network. Many applications require persistent data, from personal projects to business-critical workloads. Workers offer various database and storage options tailored to developer needs, such as key-value and object storage.
Relational databases are the backbone of many applications today. D1, Cloudflare’s relational database complement, is now generally available. Our journey from alpha in late 2022 to GA in April 2024 focused on enabling developers to build production workloads with the familiarity of relational data and SQL.
What’s D1?
D1 is Cloudflare’s built-in, serverless relational database. For Worker applications, D1 offers SQL’s expressiveness, leveraging SQLite’s SQL dialect, and developer tooling integrations, including object-relational mappers (ORMs) like Drizzle ORM. D1 is accessible via Workers or an HTTP API.
Serverless means no provisioning, default disaster recovery with Time Travel, and usage-based pricing. D1 includes a generous free tier that allows developers to experiment with D1 and then graduate those trials to production.
How to make data global?
D1 GA has focused on reliability and developer experience. Now, we plan on extending D1 to better support globally-distributed applications.
In the Workers model, an incoming request invokes serverless execution in the closest data center. A Worker application can scale globally with user requests. Application data, however, remains stored in centralized databases, and global user traffic must account for access round trips to data locations. For example, a D1 database today resides in a single location.
Workers support Smart Placement to account for frequently accessed data locality. Smart Placement invokes a Worker closer to centralized backend services like databases to lower latency and improve application performance. We’ve addressed Workers placement in global applications, but need to solve data placement.
The question, then, is how can D1, as Cloudflare’s built-in database solution, better support data placement for global applications? The answer is asynchronous read replication.
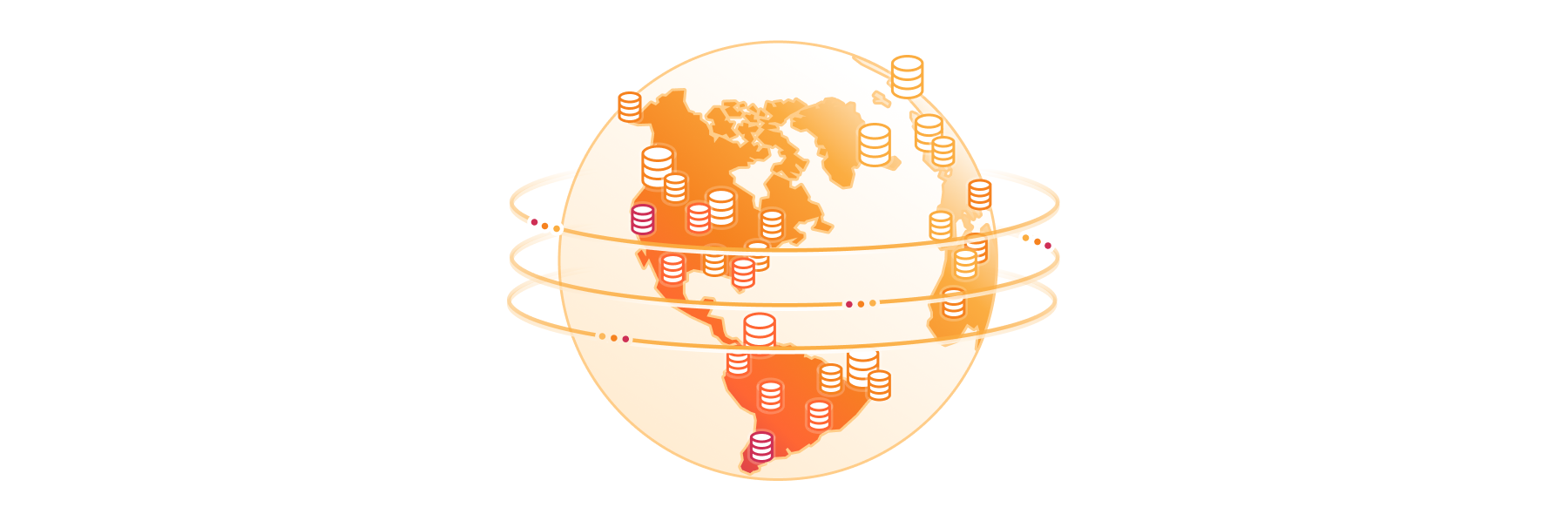
What is asynchronous read replication?
In a server-based database management system, like Postgres, MySQL, SQL Server, or Oracle, a read replica is a separate database server that serves as a read-only, almost up-to-date copy of the primary database server. An administrator creates a read replica by starting a new server from a snapshot of the primary server and configuring the primary server to send updates asynchronously to the replica server. Since the updates are asynchronous, the read replica may be behind the current state of the primary server. The difference between the primary server and a replica is called replica lag. It’s possible to have more than one read replica.
Asynchronous read replication is a time-proven solution for improving the performance of databases:
- It’s possible to increase throughput by distributing load across multiple replicas.
- It’s possible to lower query latency when the replicas are close to the users making queries.
Note that some database systems also offer synchronous replication. In a synchronous replicated system, writes must wait until all replicas have confirmed the write. Synchronous replicated systems can run only as fast as the slowest replica and come to a halt when a replica fails. If we’re trying to improve performance on a global scale, we want to avoid synchronous replication as much as possible!
Consistency models & read replicas
Most database systems provide read committed, snapshot isolation, or serializable consistency models, depending on their configuration. For example, Postgres defaults to read committed but can be configured to use stronger modes. SQLite provides snapshot isolation in WAL mode. Stronger modes like snapshot isolation or serializable are easier to program against because they limit the permitted system concurrency scenarios and the kind of concurrency race conditions the programmer has to worry about.
Read replicas are updated independently, so each replica’s contents may differ at any moment. If all of your queries go to the same server, whether the primary or a read replica, your results should be consistent according to whatever consistency model your underlying database provides. If you’re using a read replica, the results may just be a little old.
In a server-based database with read replicas, it’s important to stick with the same server for all of the queries in a session. If you switch among different read replicas in the same session, you compromise the consistency model provided by your application, which may violate your assumptions about how the database acts and cause your application to return incorrect results!
Example
For example, there are two replicas, A and B. Replica A lags the primary database by 100ms, and replica B lags the primary database by 2s. Suppose a user wishes to:
- Execute query 1
1a. Do some computation based on query 1 results - Execute query 2 based on the results of the computation in (1a)
At time t=10s, query 1 goes to replica A and returns. Query 1 sees what the primary database looked like at t=9.9s. Suppose it takes 500ms to do the computation, so at t=10.5s, query 2 goes to replica B. Remember, replica B lags the primary database by 2s, so at t=10.5s, query 2 sees what the database looks like at t=8.5s. As far as the application is concerned, the results of query 2 look like the database has gone backwards in time!
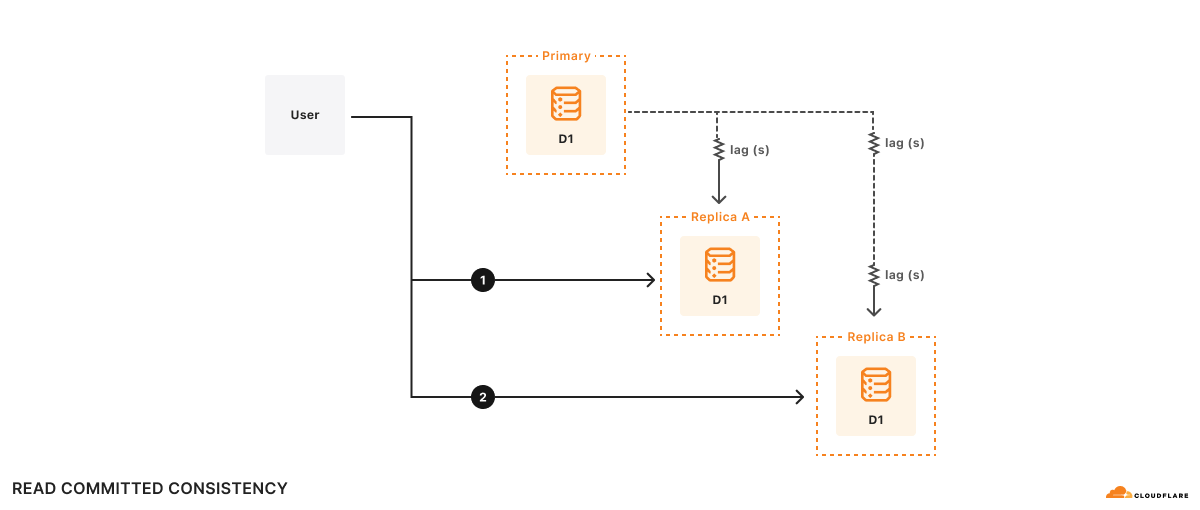
Formally, this is read committed consistency since your queries will only see committed data, but there’s no other guarantee – not even that you can read your own writes. While read committed is a valid consistency model, it’s hard to reason about all of the possible race conditions the read committed model allows, making it difficult to write applications correctly.
D1’s consistency model & read replicas
By default, D1 provides the snapshot isolation that SQLite provides.
Snapshot isolation is a familiar consistency model that most developers find easy to use. We implement this consistency model in D1 by ensuring at most one active copy of the D1 database and routing all HTTP requests to that single database. While ensuring that there’s at most one active copy of the D1 database is a gnarly distributed systems problem, it’s one that we’ve solved by building D1 using Durable Objects. Durable Objects guarantee global uniqueness, so once we depend on Durable Objects, routing HTTP requests is easy: just send them to the D1 Durable Object.
This trick doesn’t work if you have multiple active copies of the database since there’s no 100% reliable way to look at a generic incoming HTTP request and route it to the same replica 100% of the time. Unfortunately, as we saw in the previous section’s example, if we don’t route related requests to the same replica 100% of the time, the best consistency model we can provide is read committed.
Given that it’s impossible to route to a particular replica consistently, another approach is to route requests to any replica and ensure that the chosen replica responds to requests according to a consistency model that “makes sense” to the programmer. If we’re willing to include a Lamport timestamp in our requests, we can implement sequential consistency using any replica. The sequential consistency model has important properties like “read my own writes” and “writes follow reads,” as well as a total ordering of writes. The total ordering of writes means that every replica will see transactions commit in the same order, which is exactly the behavior we want in a transactional system. Sequential consistency comes with the caveat that any individual entity in the system may be arbitrarily out of date, but that caveat is a feature for us because it allows us to consider replica lag when designing our APIs.
The idea is that if D1 gives applications a Lamport timestamp for every database query and those applications tell D1 the last Lamport timestamp they’ve seen, we can have each replica determine how to make queries work according to the sequential consistency model.
A robust, yet simple, way to implement sequential consistency with replicas is to:
- Associate a Lamport timestamp with every single request to the database. A monotonically increasing commit token works well for this.
- Send all write queries to the primary database to ensure the total ordering of writes.
- Send read queries to any replica, but have the replica delay servicing the query until the replica receives updates from the primary database that are later than the Lamport timestamp in the query.
What’s nice about this implementation is that it’s fast in the common case where a read-heavy workload always goes to the same replica and will work even if requests get routed to different replicas.
Sneak Preview: bringing read replication to D1 with Sessions
To bring read replication to D1, we will expand the D1 API with a new concept: Sessions. A Session encapsulates all the queries representing one logical session for your application. For example, a Session might represent all requests coming from a particular web browser or all requests coming from a mobile app. If you use Sessions, your queries will use whatever copy of the D1 database makes the most sense for your request, be that the primary database or a nearby replica. D1’s Sessions implementation will ensure sequential consistency for all queries in the Session.
Since the Sessions API changes D1’s consistency model, developers must opt-in to the new API. Existing D1 API methods are unchanged and will still have the same snapshot isolation consistency model as before. However, only queries made using the new Sessions API will use replicas.
Here’s an example of the D1 Sessions API:
export default {
async fetch(request: Request, env: Env) {
// When we create a D1 Session, we can continue where we left off
// from a previous Session if we have that Session's last commit
// token. This Worker will return the commit token back to the
// browser, so that it can send it back on the next request to
// continue the Session.
//
// If we don't have a commit token, make the first query in this
// session an "unconditional" query that will use the state of the
// database at whatever replica we land on.
const token = request.headers.get('x-d1-token') ?? 'first-unconditional'
const session = env.DB.withSession(token)
// Use this Session for all our Workers' routes.
const response = await handleRequest(request, session)
if (response.status === 200) {
// Set the token so we can continue the Session in another request.
response.headers.set('x-d1-token', session.latestCommitToken)
}
return response
}
}
async function handleRequest(request: Request, session: D1DatabaseSession) {
const { pathname } = new URL(request.url)
if (pathname === '/api/orders/list') {
// This statement is a read query, so it will execute on any
// replica that has a commit equal or later than `token` we used
// to create the Session.
const { results } = await session.prepare('SELECT * FROM Orders').all()
return Response.json(results)
} else if (pathname === '/api/orders/add') {
const order = await request.json<Order>()
// This statement is a write query, so D1 will send the query to
// the primary, which always has the latest commit token.
await session
.prepare('INSERT INTO Orders VALUES (?, ?, ?)')
.bind(order.orderName, order.customer, order.value)
.run()
// In order for the application to be correct, this SELECT
// statement must see the results of the INSERT statement above.
// The Session API keeps track of commit tokens for queries
// within the session and will ensure that we won't execute this
// query until whatever replica we're using has seen the results
// of the INSERT.
const { results } = await session
.prepare('SELECT COUNT(*) FROM Orders')
.all()
return Response.json(results)
}
return new Response('Not found', { status: 404 })
}
D1’s implementation of Sessions makes use of commit tokens. Commit tokens identify a particular committed query to the database. Within a session, D1 will use commit tokens to ensure that queries are sequentially ordered. In the example above, the D1 session ensures that the “SELECT COUNT(*)” query happens after the “INSERT” of the new order, even if we switch replicas between the awaits.
There are several options on how you want to start a session in a Workers fetch handler. db.withSession(<condition>)
accepts these arguments:
|
Behavior |
|
(1) starts Session as of given commit token (2) subsequent queries have sequential consistency |
|
(1) if the first query is read, read whatever current replica has and use the commit token of that read as the basis for subsequent queries. If the first query is a write, forward the query to the primary and use the commit token of the write as the basis for subsequent queries. (2) subsequent queries have sequential consistency |
|
(1) runs first query, read or write, against the primary (2) subsequent queries have sequential consistency |
|
treated like |
It’s possible to have a session span multiple requests by “round-tripping” the commit token from the last query of the session and using it to start a new session. This enables individual user agents, like a web app or a mobile app, to make sure that all of the queries the user sees are sequentially consistent.
D1’s read replication will be built-in, will not incur extra usage or storage costs, and will require no replica configuration. Cloudflare will monitor an application’s D1 traffic and automatically create database replicas to spread user traffic across multiple servers in locations closer to users. Aligned with our serverless model, D1 developers shouldn’t worry about replica provisioning and management. Instead, developers should focus on designing applications for replication and data consistency tradeoffs.
We’re actively working on global read replication and realizing the above proposal (share feedback In the #d1 channel on our Developer Discord). Until then, D1 GA includes several exciting new additions.
Check out D1 GA
Since D1’s open beta in October 2023, we’ve focused on D1’s reliability, scalability, and developer experience demanded of critical services. We’ve invested in several new features that allow developers to build and debug applications faster with D1.
Build bigger with larger databases
We’ve listened to developers who requested larger databases. D1 now supports up to 10GB databases, with 50K databases on the Workers Paid plan. With D1’s horizontal scaleout, applications can model database-per-business-entity use cases. Since beta, new D1 databases process 40x more requests than D1 alpha databases in a given period.
Import & export bulk data
Developers import and export data for multiple reasons:
- Database migration testing to/from different database systems
- Data copies for local development or testing
- Manual backups for custom requirements like compliance
While you could execute SQL files against D1 before, we’re improving wrangler d1 execute –file=<filename>
to ensure large imports are atomic operations, never leaving your database in a halfway state. wrangler d1 execute
also now defaults to local-first to protect your remote production database.
To import our Northwind Traders demo database, you can download the schema & data and execute the SQL files.
npx wrangler d1 create northwind-traders
# omit --remote to run on a local database for development
npx wrangler d1 execute northwind-traders --remote --file=./schema.sql
npx wrangler d1 execute northwind-traders --remote --file=./data.sql
D1 database data & schema, schema-only, or data-only can be exported to a SQL file using:
# database schema & data
npx wrangler d1 export northwind-traders --remote --output=./database.sql
# single table schema & data
npx wrangler d1 export northwind-traders --remote --table='Employee' --output=./table.sql
# database schema only
npx wrangler d1 export <database_name> --remote --output=./database-schema.sql --no-data=true
Debug query performance
Understanding SQL query performance and debugging slow queries is a crucial step for production workloads. We’ve added the experimental wrangler d1 insights
to help developers analyze query performance metrics also available via GraphQL API.
# To find top 10 queries by average execution time:
npx wrangler d1 insights <database_name> --sort-type=avg --sort-by=time --count=10
Developer tooling
Various community developer projects support D1. New additions include Prisma ORM, in version 5.12.0, which now supports Workers and D1.
Next steps
The features available now with GA and our global read replication design are just the start of delivering the SQL database needs for developer applications. If you haven’t yet used D1, you can get started right now, visit D1’s developer documentation to spark some ideas, or join the #d1 channel on our Developer Discord to talk to other D1 developers and our product engineering team.
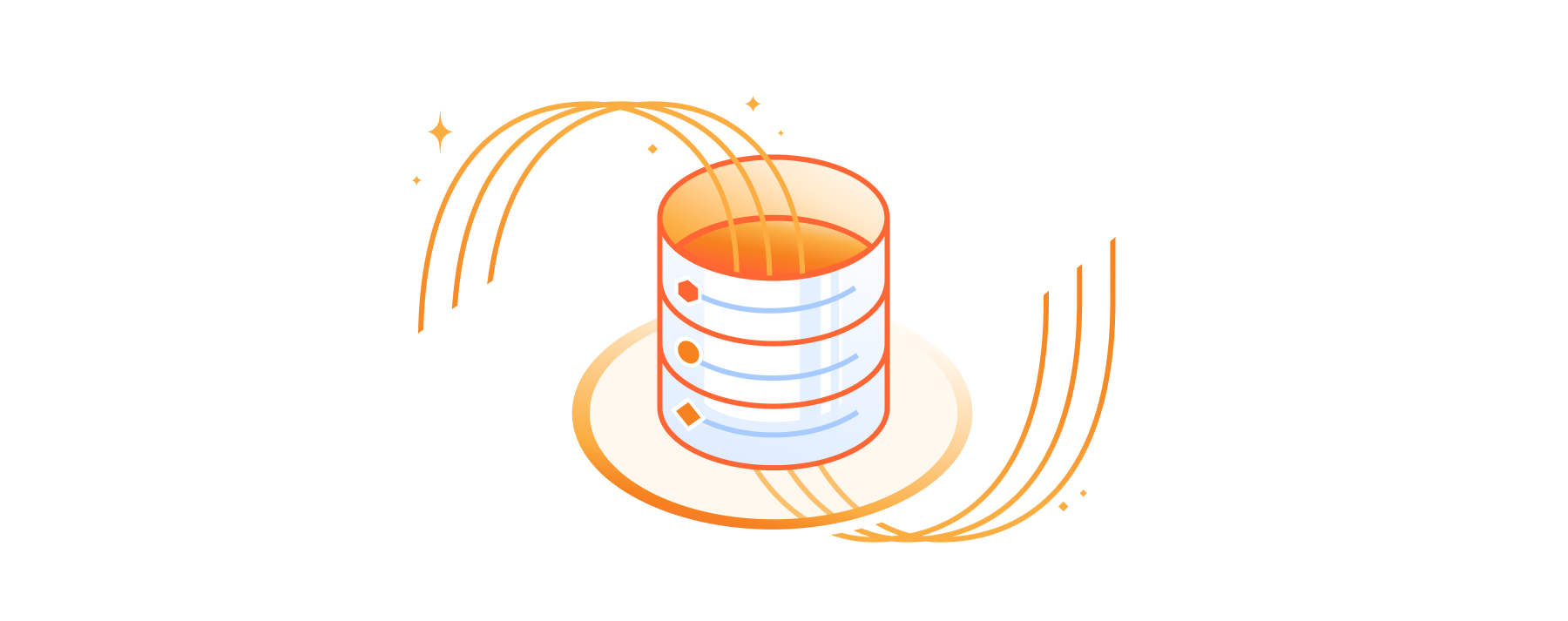